david-salazar.github.io/2020/04/17/fat…
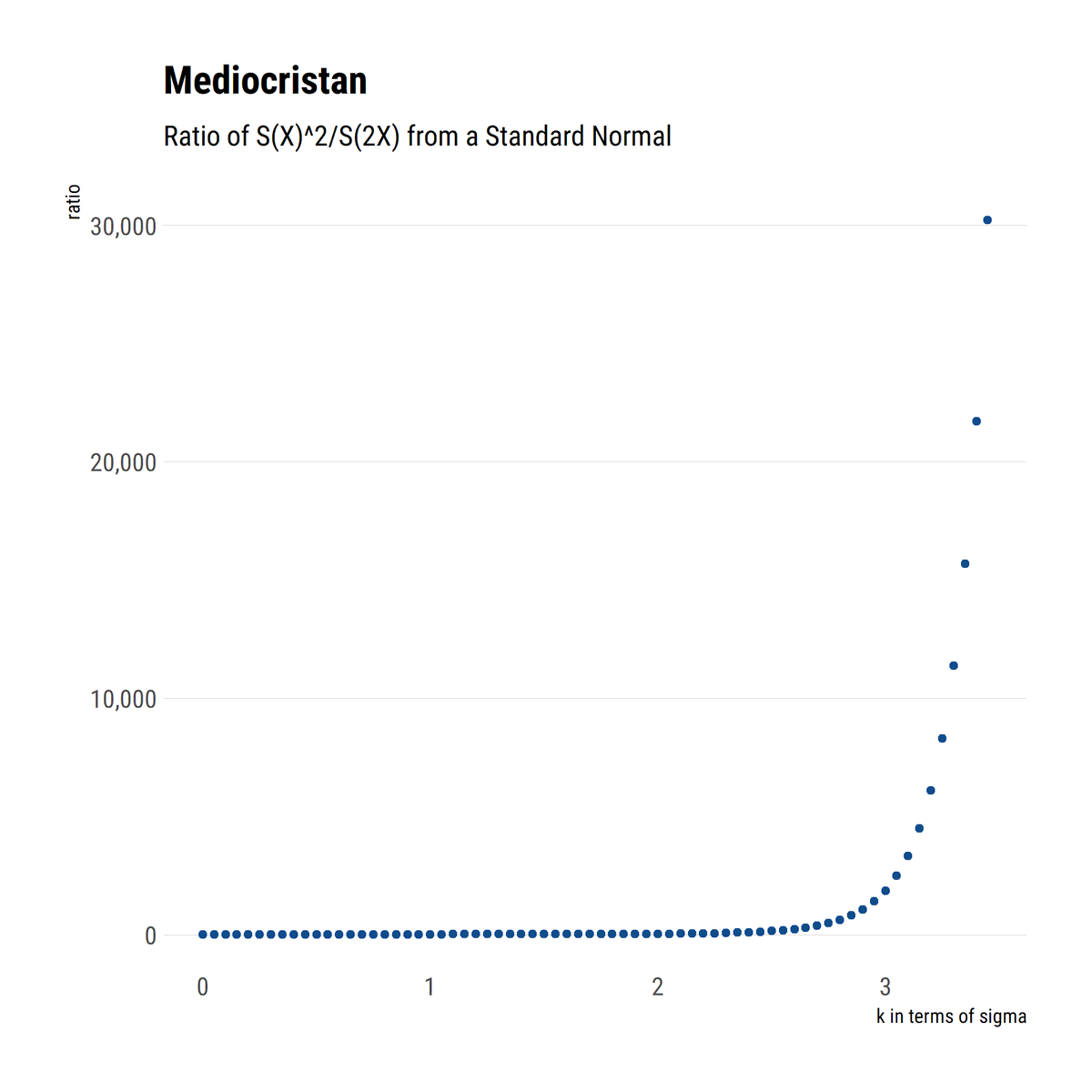
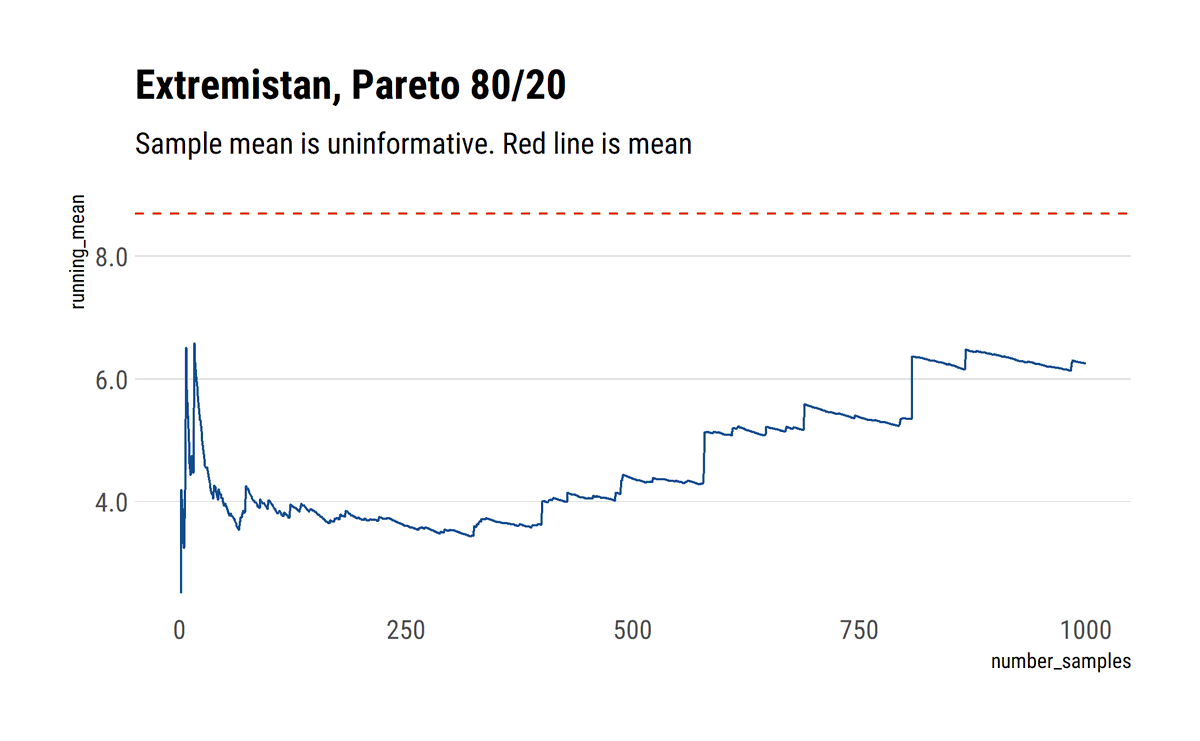
david-salazar.github.io/2020/05/09/wha…
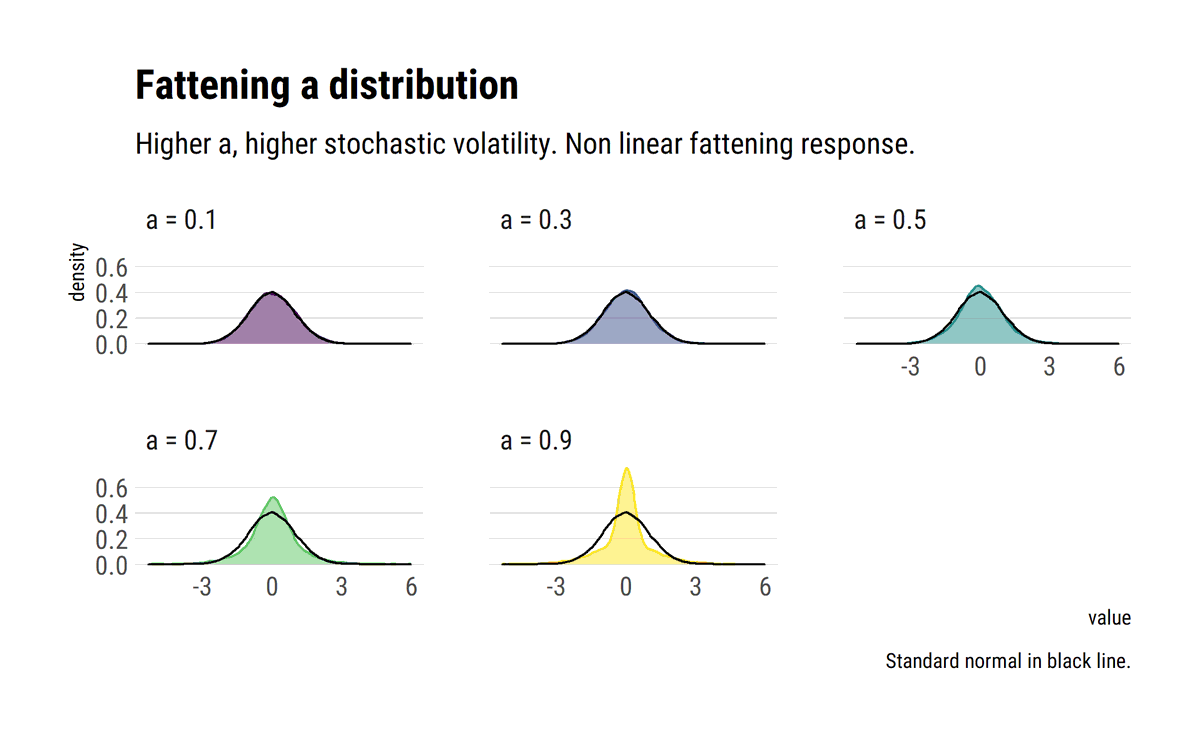
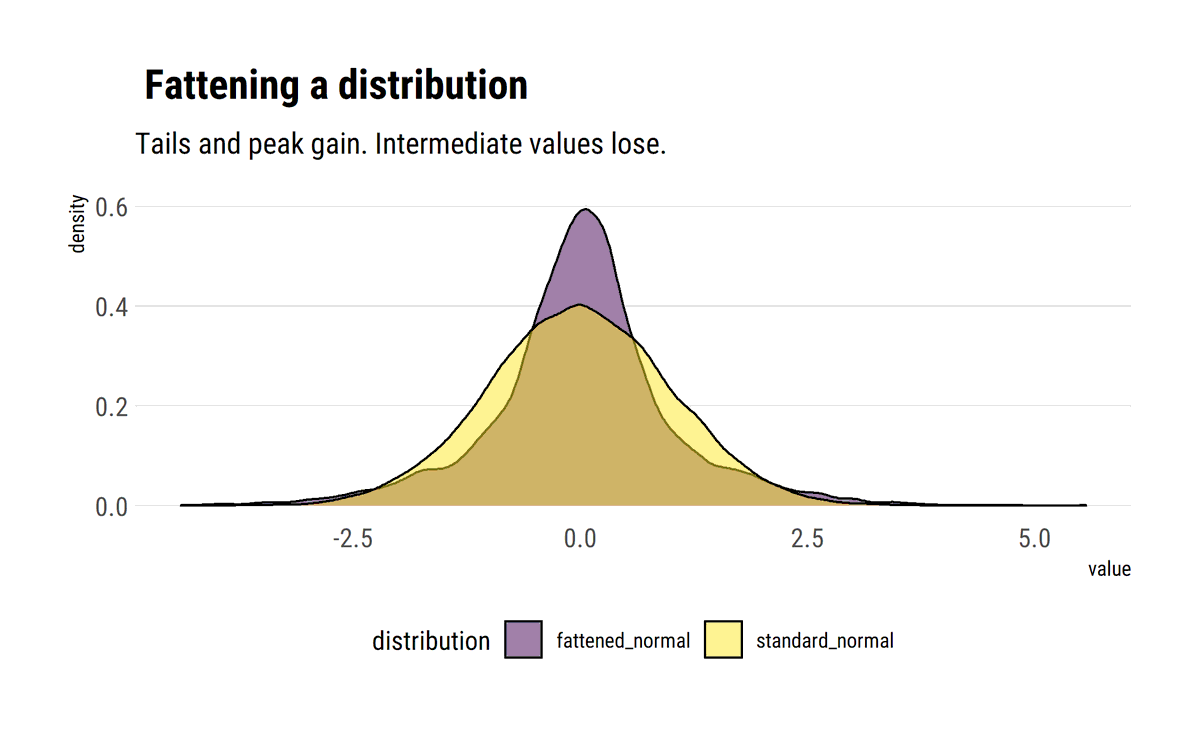
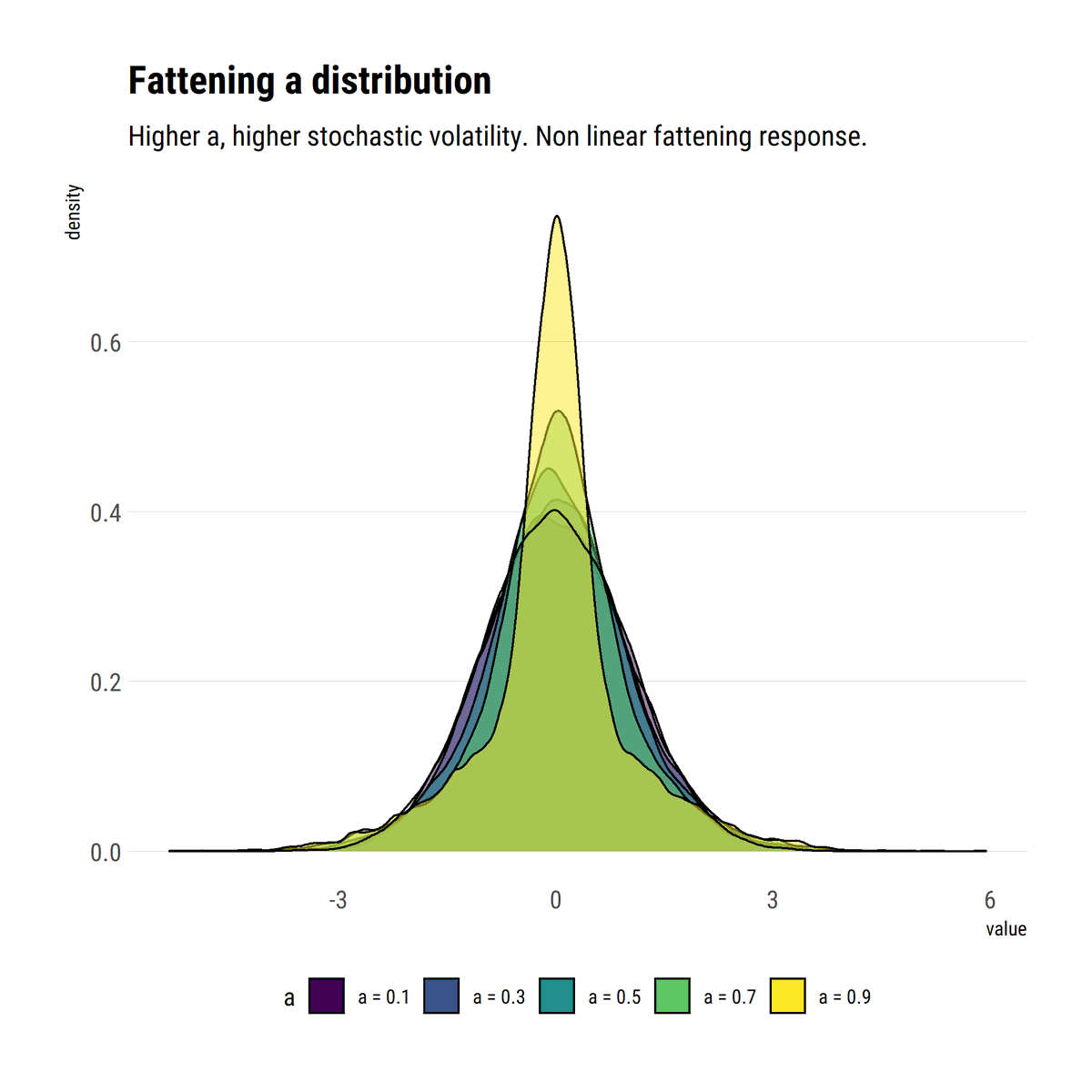
