arxiv.org/abs/1806.07555
arxiv.org/abs/1705.00253
2/N
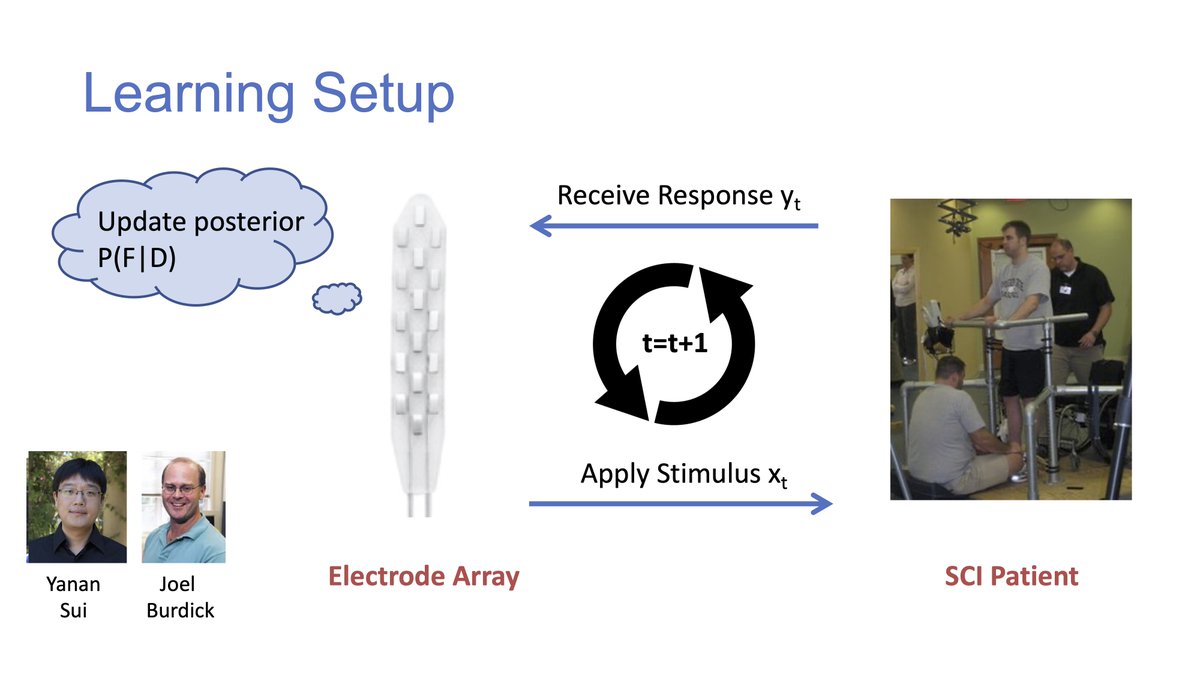
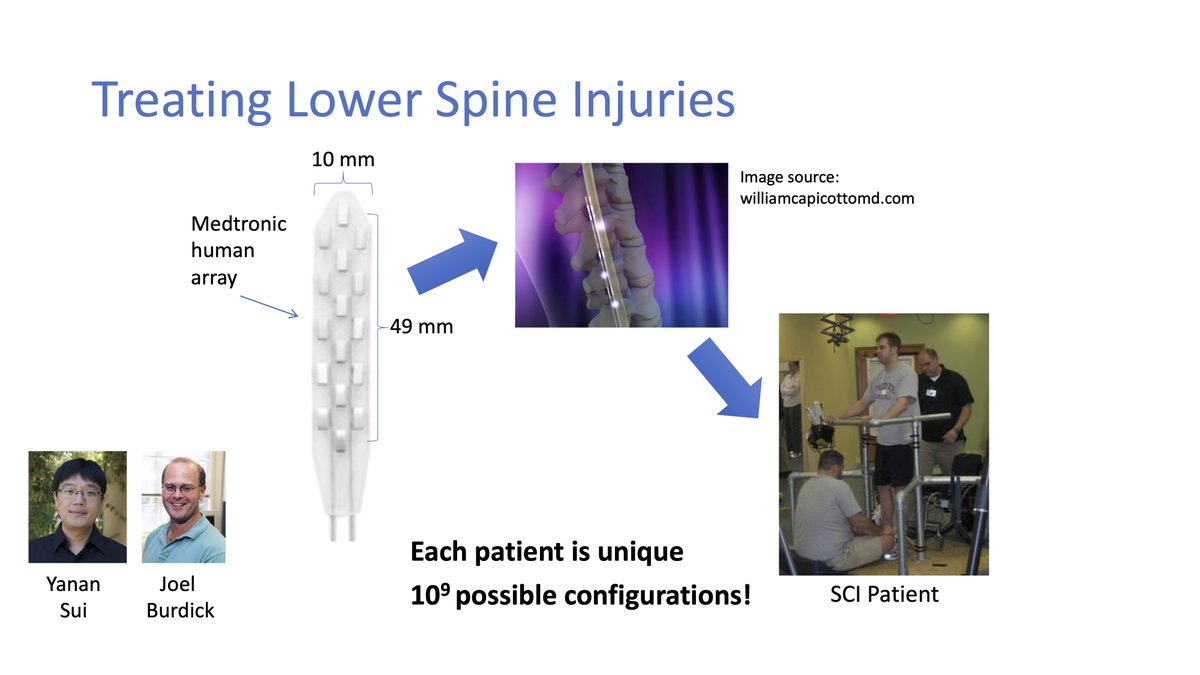
arxiv.org/abs/1909.12316
(@icra2020 Best Paper Award)
Project: roams.caltech.edu
3/N
@GuanyaShi.
