#Mathematics #COVIDー19
#mathematics
#mathematics
#mathematics #COVIDー19
#Mathematics #COVIDー19
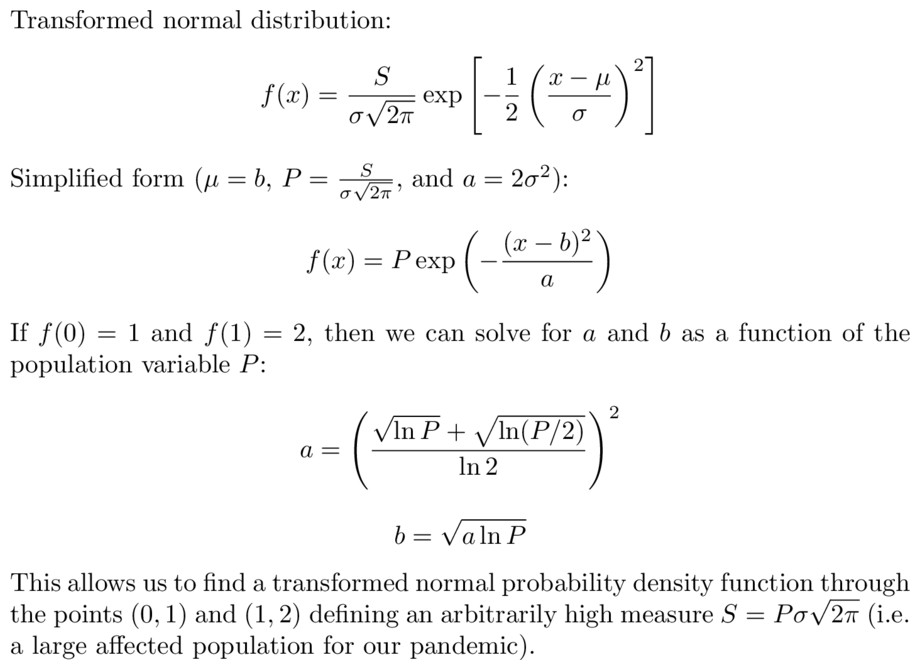
Well, naturally, the peak of the distribution gets higher and higher.
Let's zoom in on the interval [0,1]...
Very little changes in this region! In other words, we can find a transformed normal N(a',b',P') which approximates our original normal N(a,b,P) for an arbitrarily large P'!
As interpretation: The little stretch of data we know, if it's on a tail of the normal distribution, could be basically *anywhere* in the pandemic's course.
#Mathematics #COVIDー19