david-salazar.github.io/2020/05/13/sta…
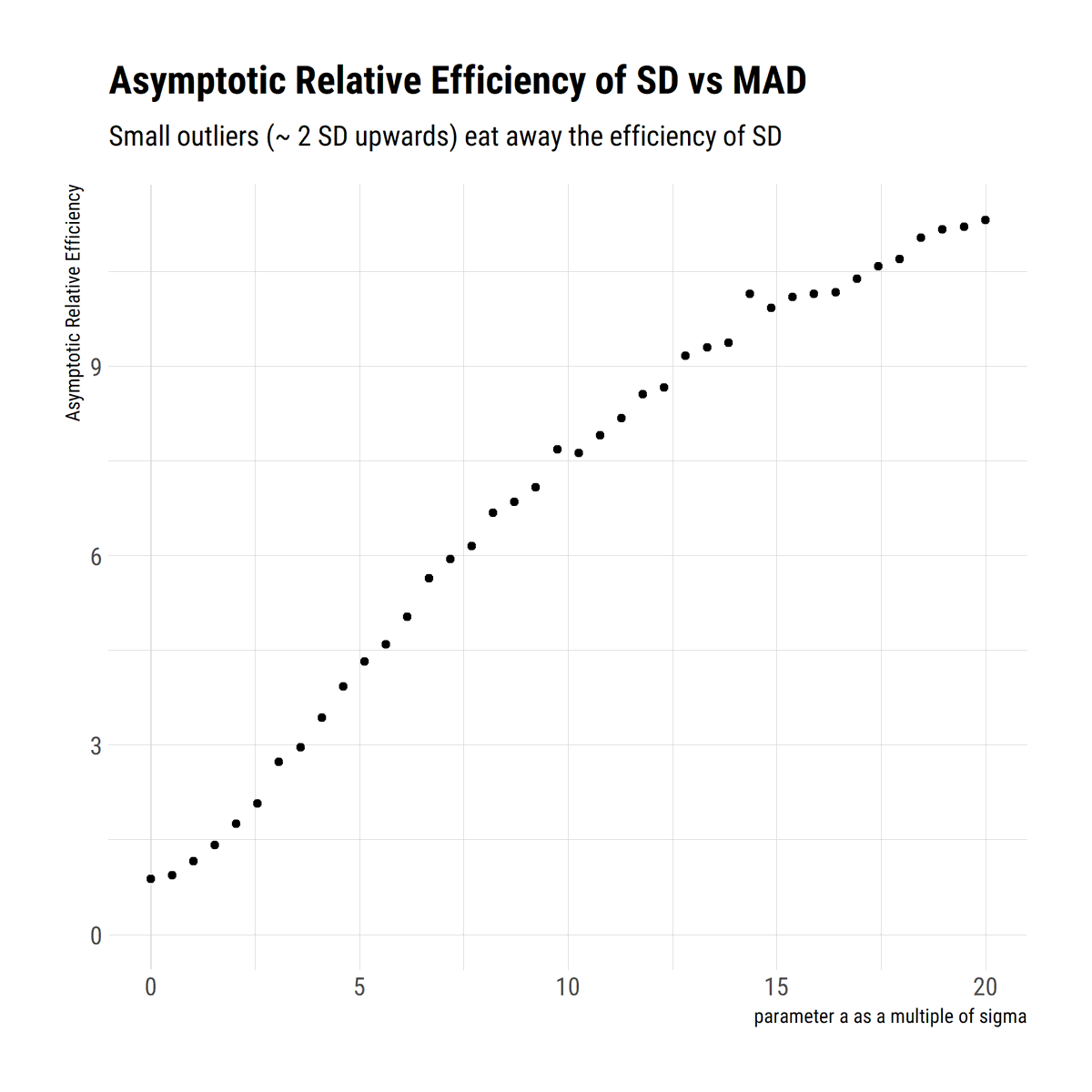
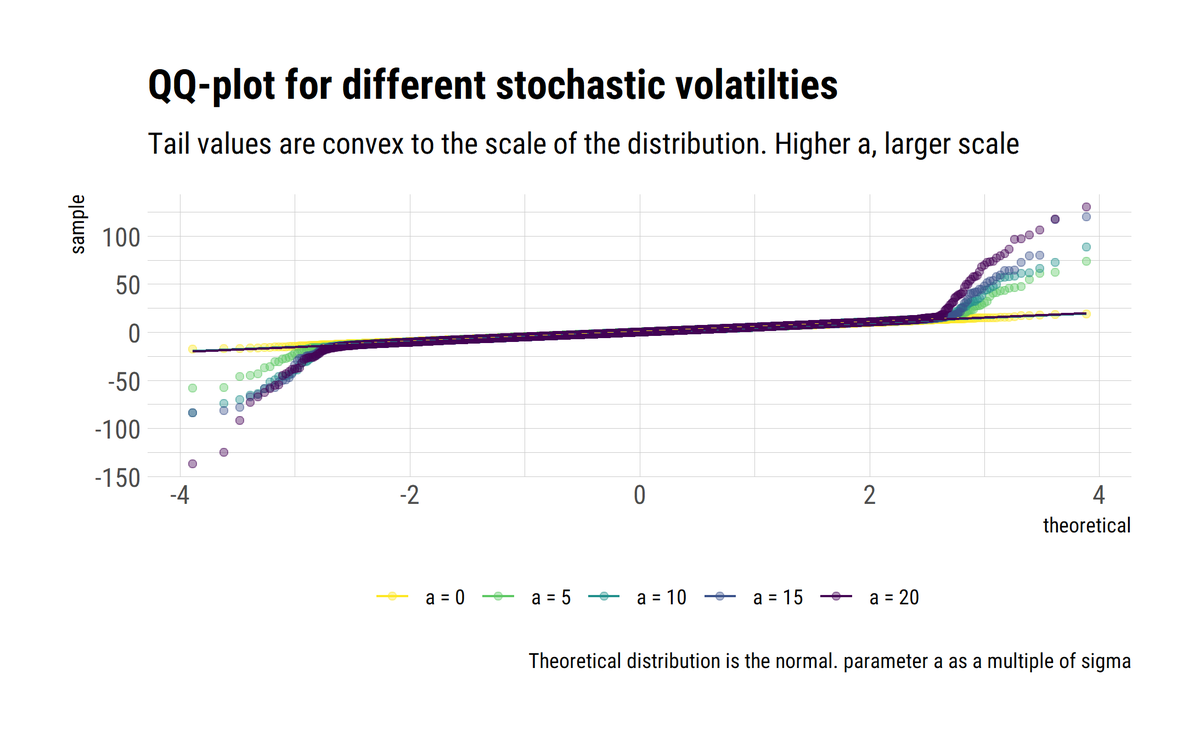
david-salazar.github.io/2020/05/19/und…
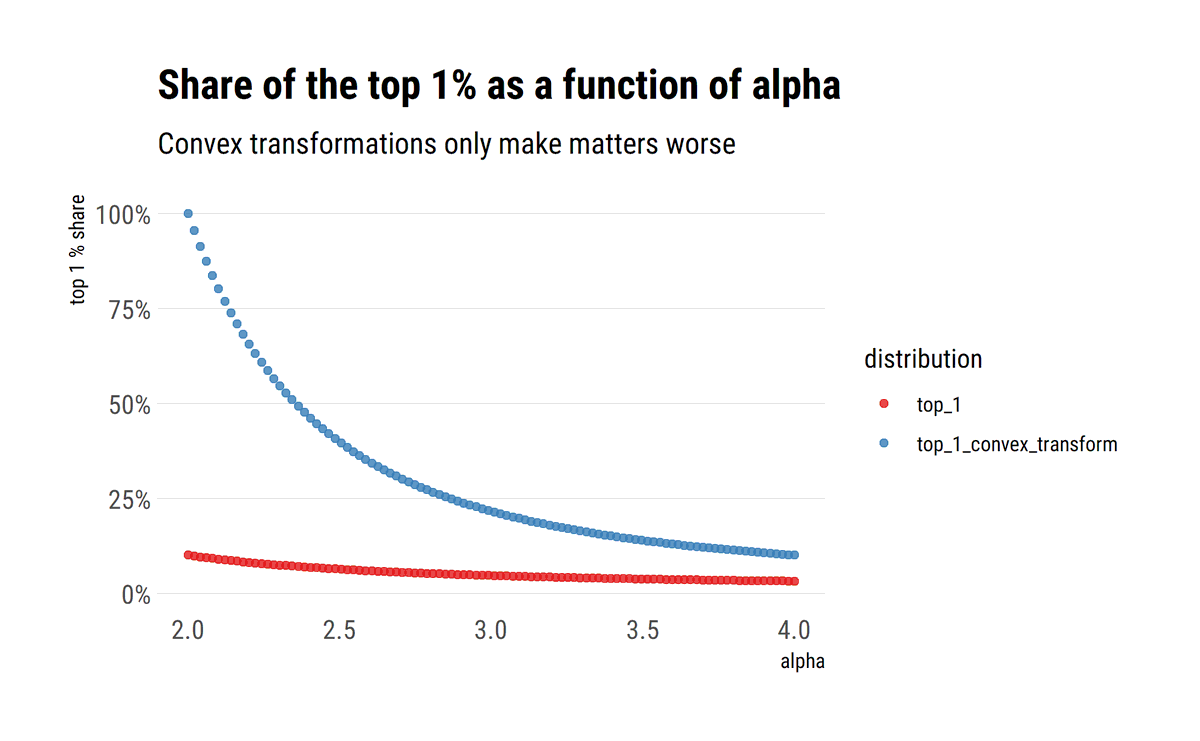
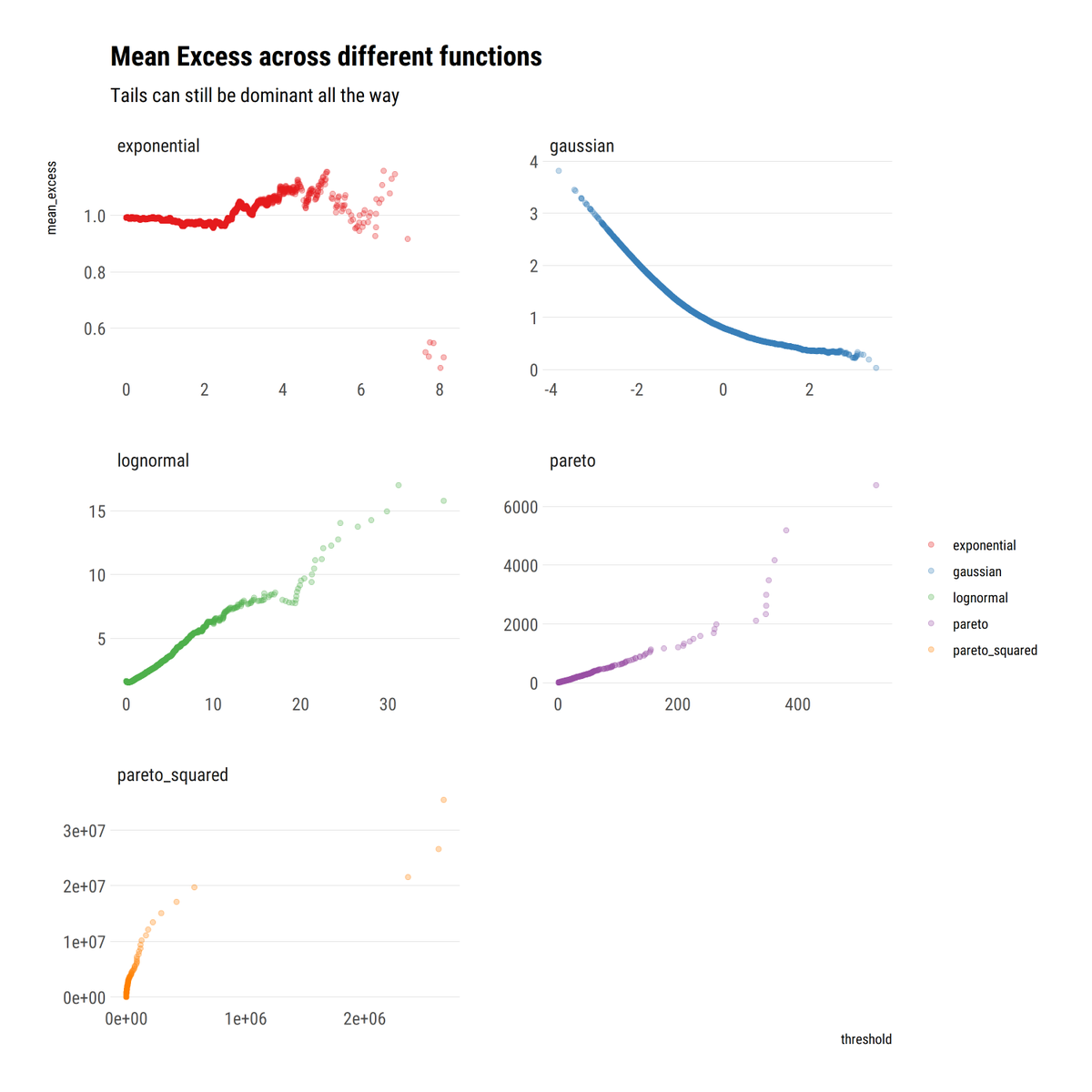