arxiv.org/abs/1811.08027
caltech.edu/about/news/neu…
4/N
arxiv.org/abs/2003.02992
caltech.edu/about/news/mac…
5/N
arxiv.org/abs/2002.11807
caltech.edu/about/news/mac…
6/N
arxiv.org/abs/2004.00422
7/N
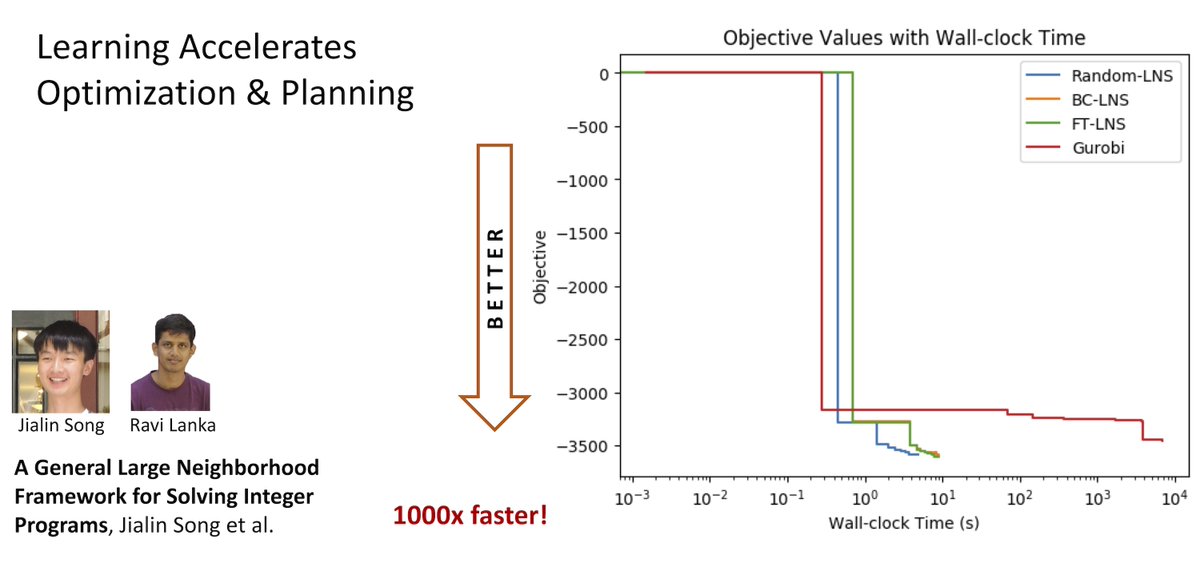
8/N
