w/ @adityagrover_ @_smileyball Trisha Singh @StefanoErmon
arxiv.org/abs/1910.12008
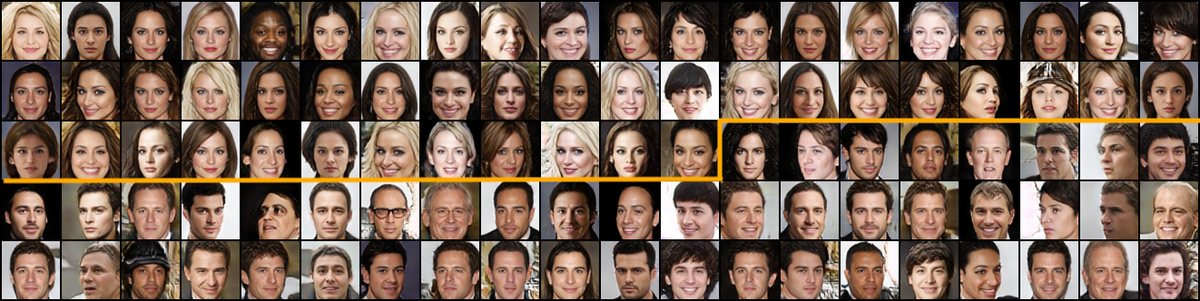
We hope our work is a first step towards solutions that take a more holistic view of bias and fairness in generative modeling, esp. in light of everything going on lately.