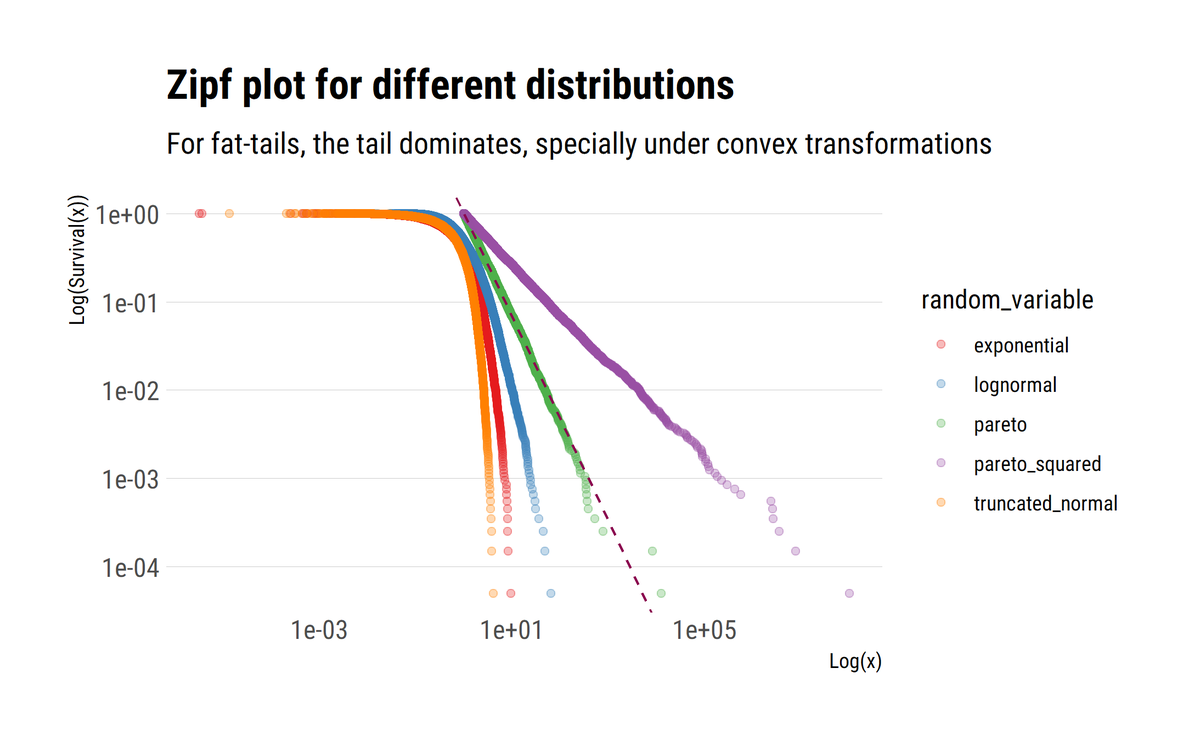
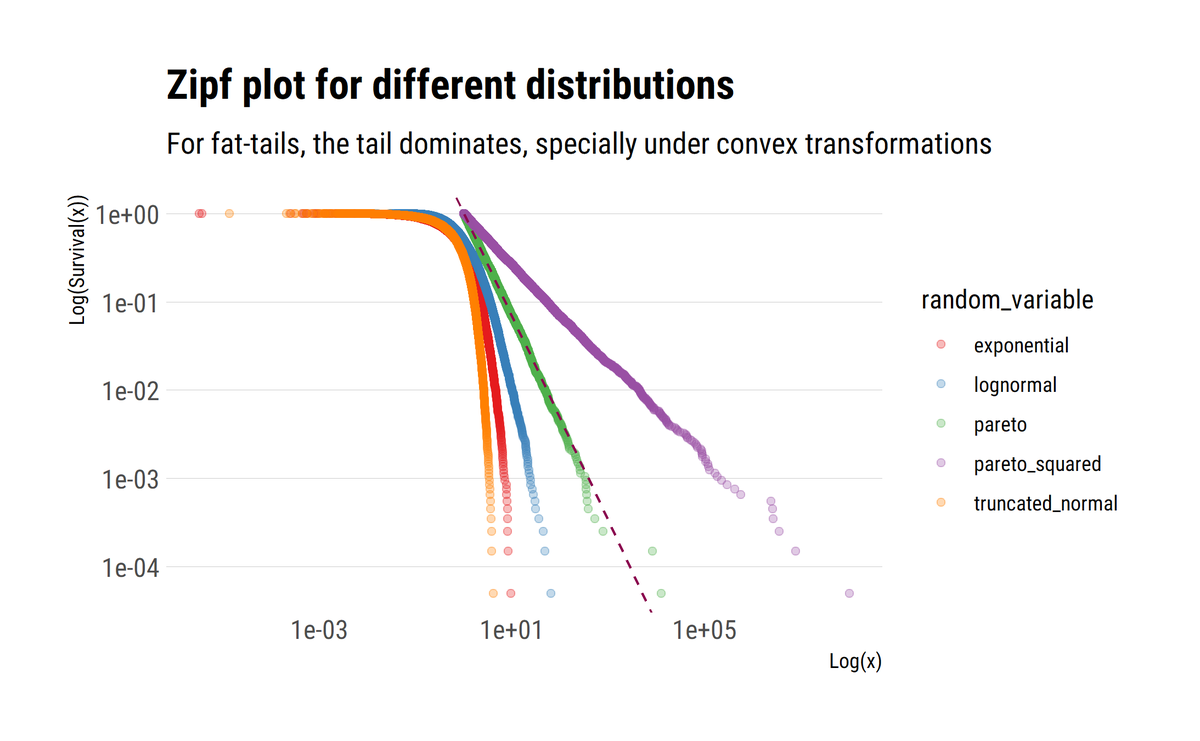
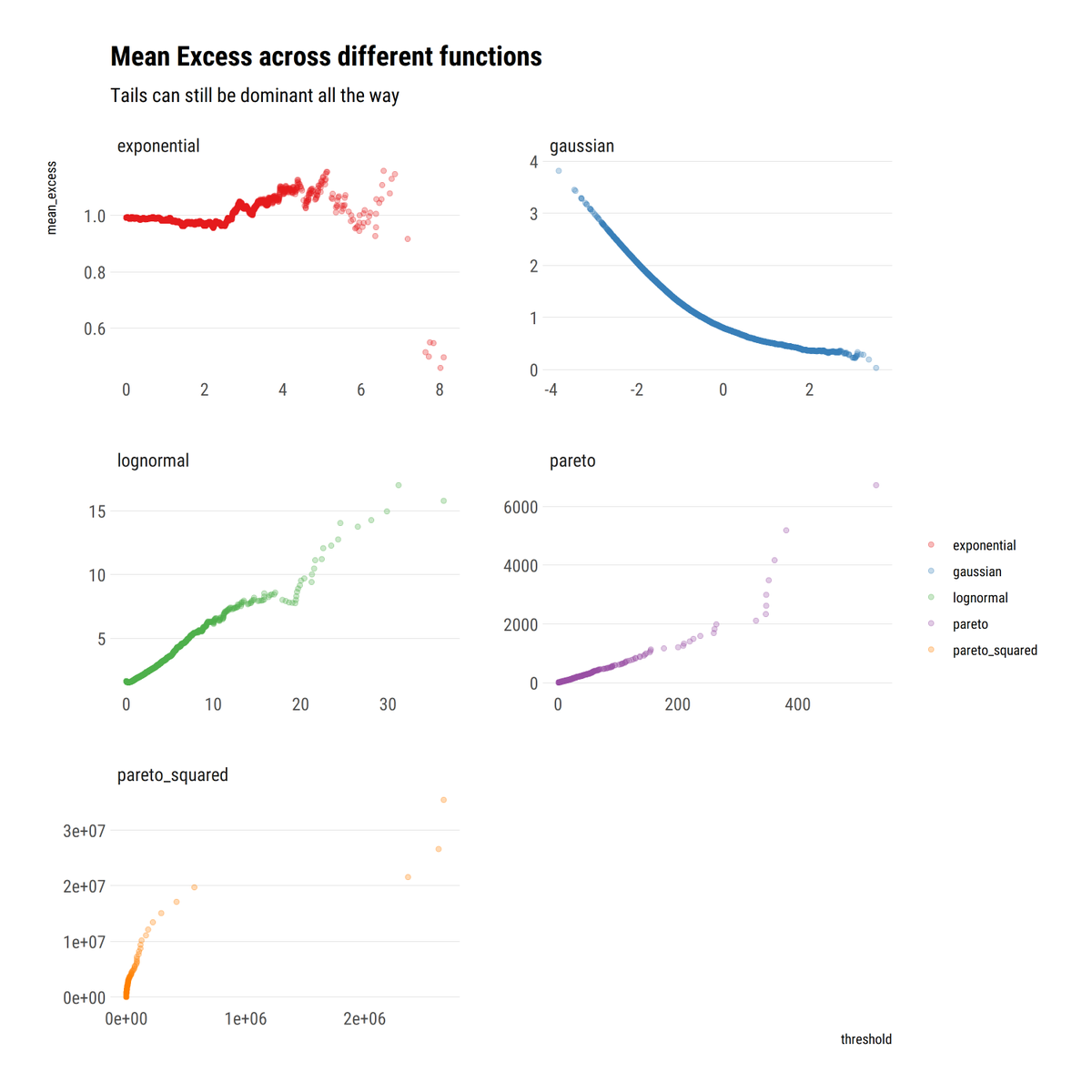
Blogpost: david-salazar.github.io/2020/05/22/cor…
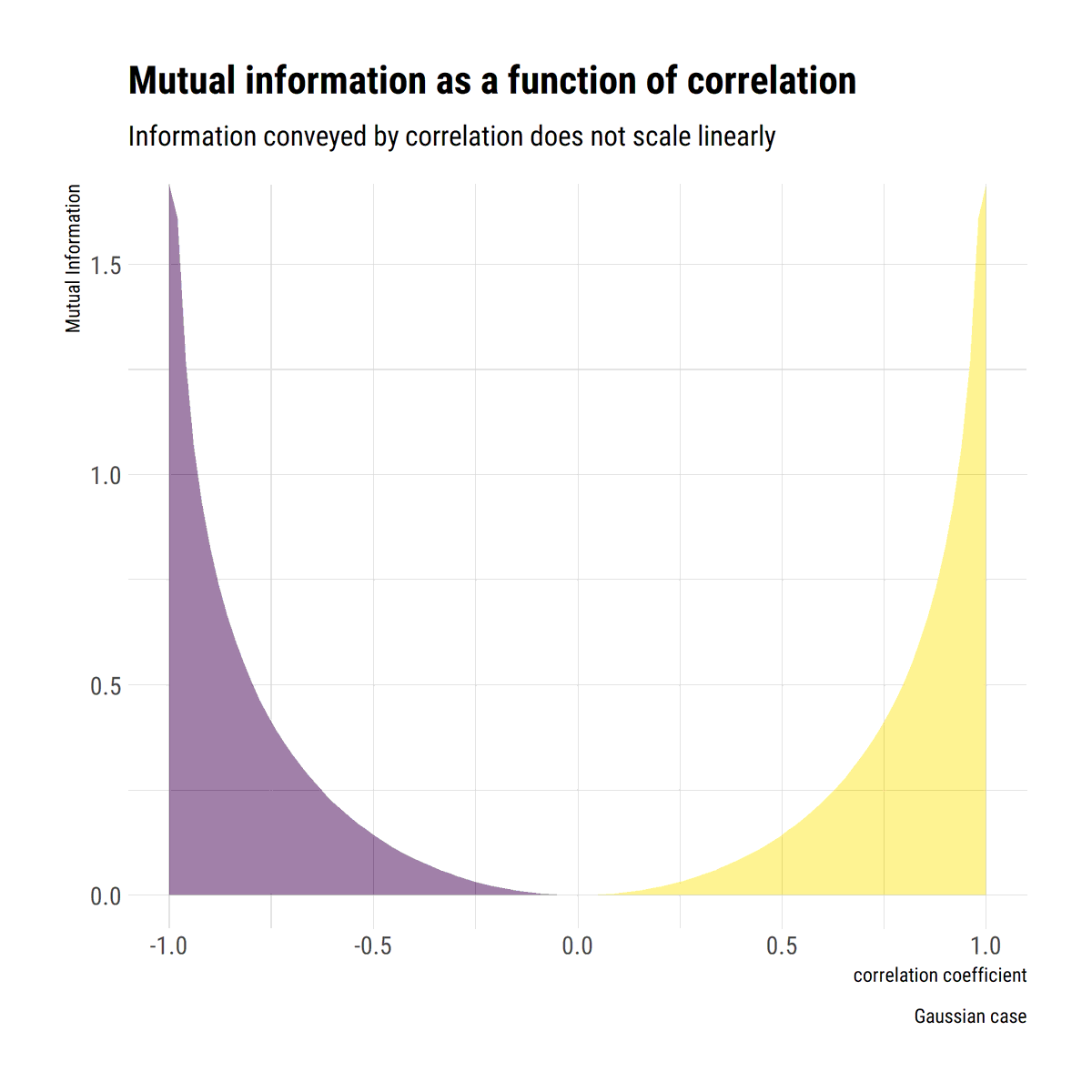
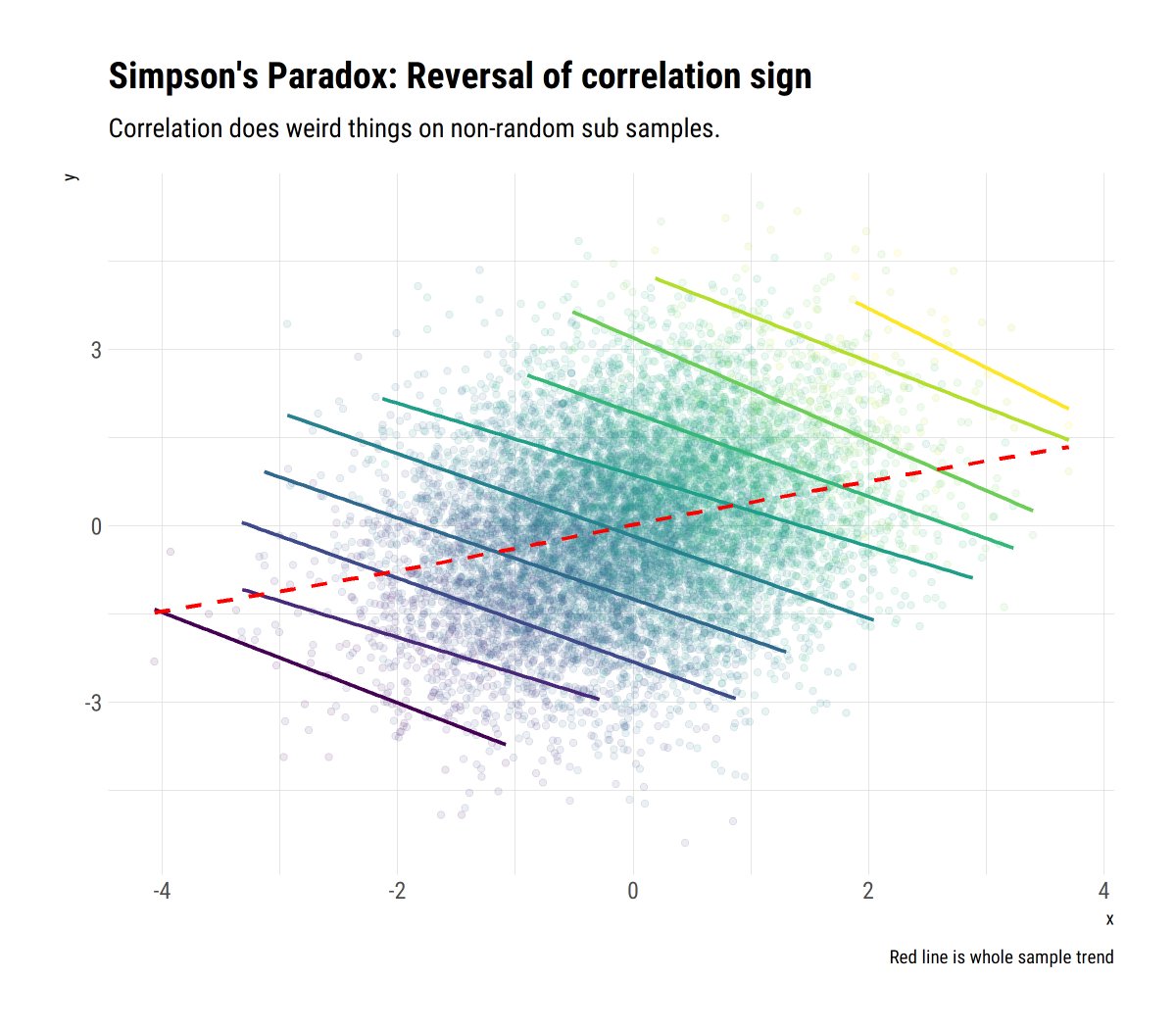
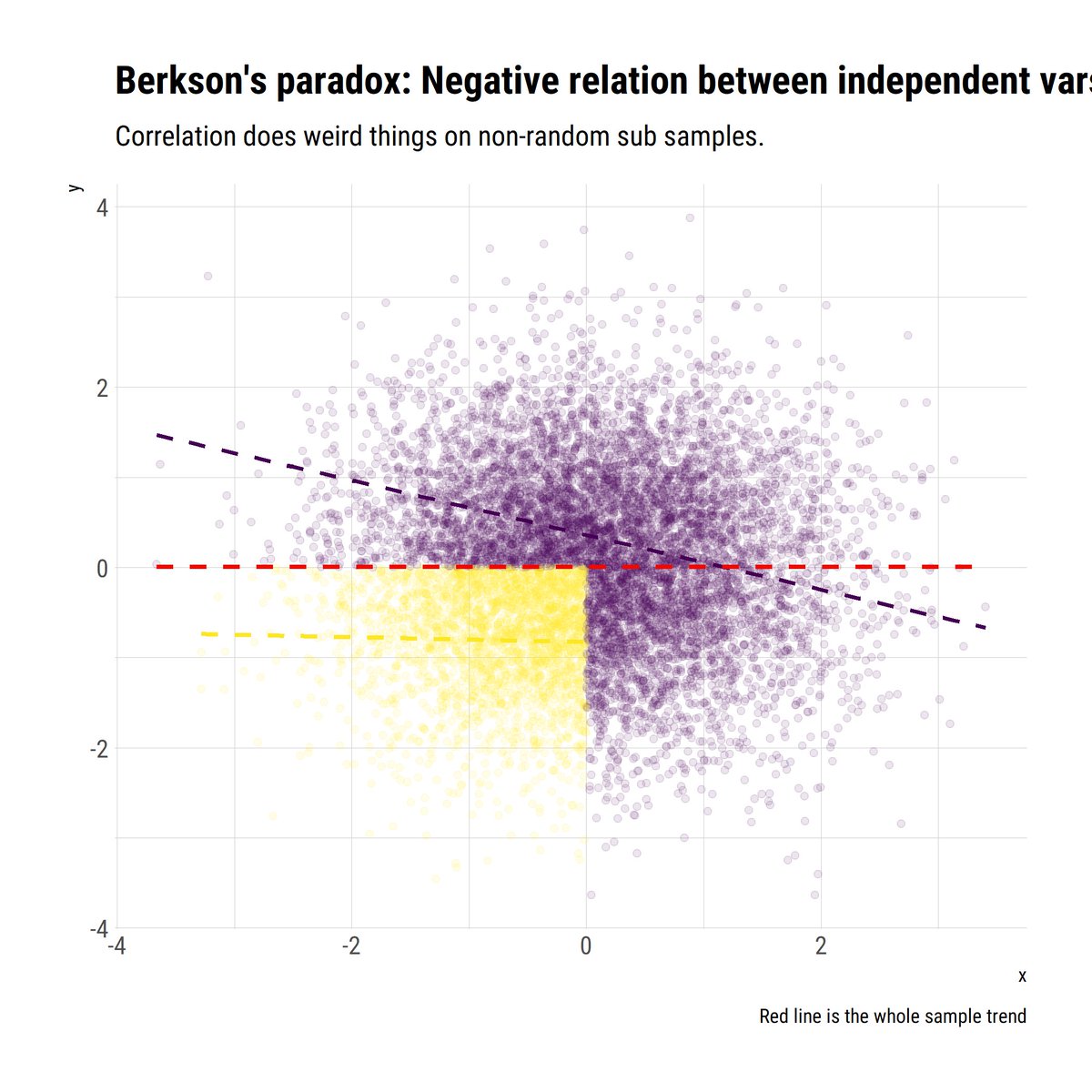