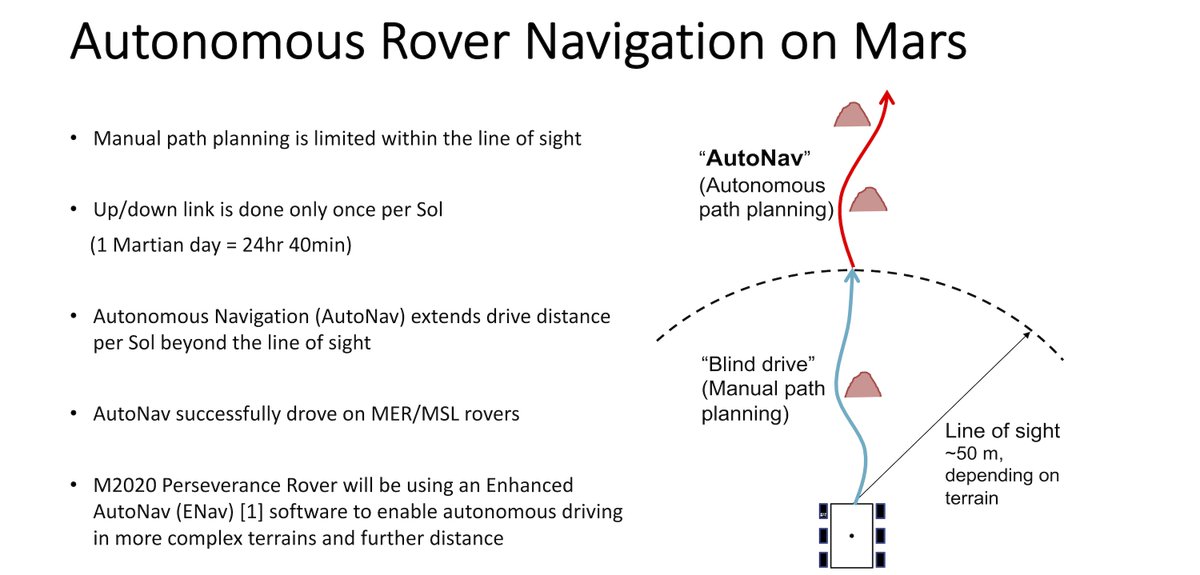
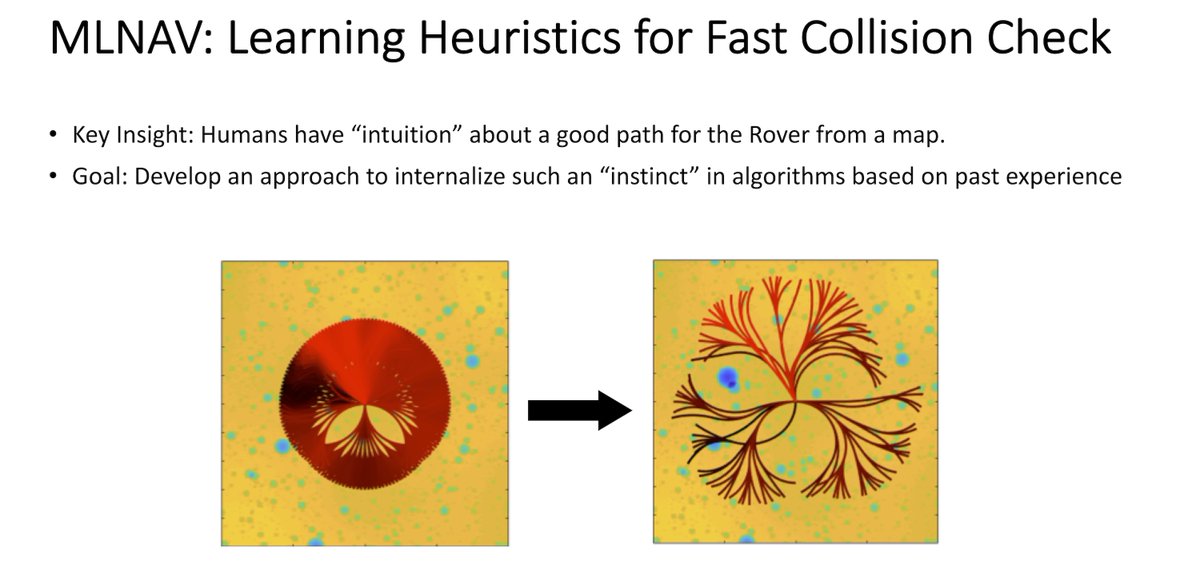
arxiv.org/abs/1906.05819
arxiv.org/abs/2005.04374
arxiv.org/abs/1912.10099
9/N
neurosymbolic.org
Relevant papers:
arxiv.org/abs/2007.12101
arxiv.org/abs/1907.05431
10/N
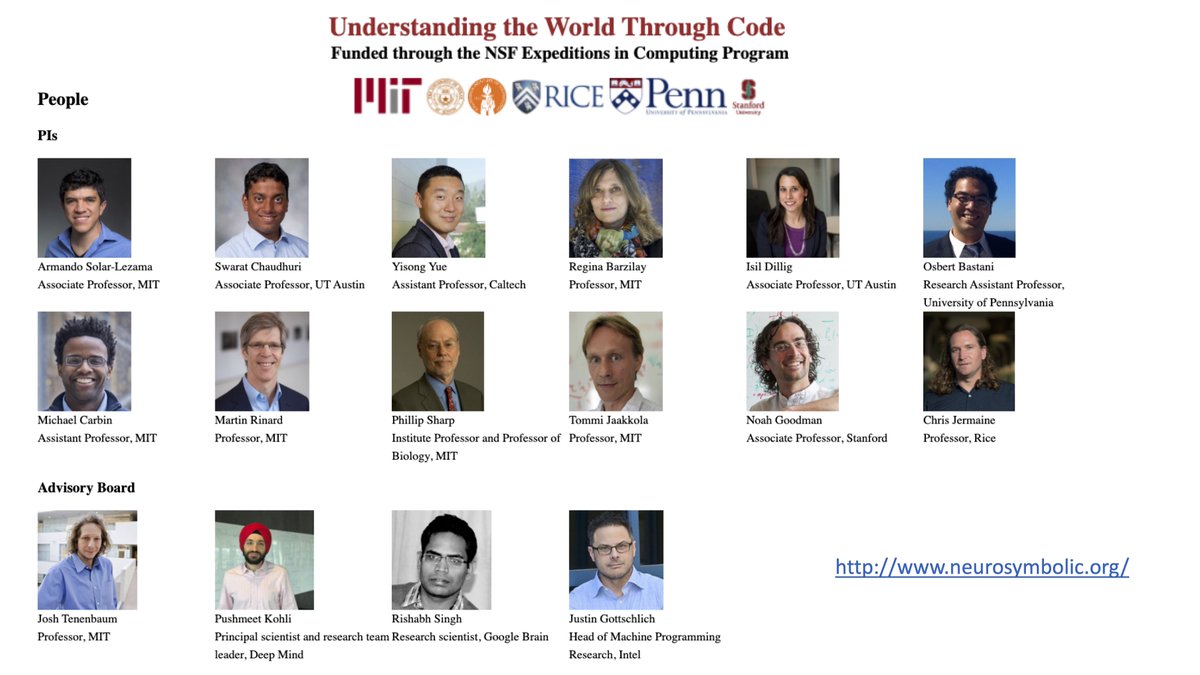
