I have zero confidence in the Santa Clara serology study, and the recent work of Eran Bendavid generally.
1. Poorly chosen, non-representative samples
2. Weakness at thinking through important Bayesian principles
3. Inconsistency with other, more verifiable results
4. Attachment to a particular idea (slow willingness to update on evidence)
This is, unfortunately, hard.
The NBA wasn't a randomly-selected employer; it was the first sports league to get a COVID positive.
Therefore, you need to be extremely mindful of a basic Bayesian principle, and especially so if those without COVID deeply outnumber those with it (and they do).
(There's some math to calculate this exactly if you're interested.)
This is a good record (99.5%) but unfortunately the sample size is not large enough to determine that for sure. It could be as low as 98.2%.
See the problem here? Given what we know of the accuracy of the test, you could still get that 1.5% result even on an entirely COVID-free sample and it wouldn't be particularly surprising.
If we take the estimate above--an 0.12% to 0.2% death rate from the virus--as a given, what number of total cases do we get in NYC?
nypost.com/2020/04/17/nyc…
These results are--again, let me say what I mean--preposterous.
The failure to grapple with any of these counterexamples--where the evidence on the ground is just obviously inconsistent with the study--does not inspire confidence.
"Alan, you're not an expert."
True, but graduate-level statistics (or maybe even precocious undergrad) is all you need here. If you want I can link people in more-biological fields with similar critiques.
Evaluating arguments on merits >> credential games.
"Alan, this seems contrary to the norms of being nice and collegial"
Yeah. A few reasons for that.
First, because getting this right is important.
Second, collegiality norms can inhibit that: more mild-mannered communication may not get the message across.
You see what they're doing.
I'm out of the field, I don't care whether people think I was too mean. So I can say it; the stats here are bad, folks. Really bad.
So I'm saying what I believe.
I believe he's much closer to the correct statistical approach than the original study.
However, for what it's worth, I'm a middle-ground person, neither hostile to reopening, nor demanding it immediately.
medium.com/@balajis/peer-…
The answer is that is greater than the total number of people. "New York City" here, in all uses, refers to combined New York, Bronx, Kings, Queens, and Richmond counties, not the MSA.
- Andrew Gelman, director of the Applied Statistics Center at Columbia University stat.columbia.edu/~gelman/
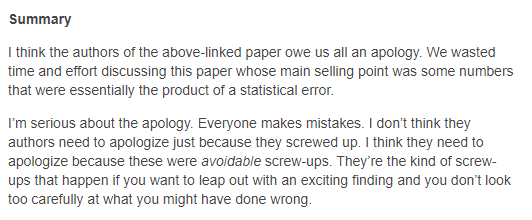