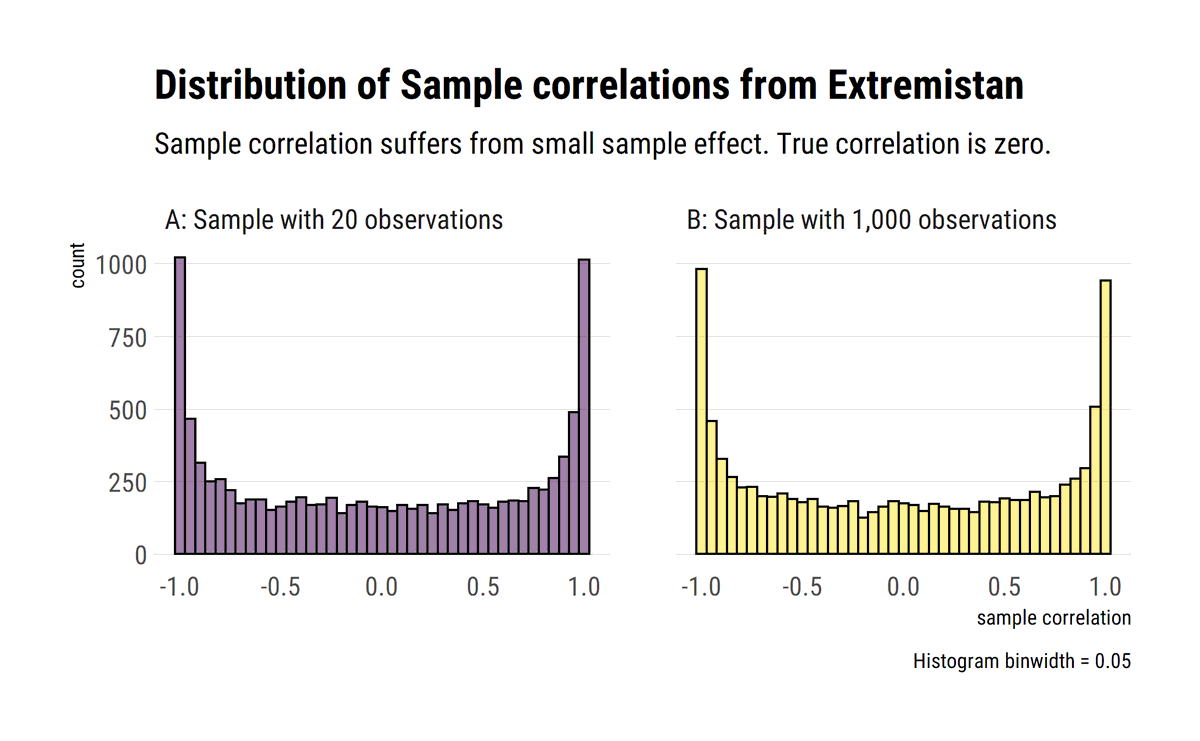
Monte-Carlo experiment:
david-salazar.github.io/2020/05/26/r-s…
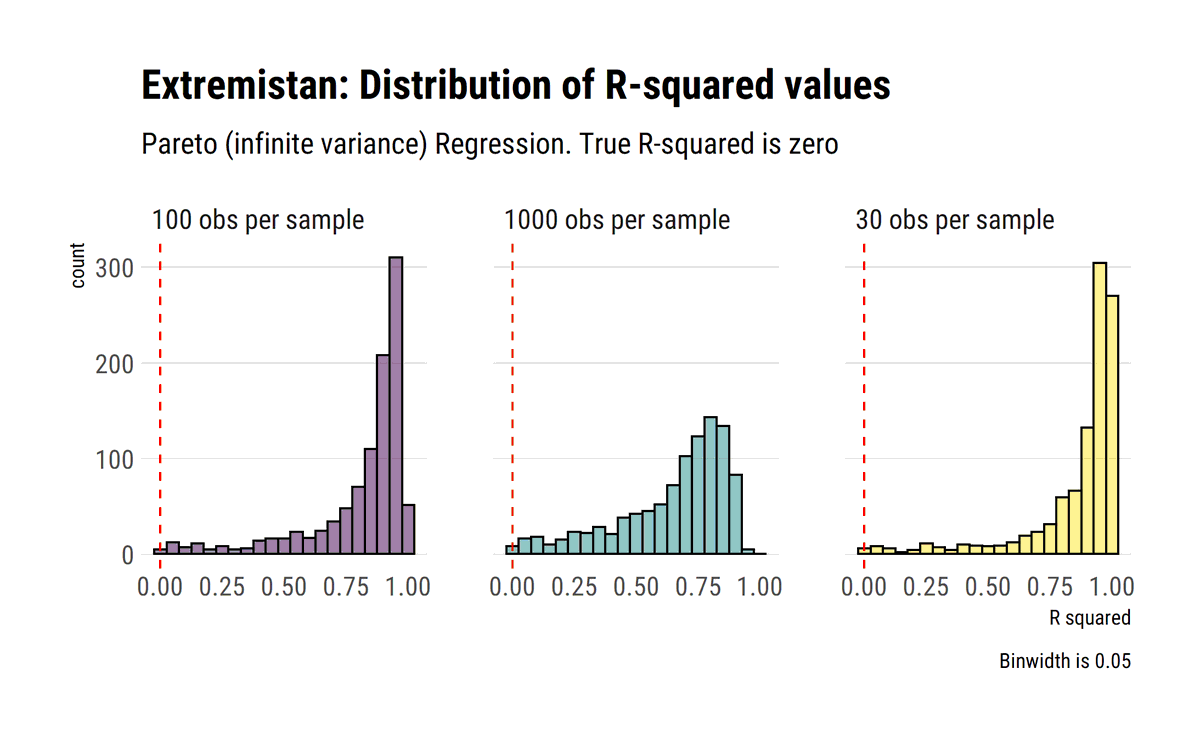
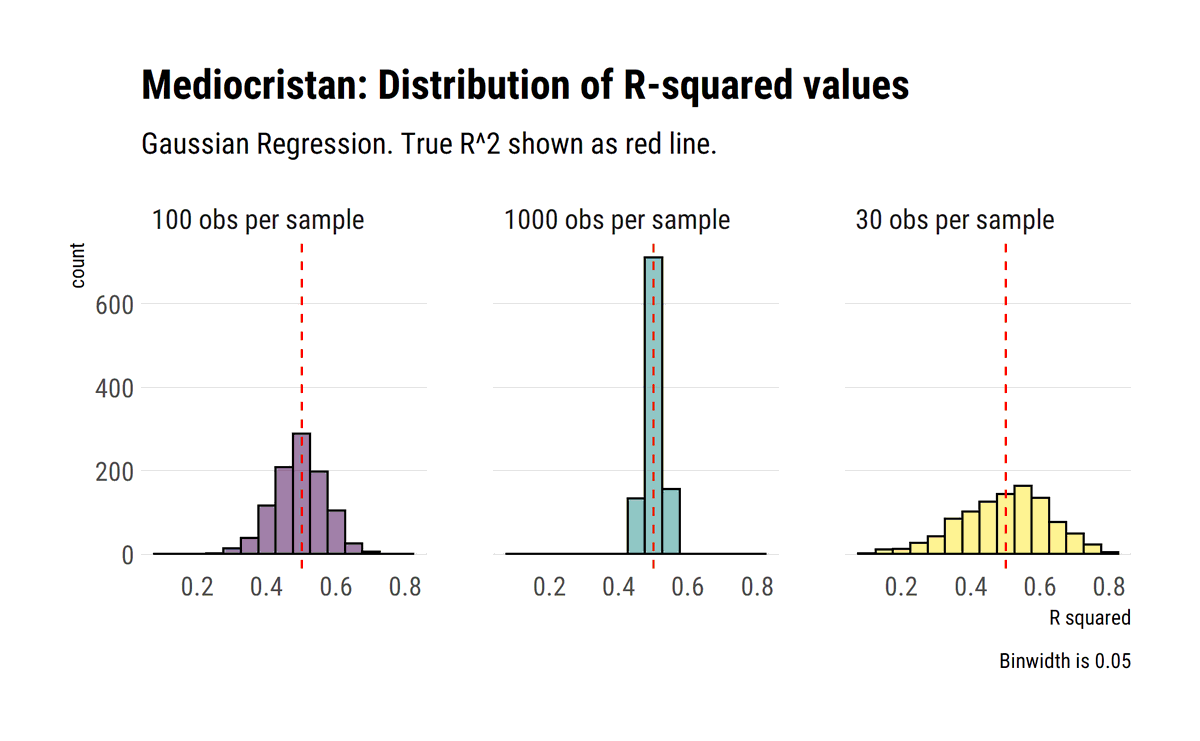
Blogpost with Monte-Carlo experiments:
david-salazar.github.io/2020/05/30/cen…