people.vision.caltech.edu/~eeyjolfs/beha…
Work led by Pietro Perona:
authors.library.caltech.edu/cgi/exportshel…
11/N
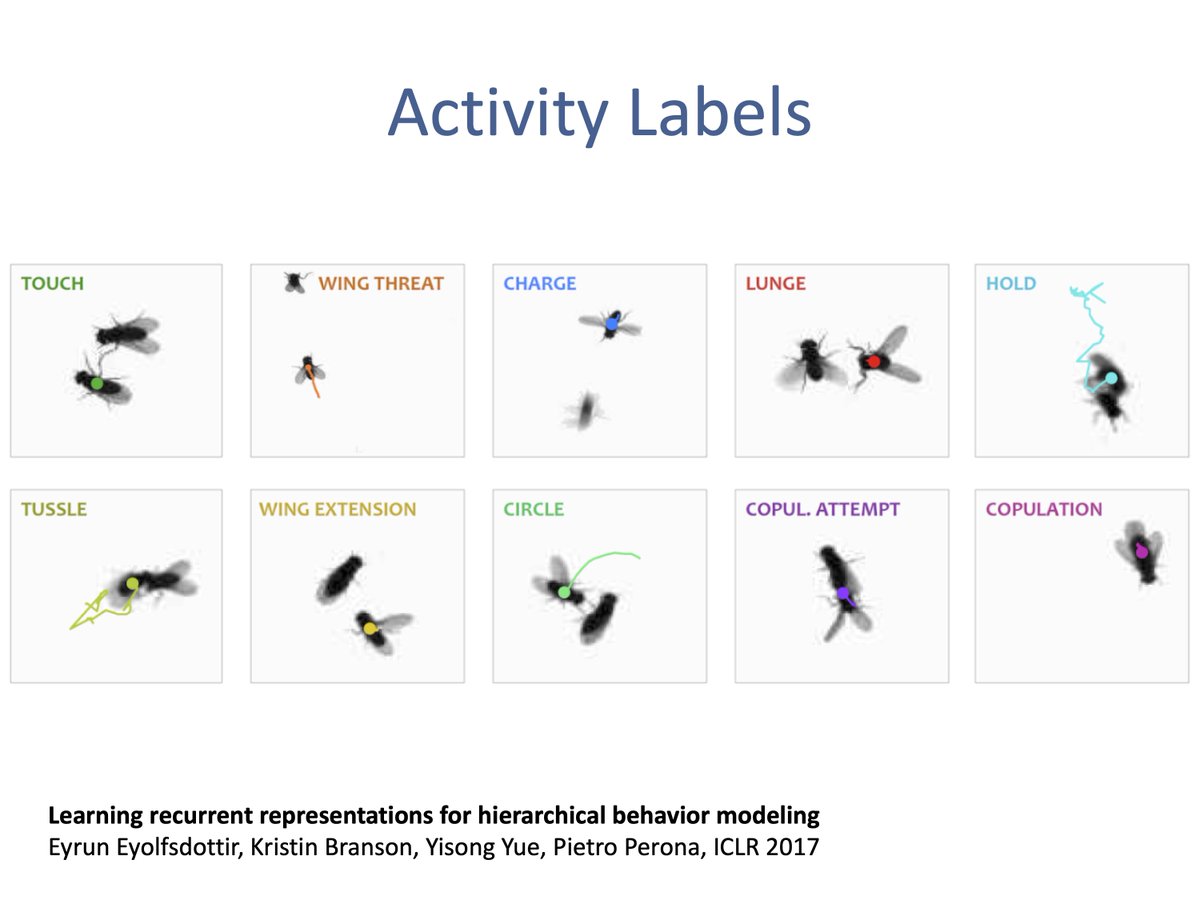
12/N
Sports Illustrated:
si.com/media/2016/06/…
Papers:
arxiv.org/abs/1703.03121
