nature.com/articles/s4146…
We use evolutionary algorithms, simulations and unsupervised machine learning clusterization to study the dynamics of CA1 pyramidal cells during theta. See the thread! 👇🏽
nature.com/articles/nn.40…
We have seen that during theta, they tend to segregate their firing and shift on demand
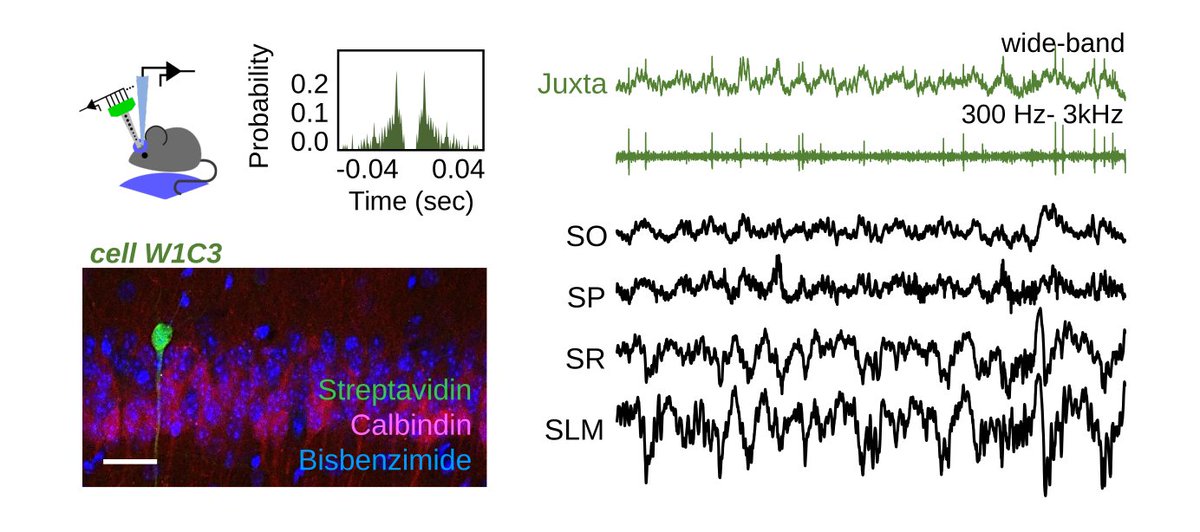
Check the model here!
> github.com/acnavasolive/L…
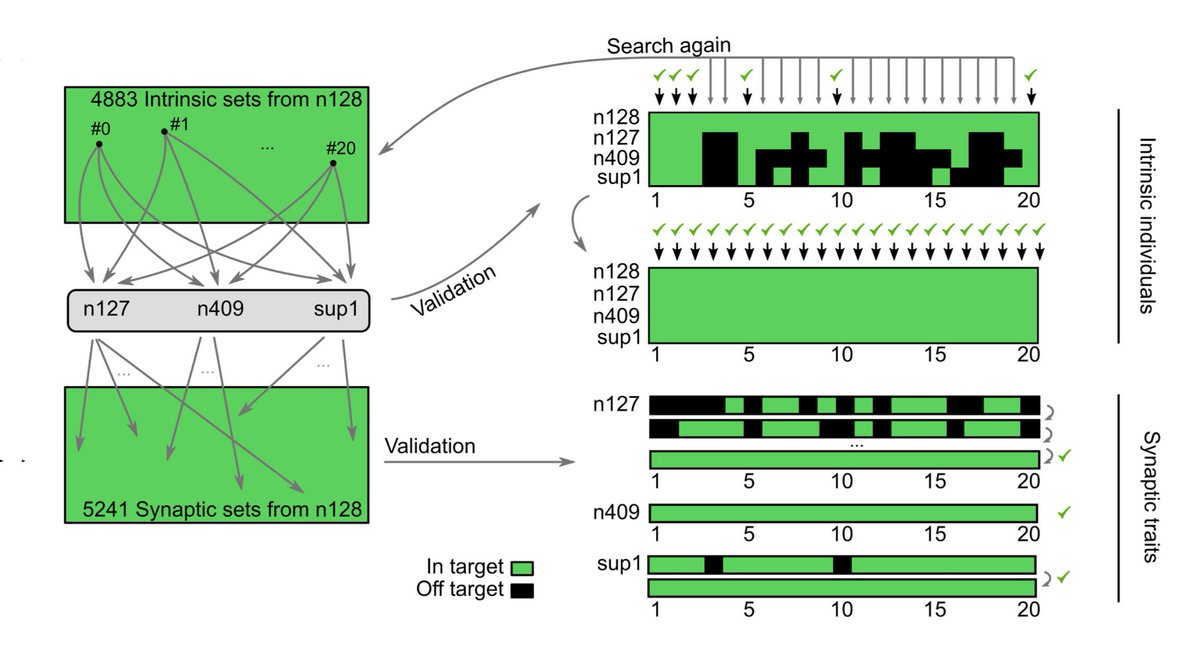
See our #Matlab tool RhythSOM:
github.com/acnavasolive/R…
Thanks @EnrRodSeb! :D
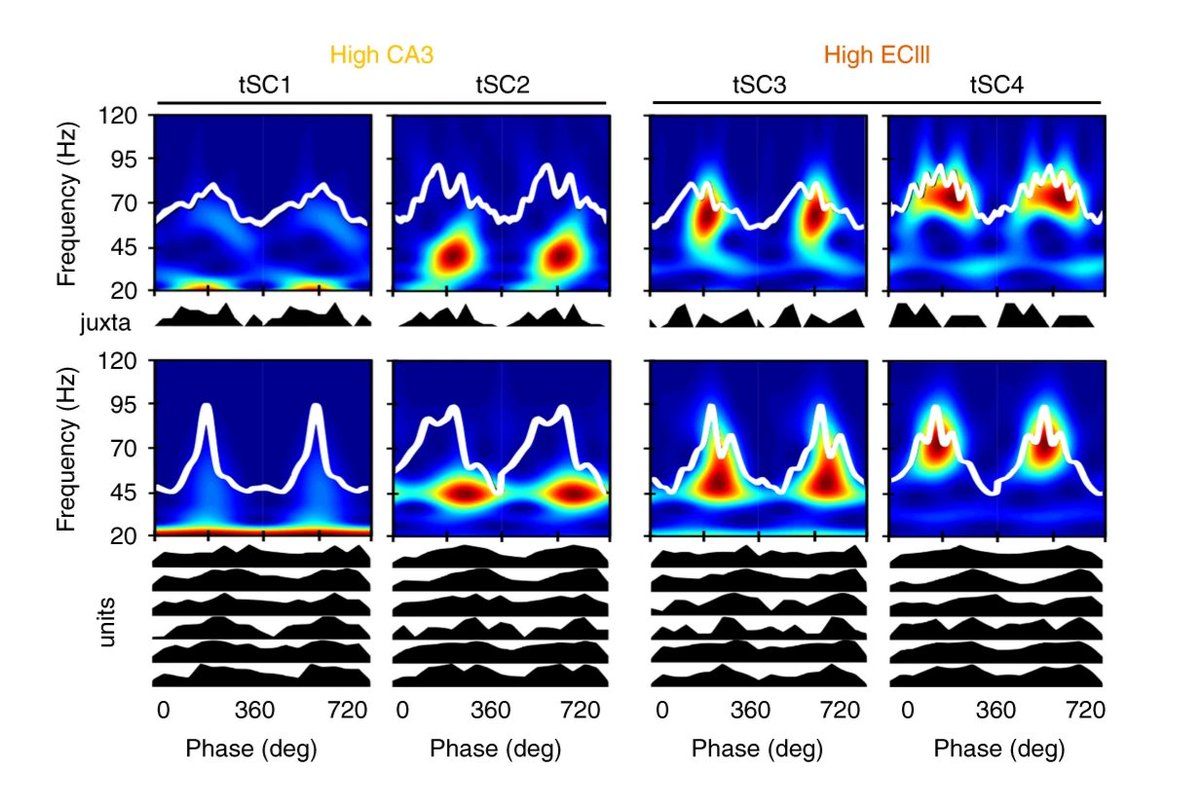