Blogpost:
david-salazar.github.io/2020/06/02/lln…
arxiv.org/ftp/arxiv/pape…
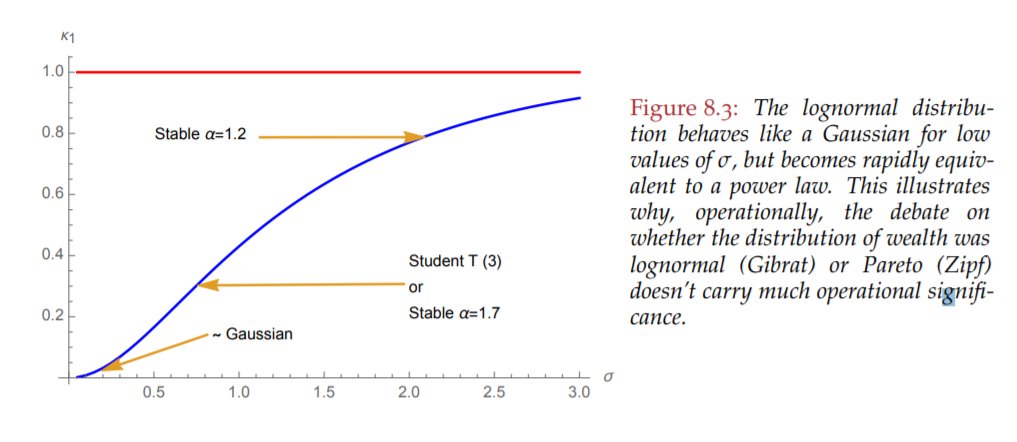
Blogpost:
david-salazar.github.io/2020/06/10/fis…
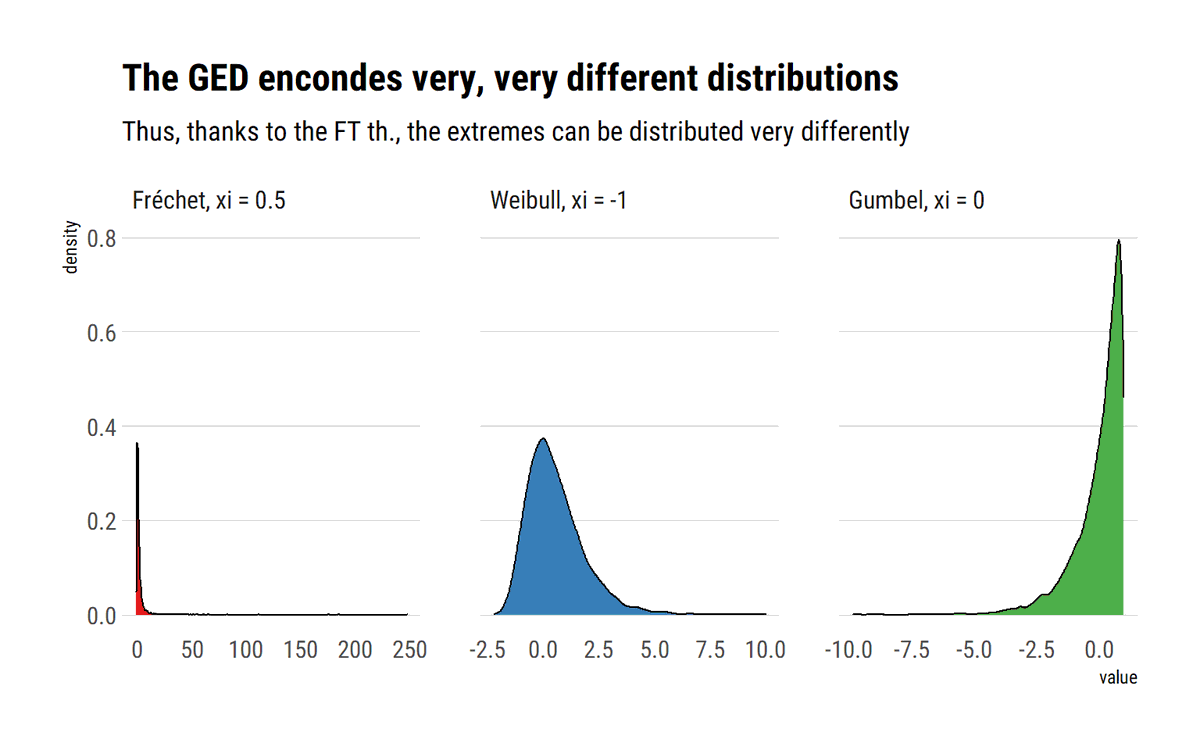
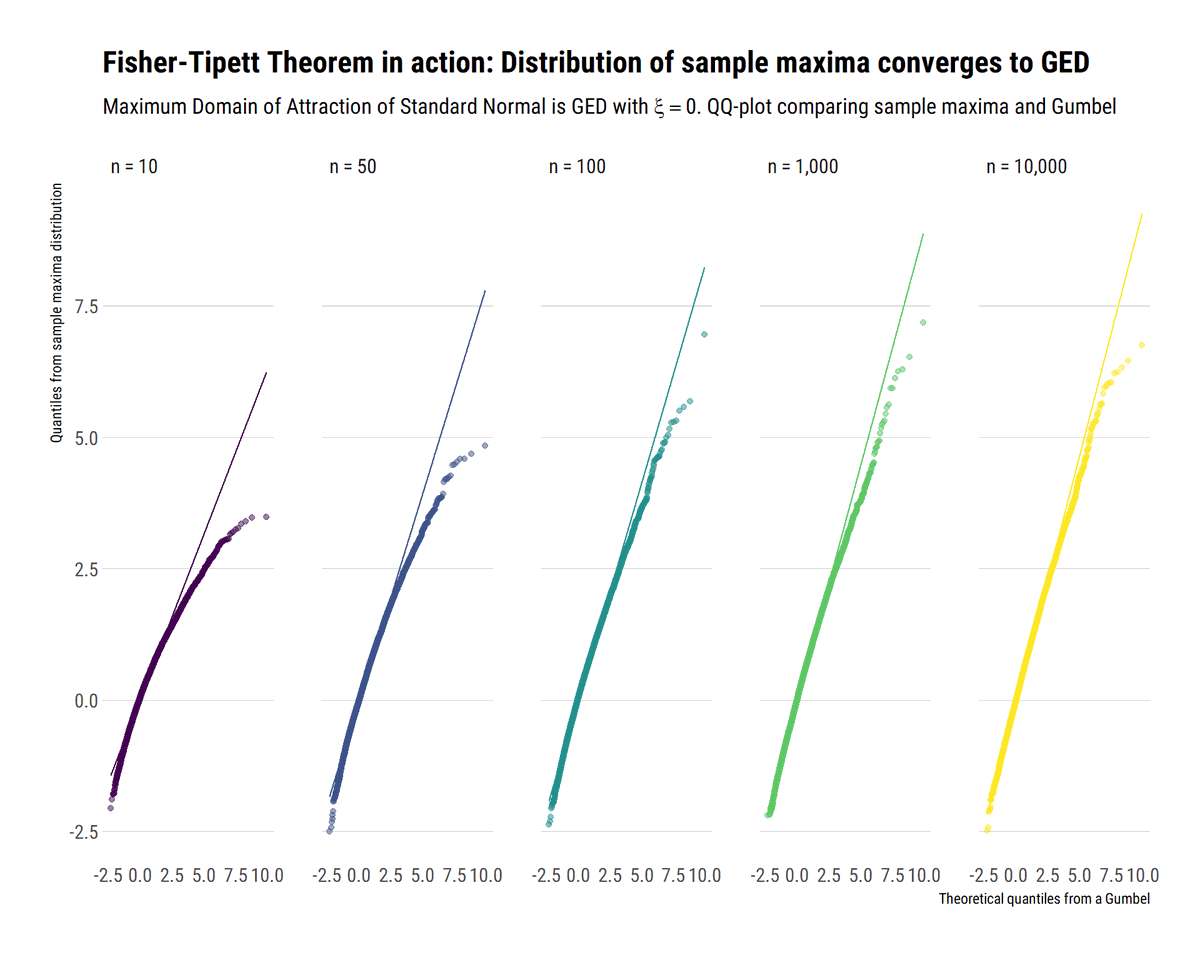