yisongyue.com/publications/i…
yisongyue.com/publications/s…
yisongyue.com/publications/c…
13/N
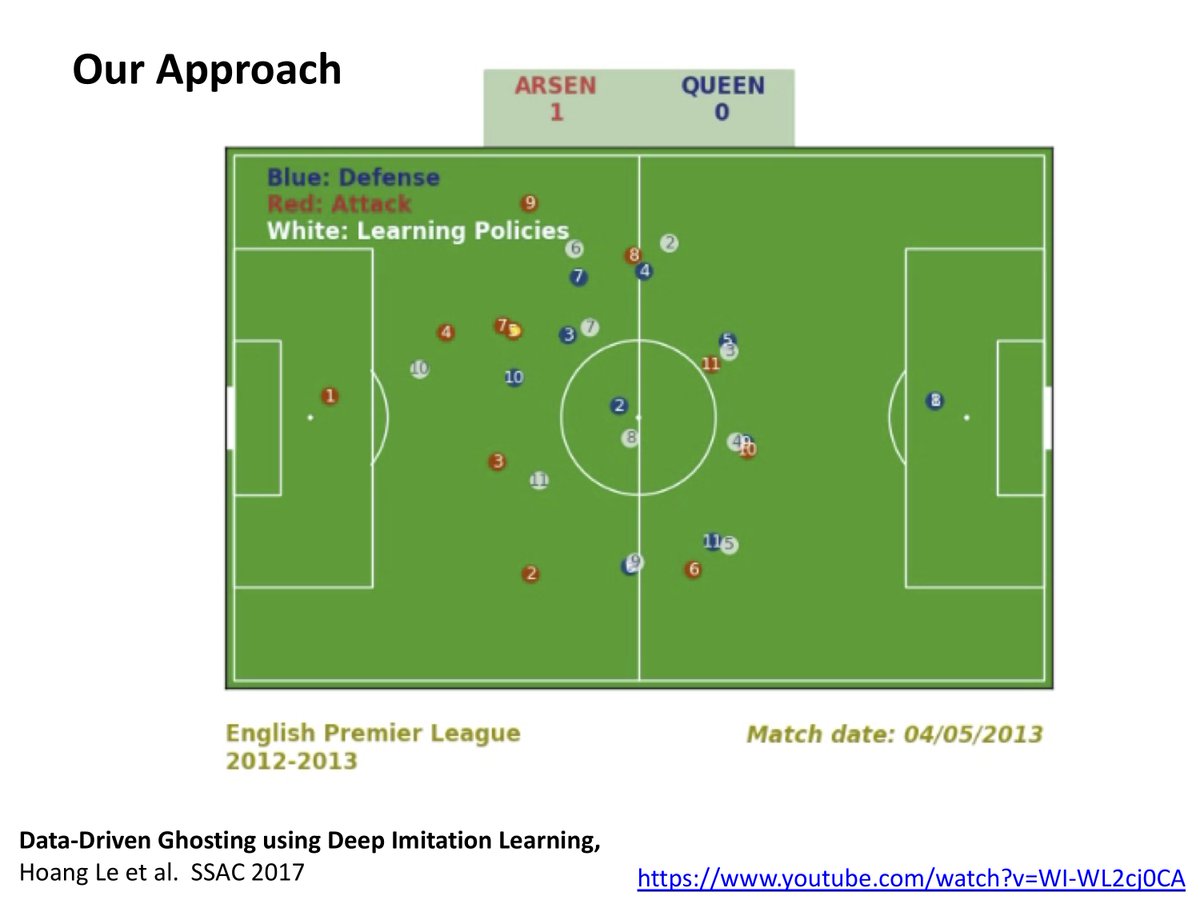
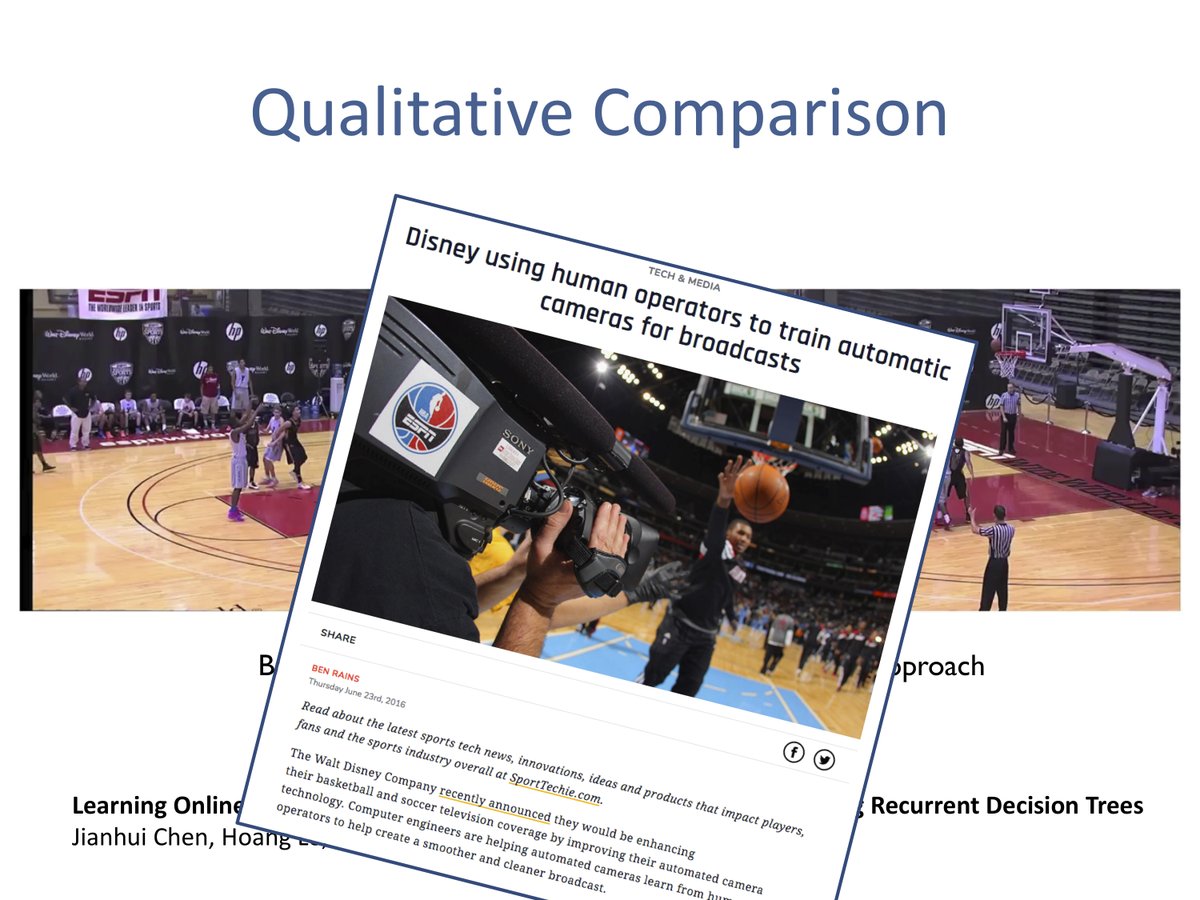
arxiv.org/abs/1811.07707
arxiv.org/abs/1811.00755
yisongyue.com/publications/a…
14/N
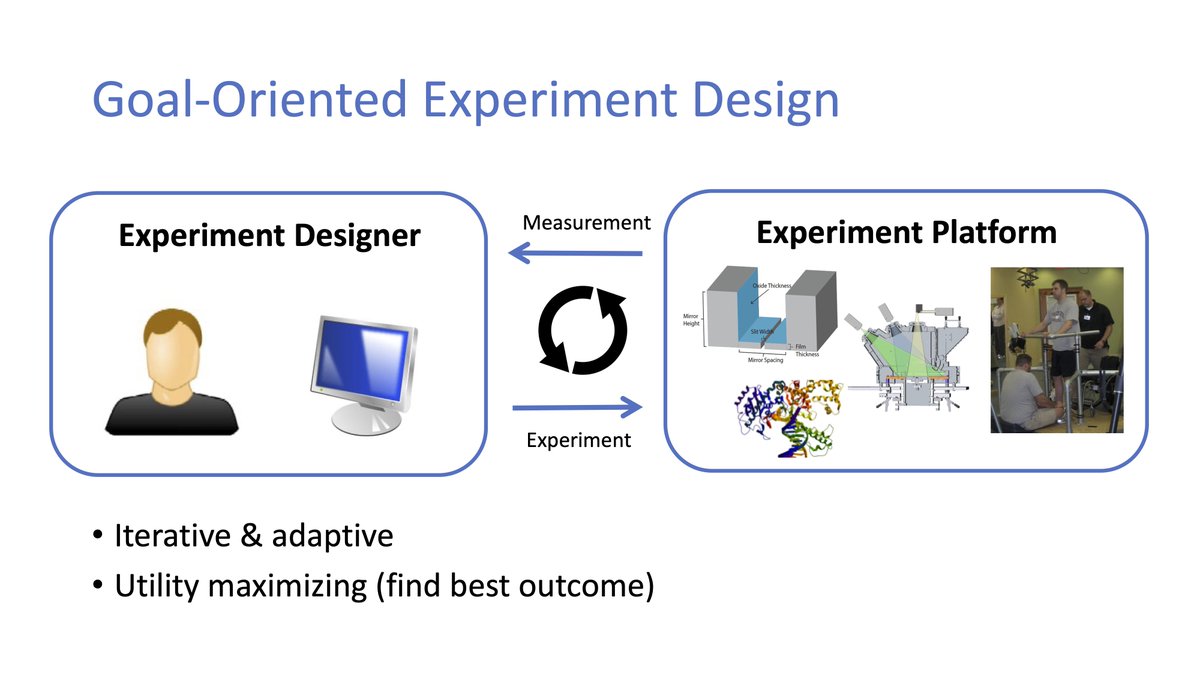
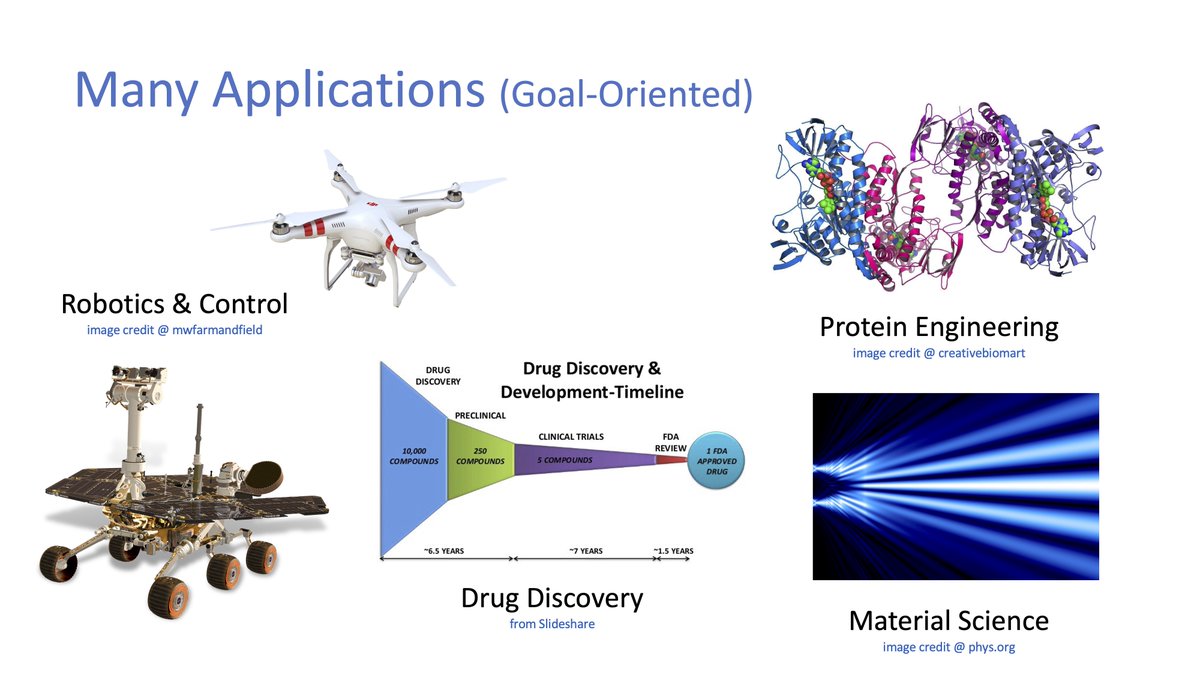
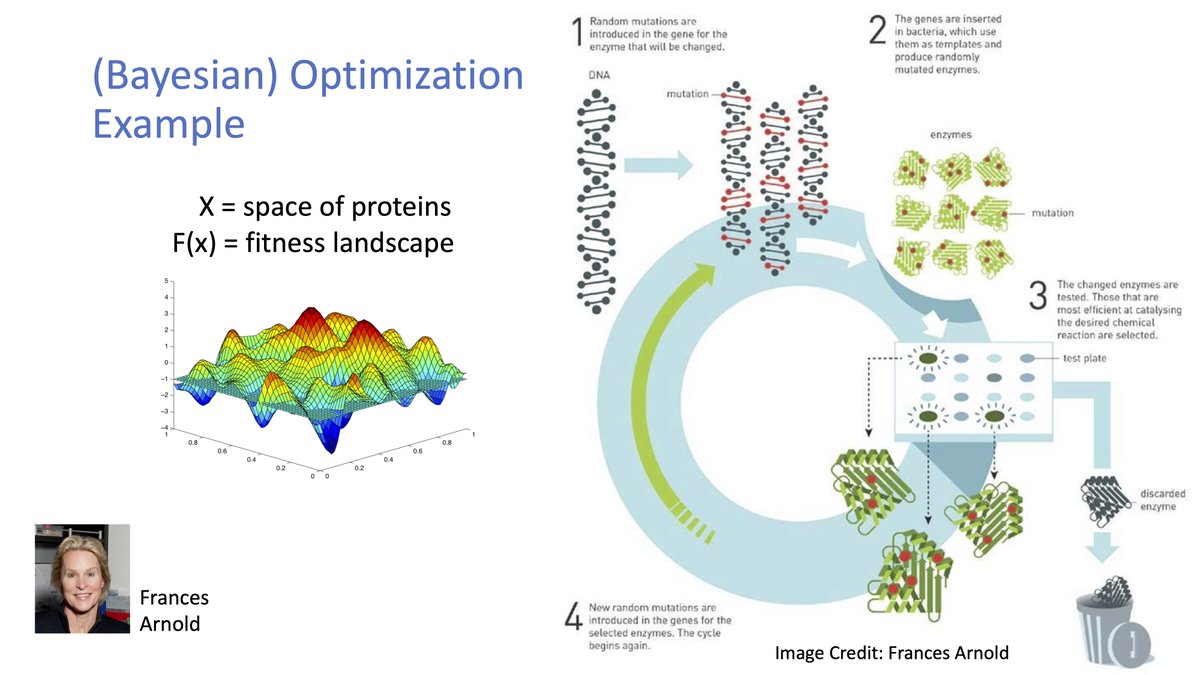
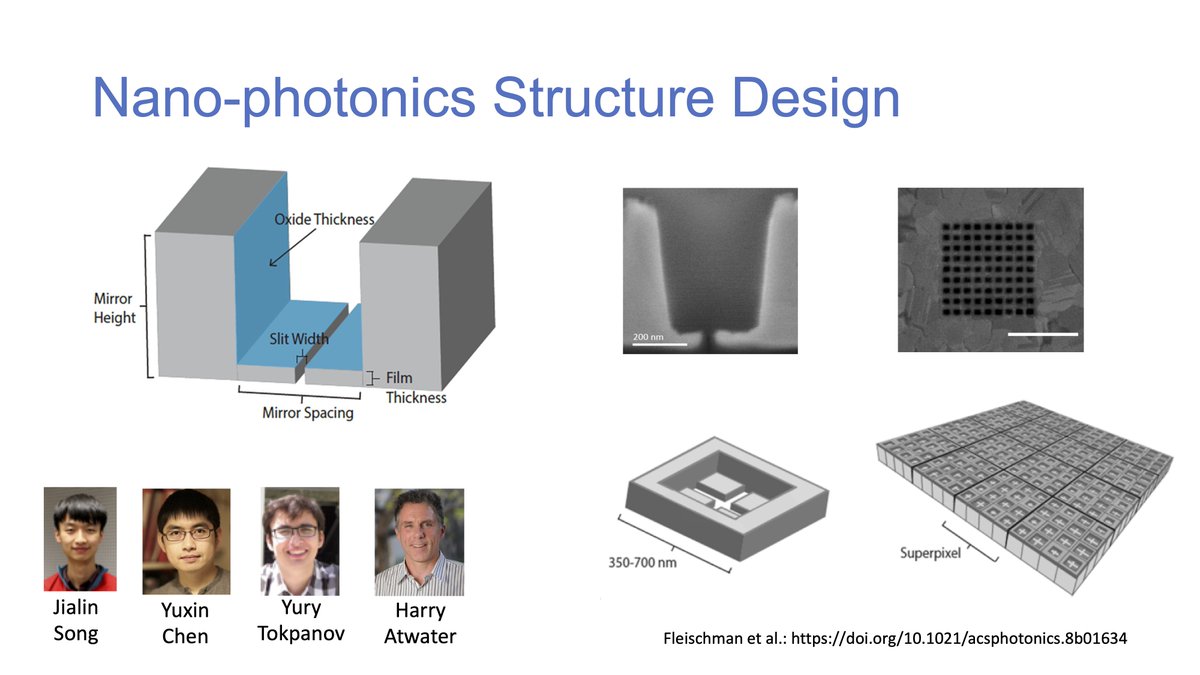
15/N
arxiv.org/abs/2007.04275
16/N
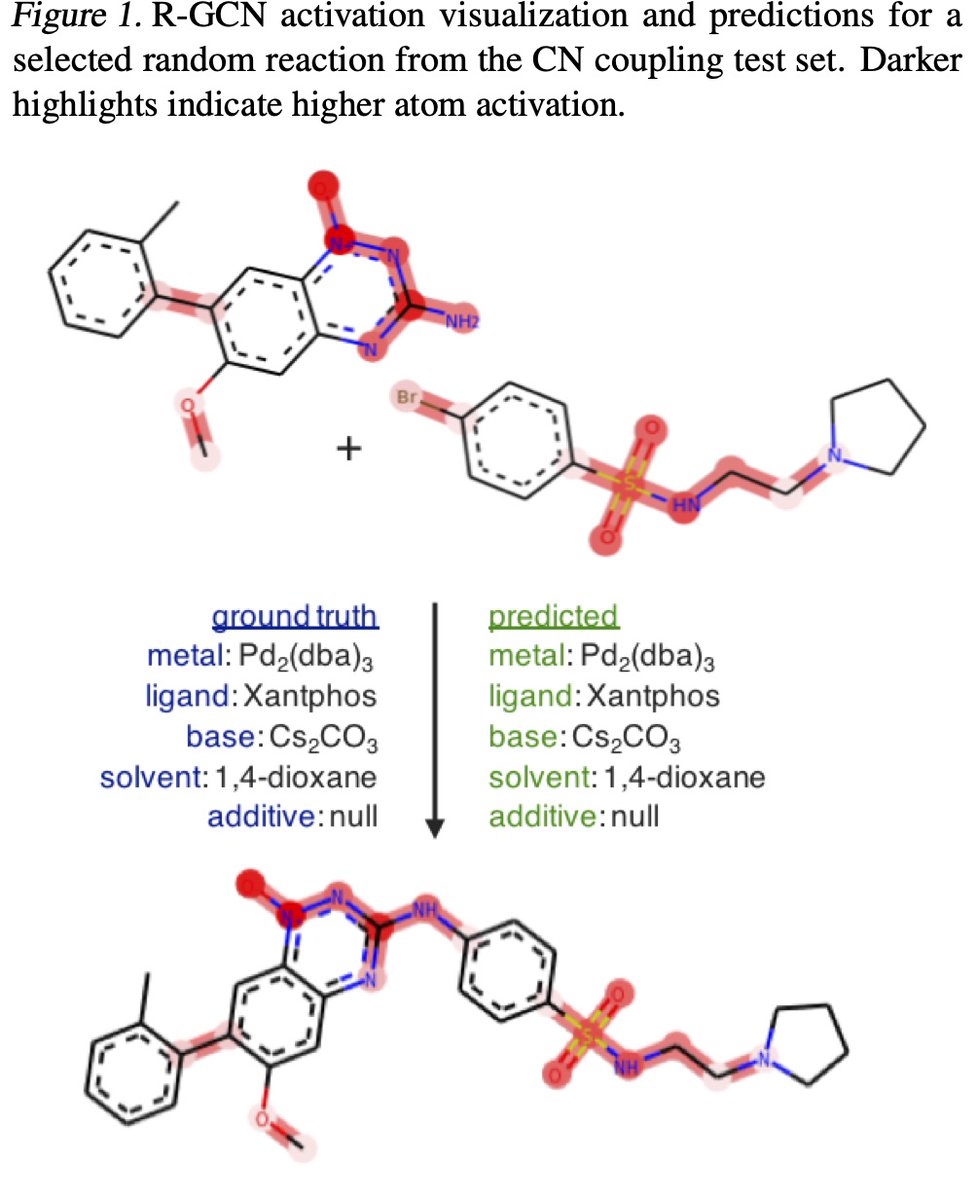
arxiv.org/abs/1809.02880