david-salazar.github.io/2020/06/11/how…
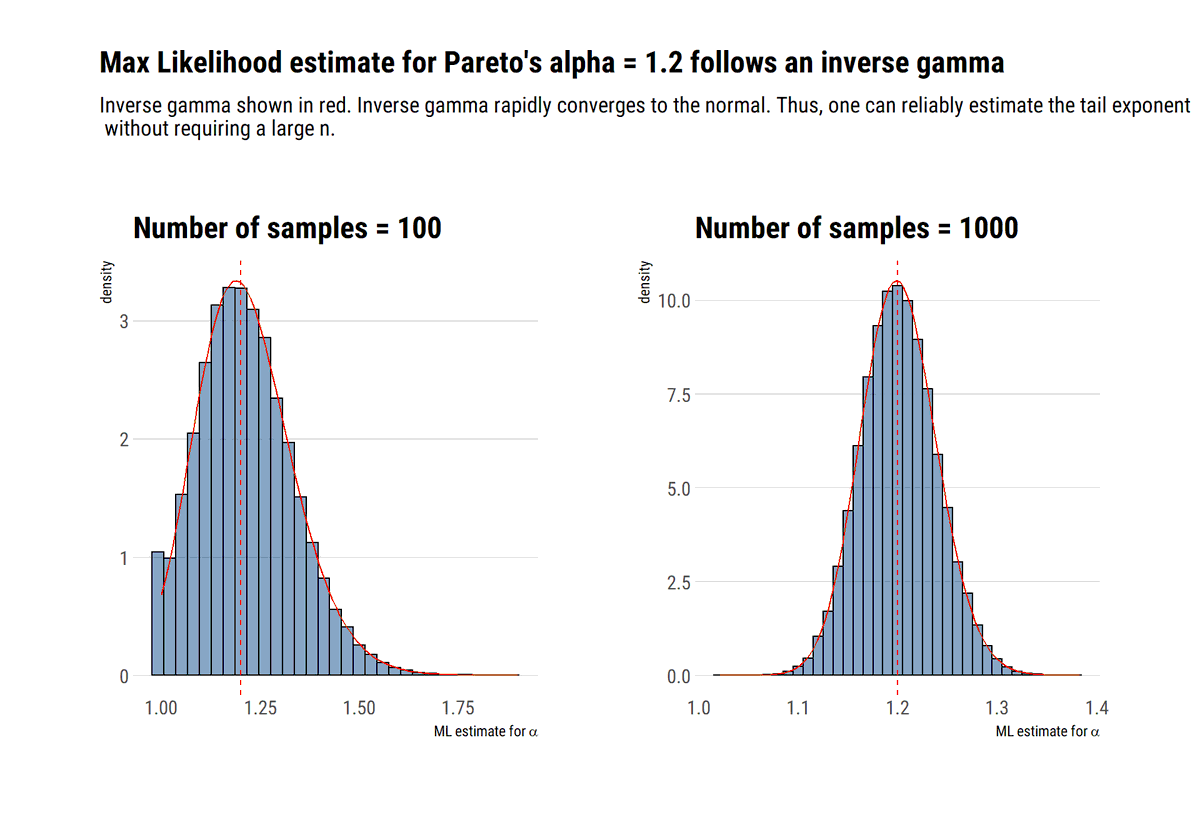
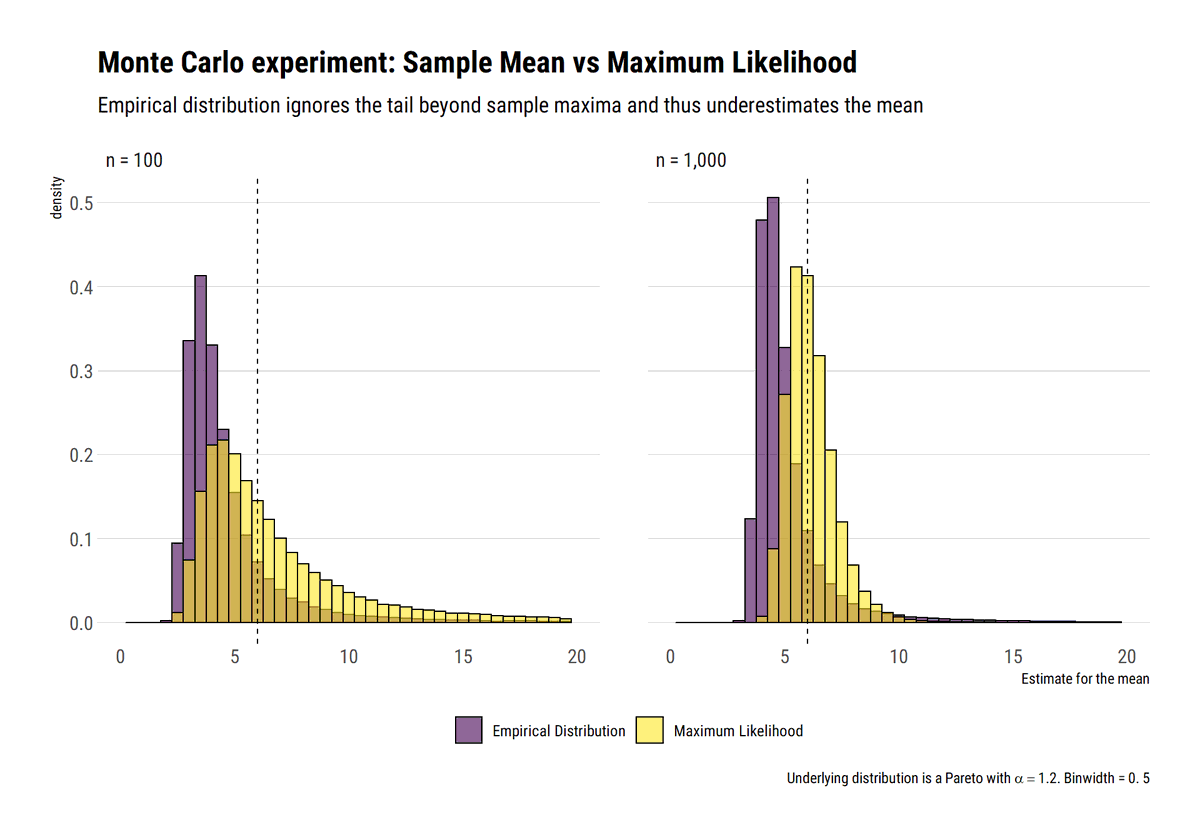
david-salazar.github.io/2020/06/14/whe…
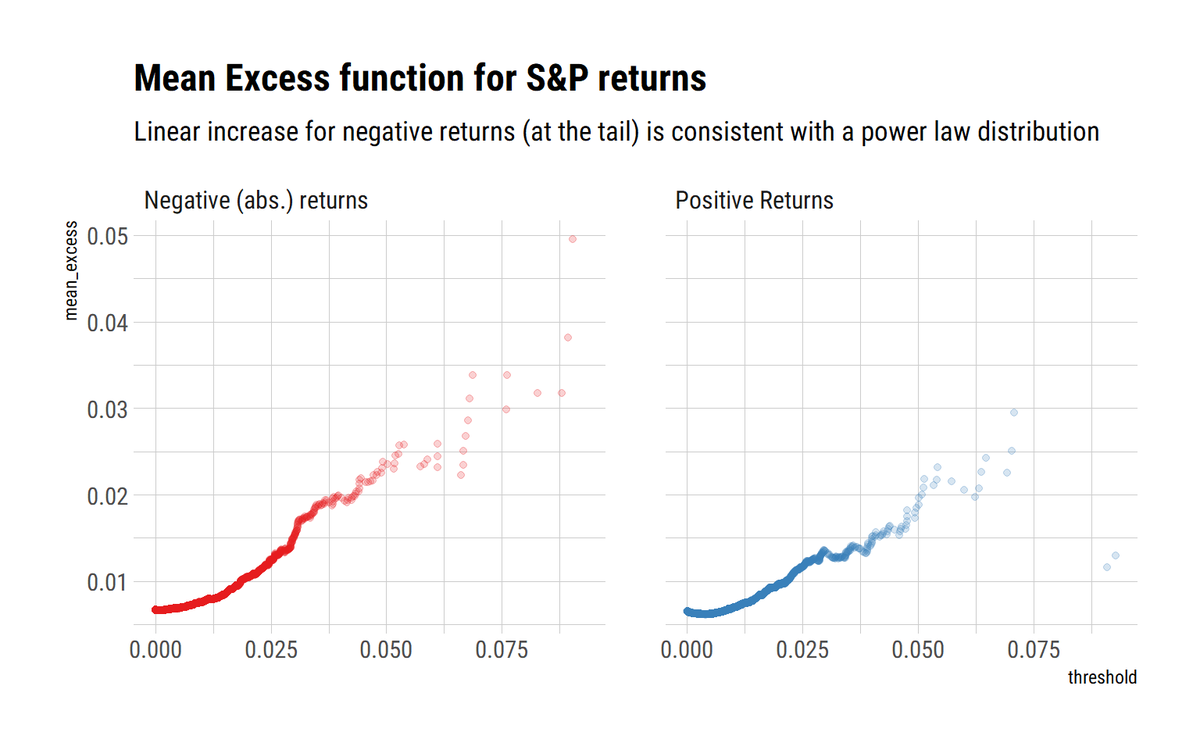
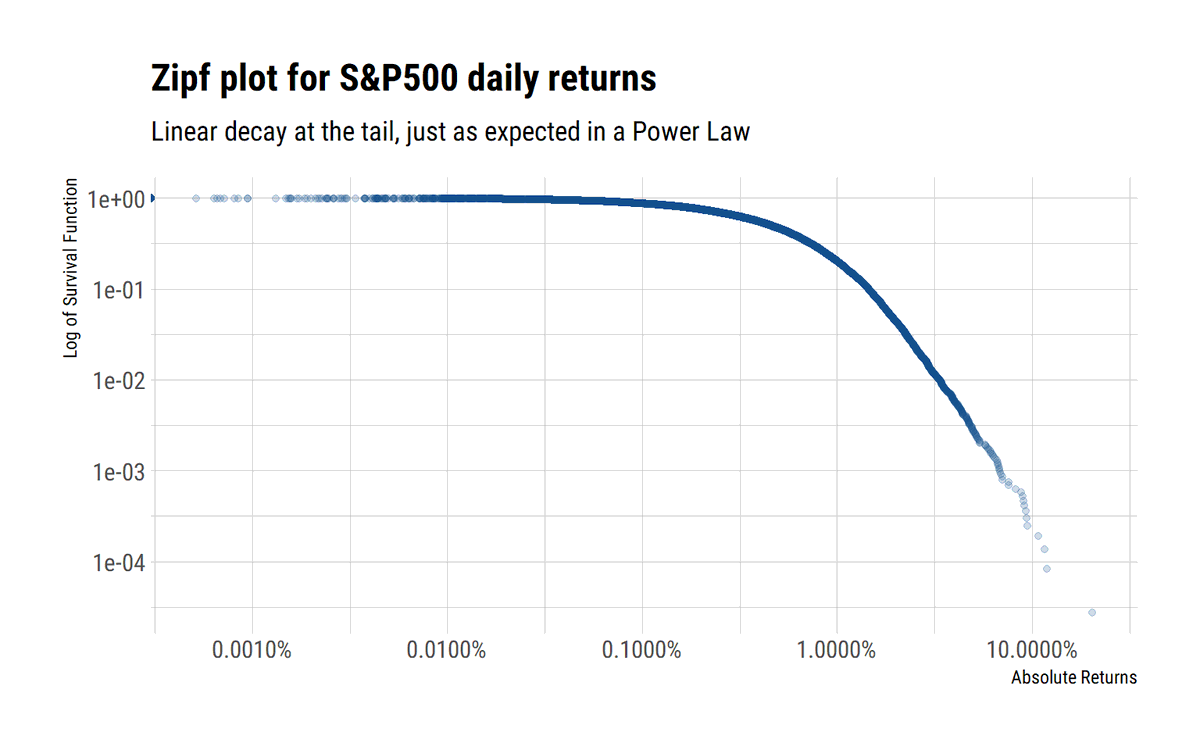
A single point rejects this possibility.