arxiv.org/abs/1901.03467
arxiv.org/abs/2002.02040
arxiv.org/abs/1907.00496
17/N
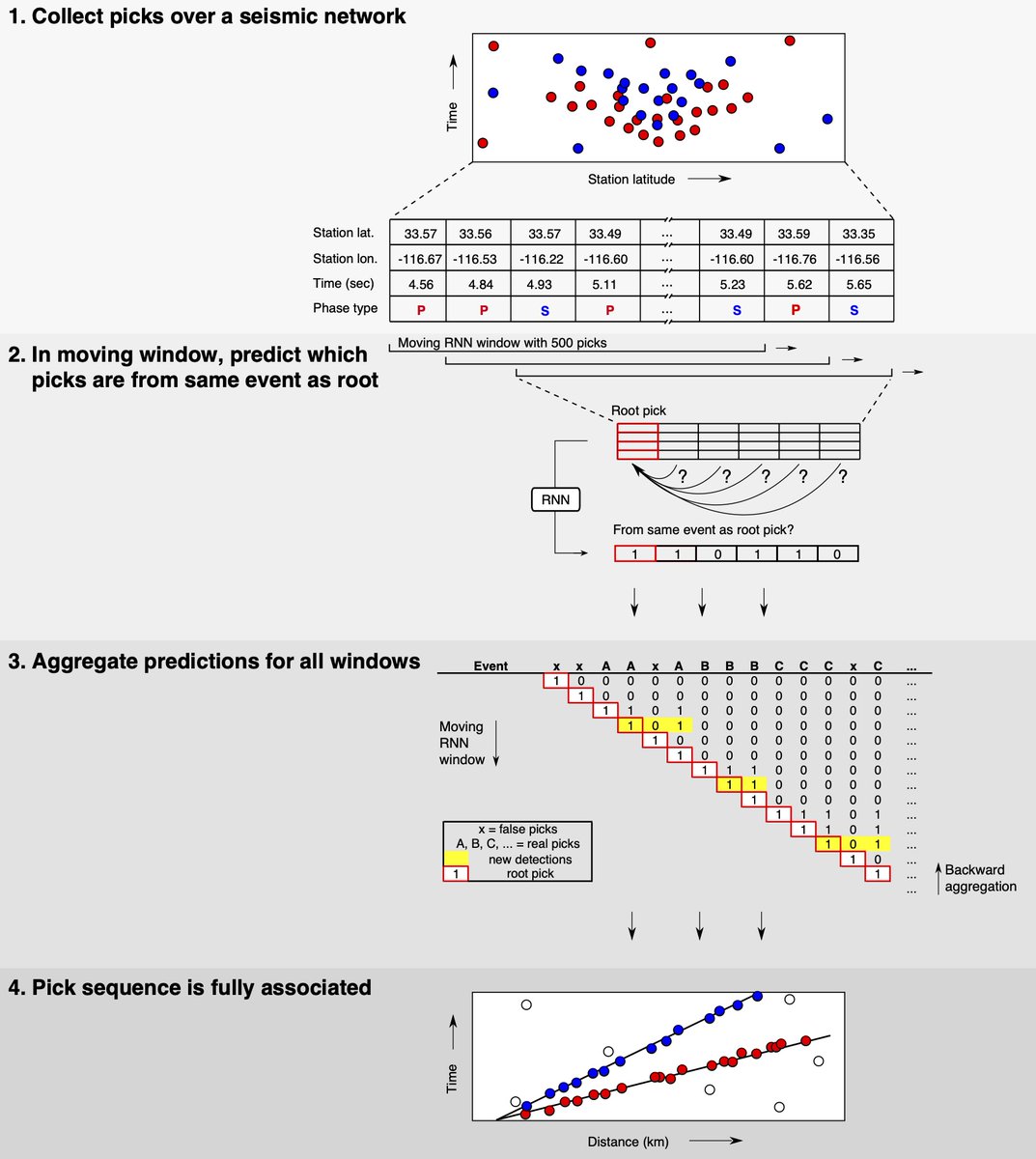
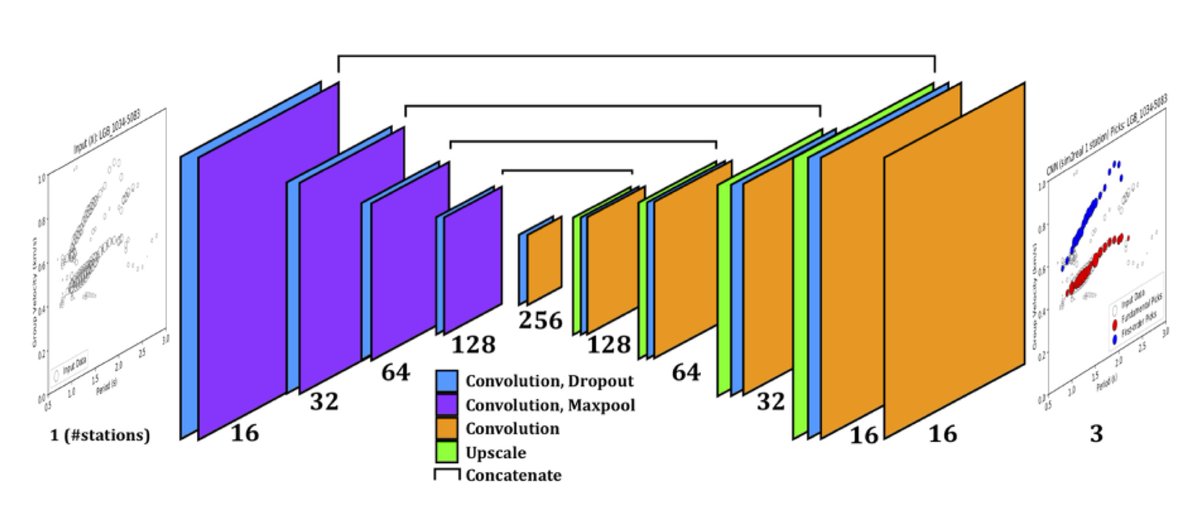
18/N
19/N
Here's a recent result by @_sun_he (postdoc w/ Katie):
cosense.cms.caltech.edu
20/N