david-salazar.github.io/2020/06/17/ext…
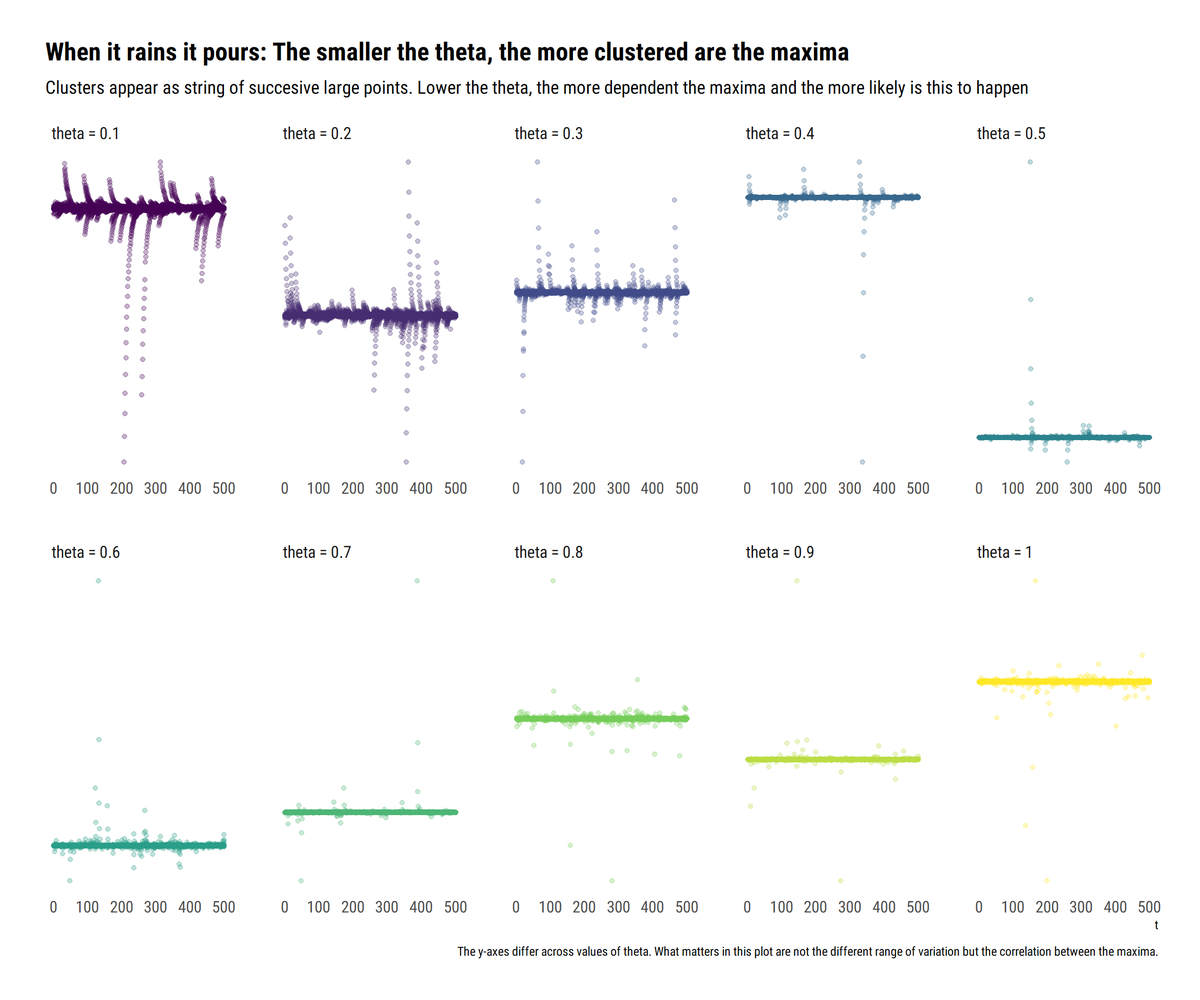
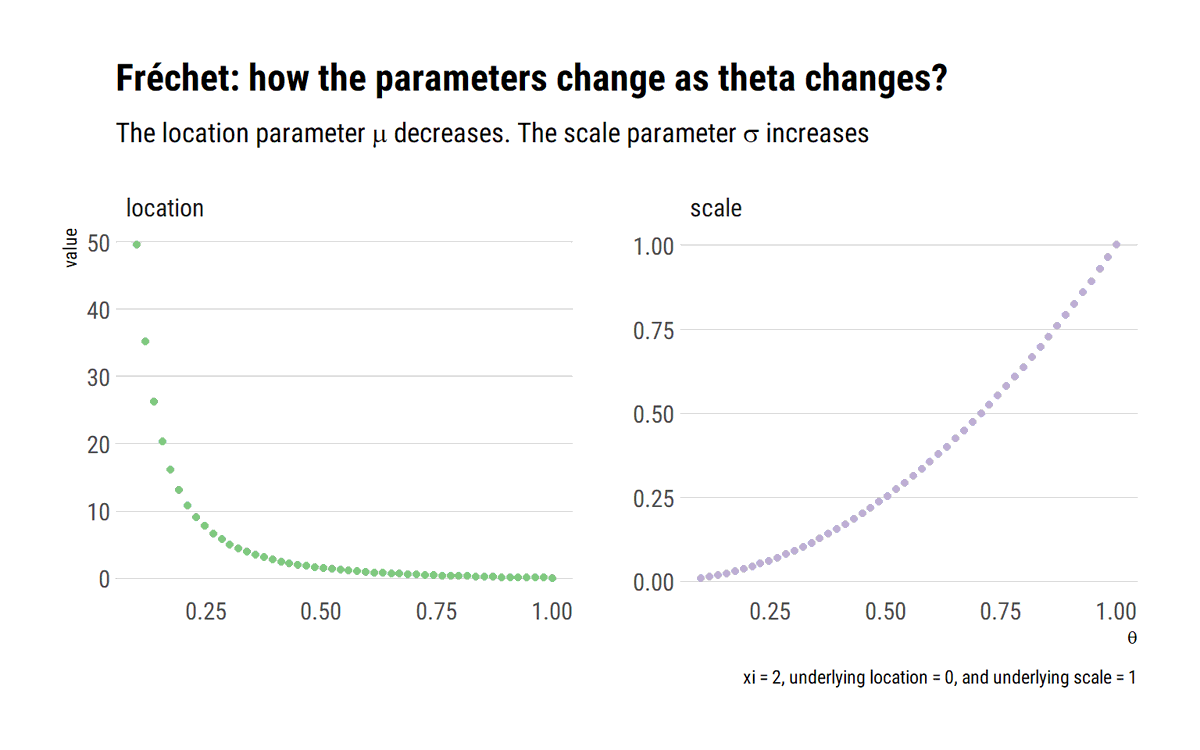
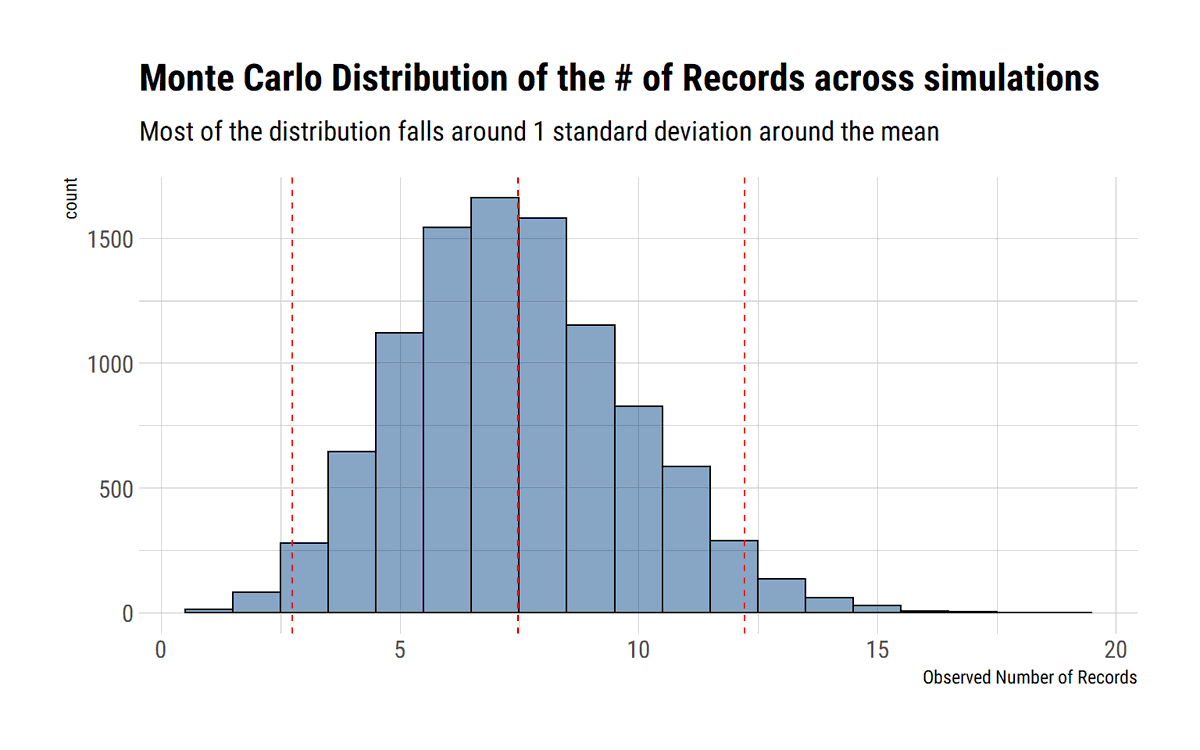
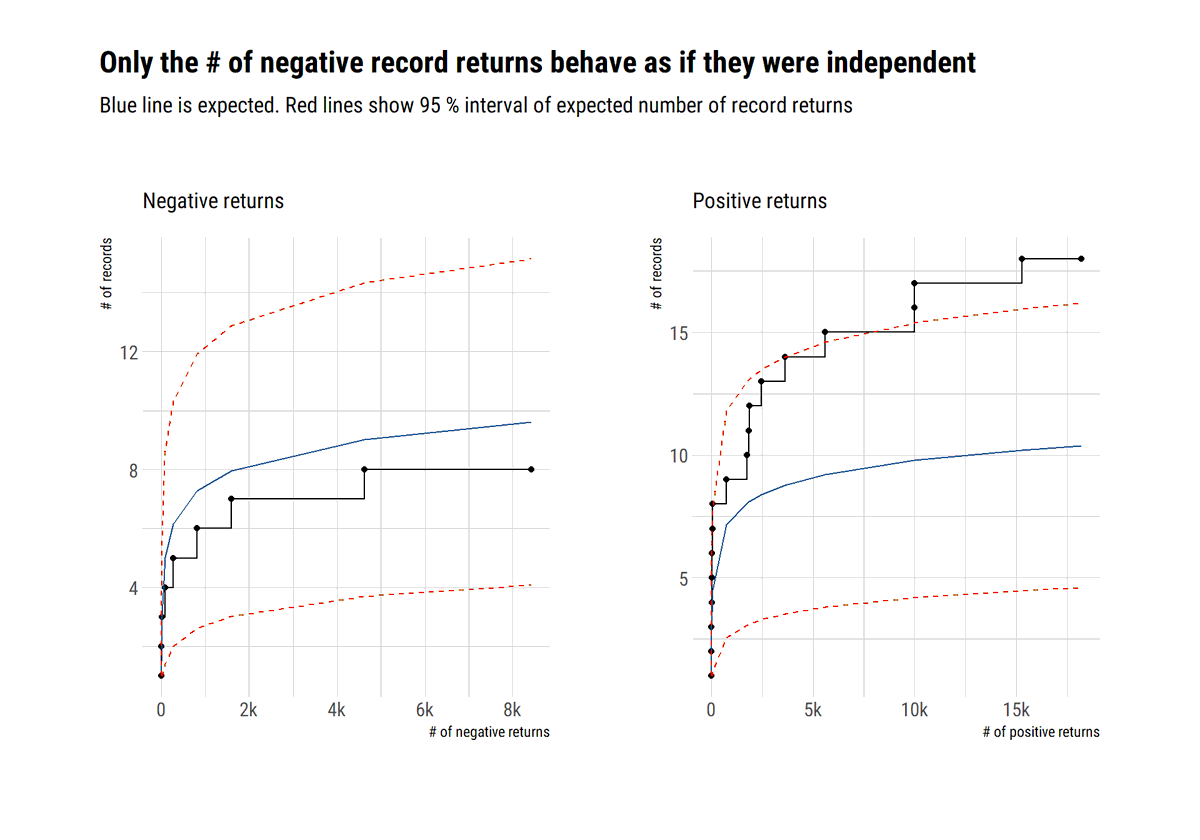
@nntaleb shows that SP500 negative returns can be considered independent
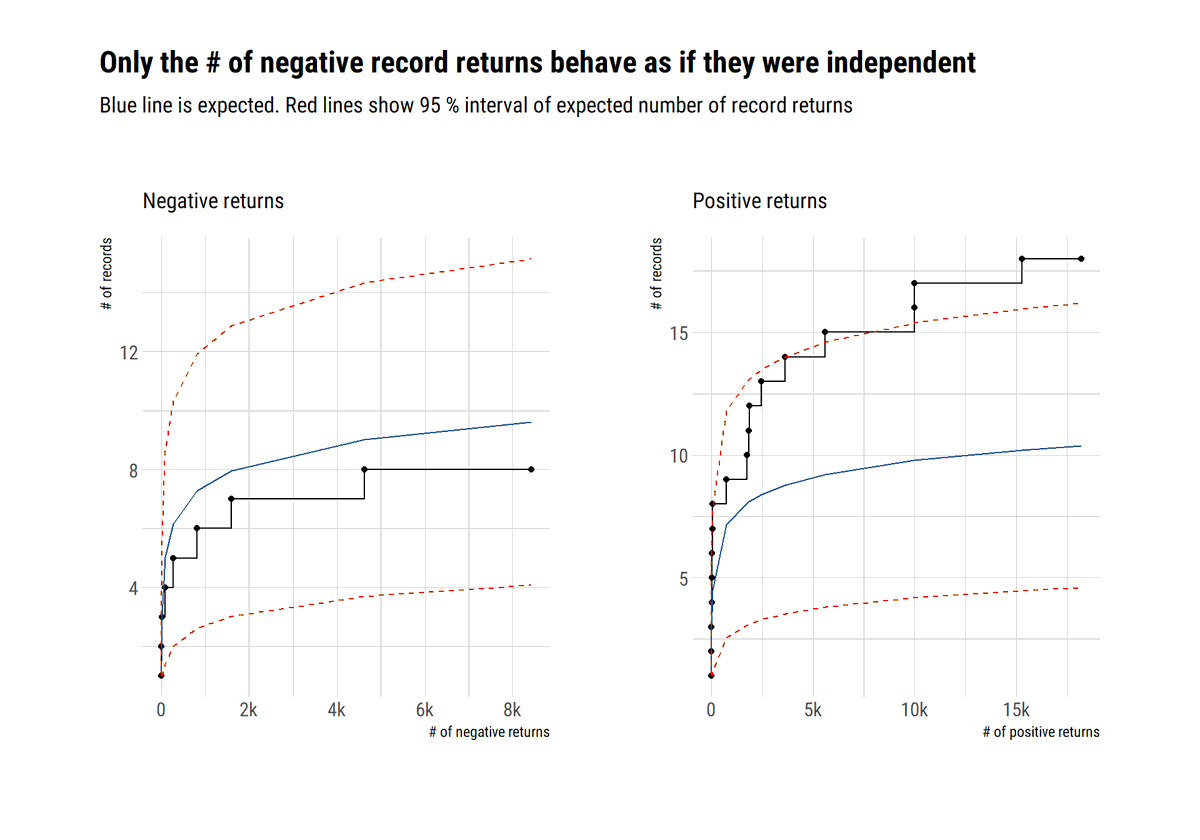