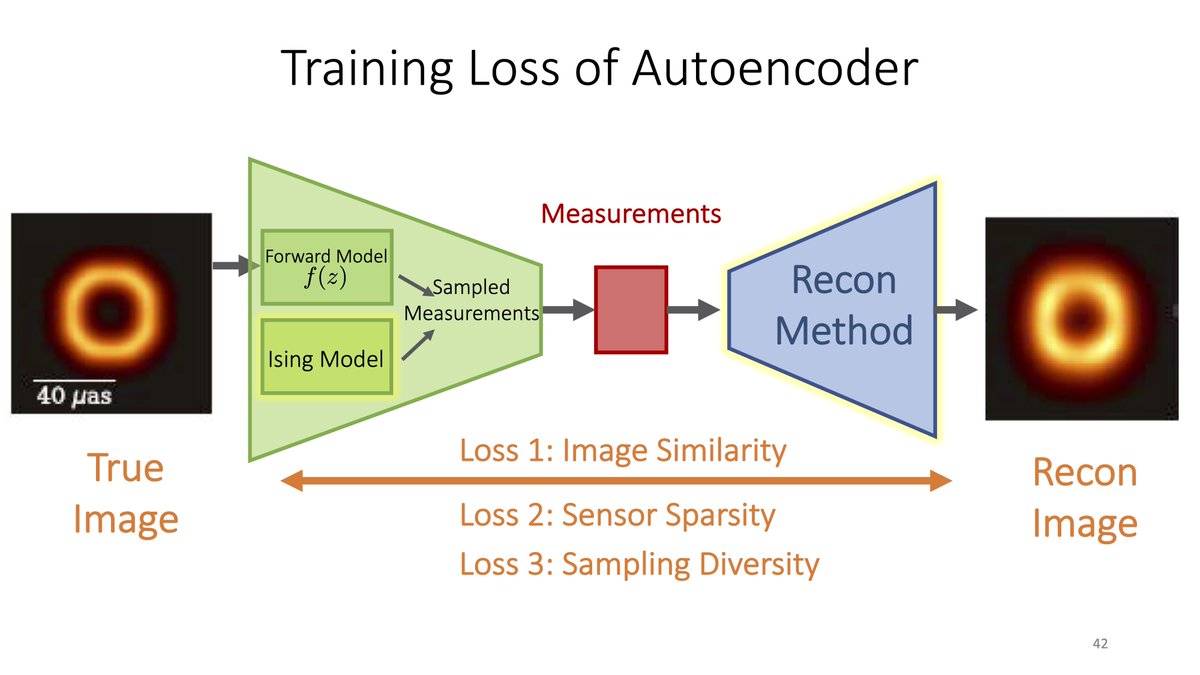
21/N
Online Optimization & Competitive Control:
arxiv.org/abs/2002.05318
Robust Regression for Safe Exploration:
arxiv.org/abs/1906.05819
Program Learning as Constrained Mirror Descent:
arxiv.org/abs/1907.05431
22/N
23/23