david-salazar.github.io/2020/06/24/pro…
david-salazar.github.io/2020/06/26/gin…
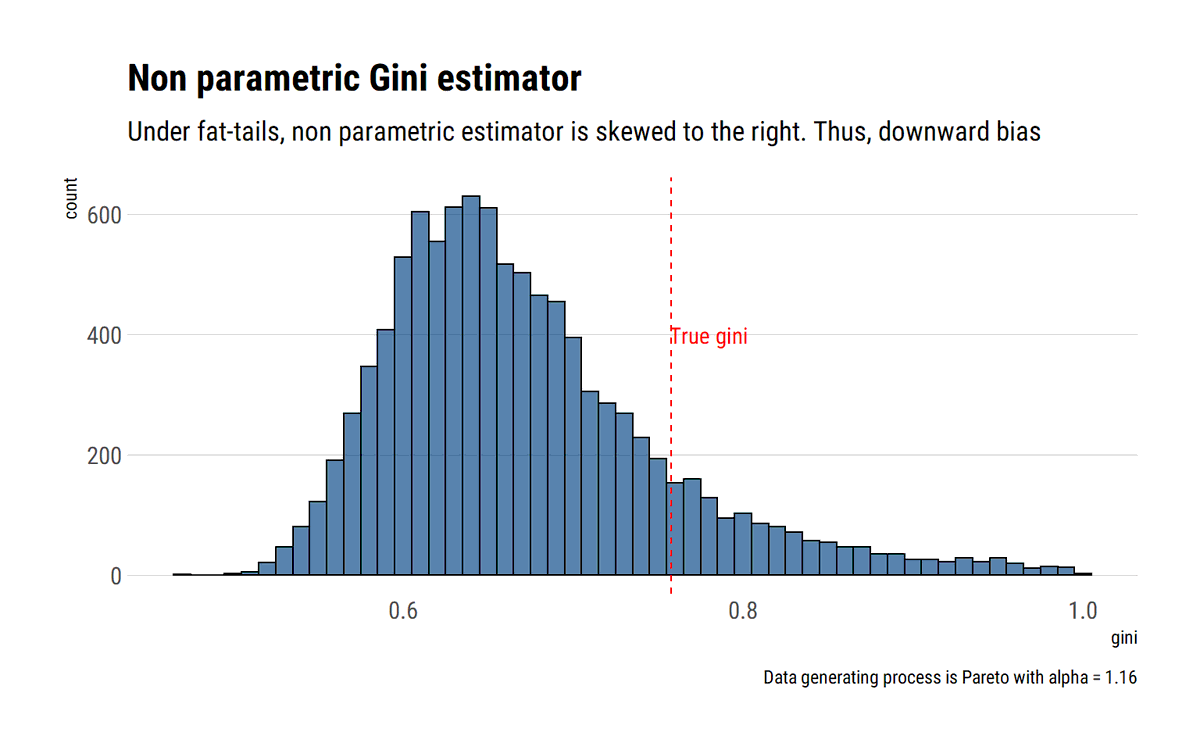
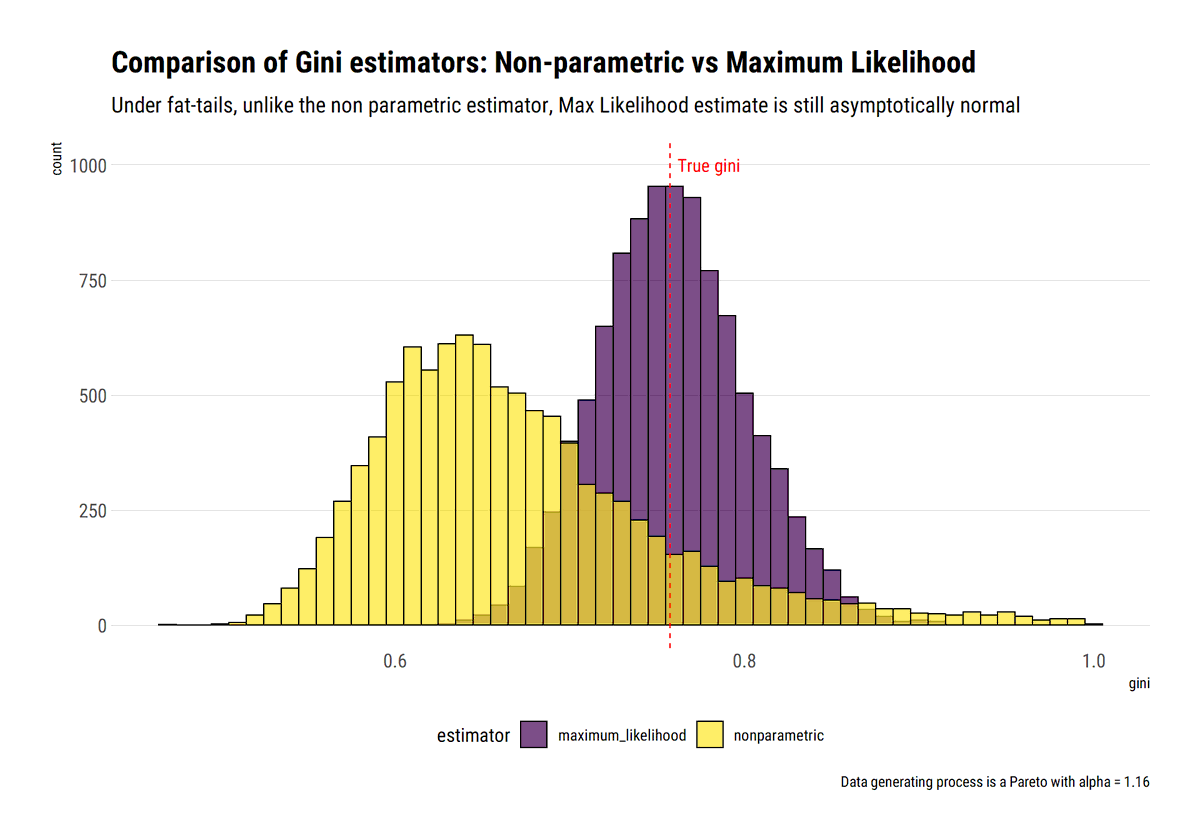
david-salazar.github.io/2020/07/05/tai…
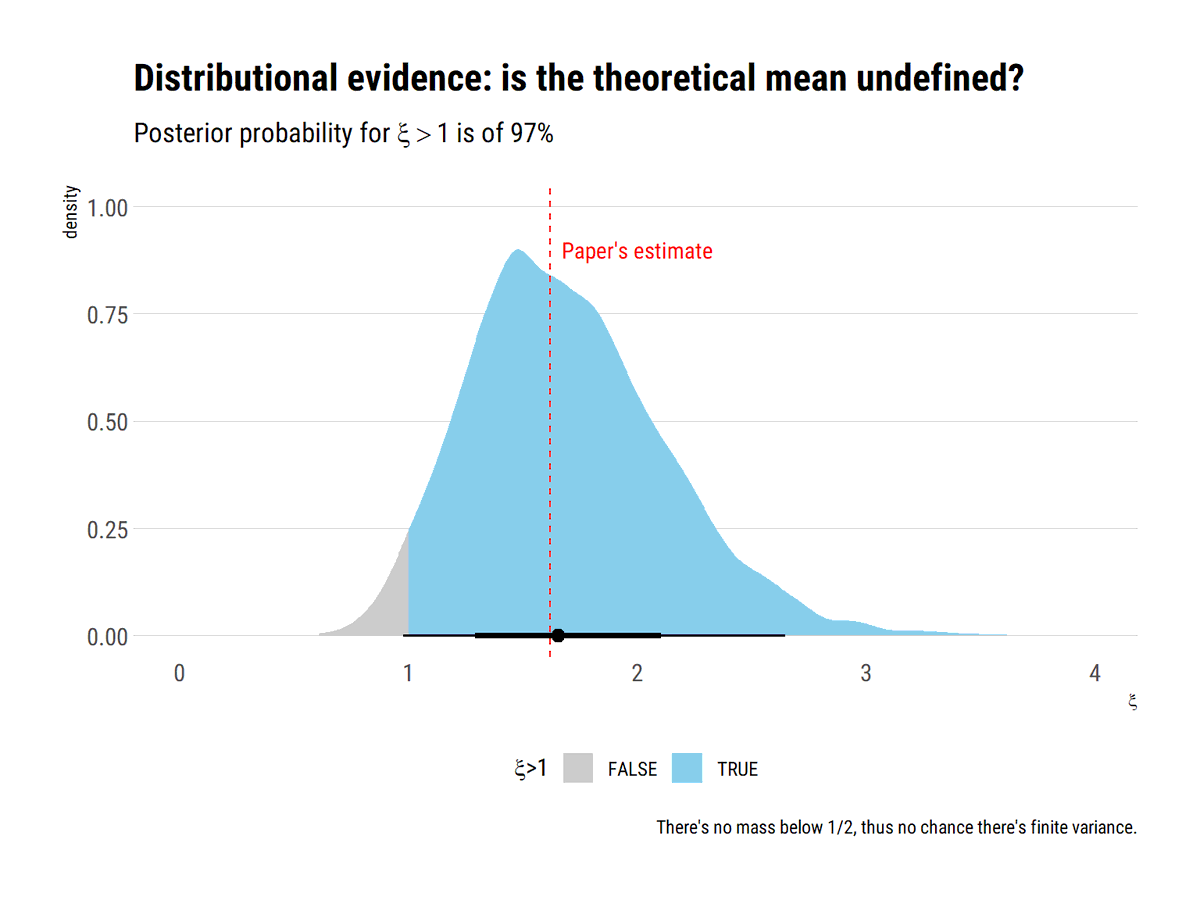
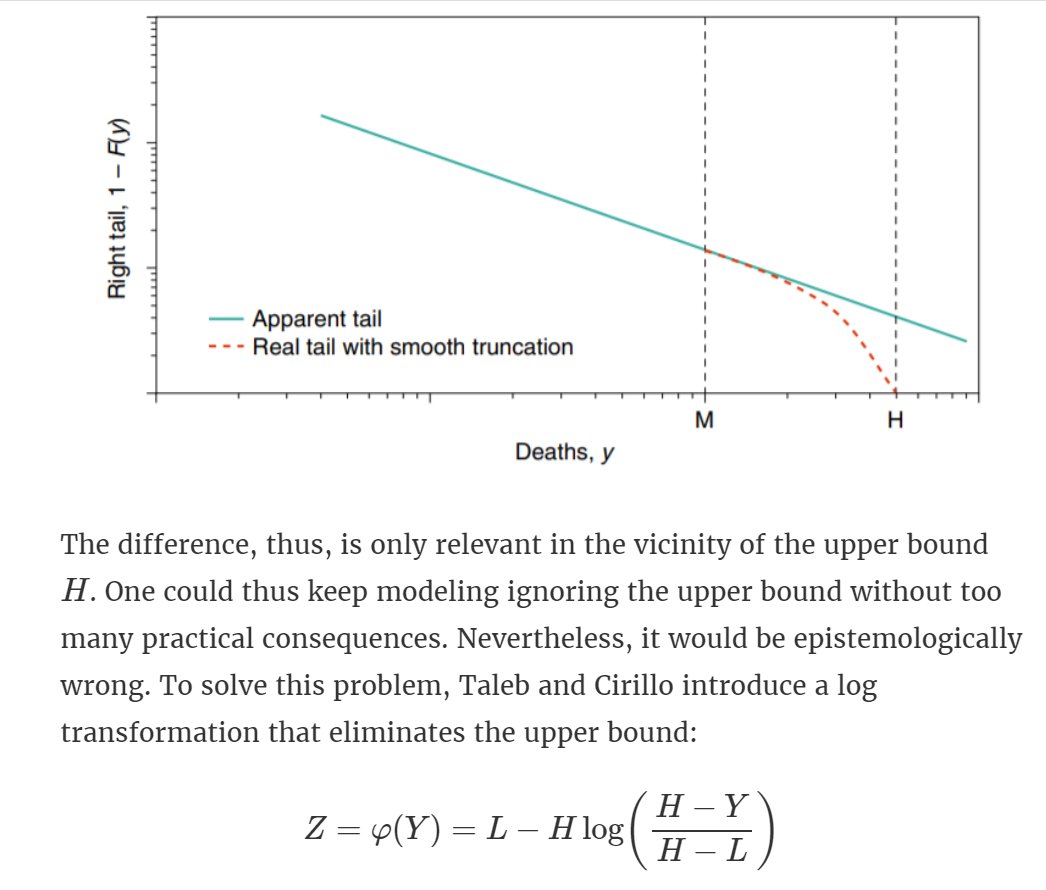
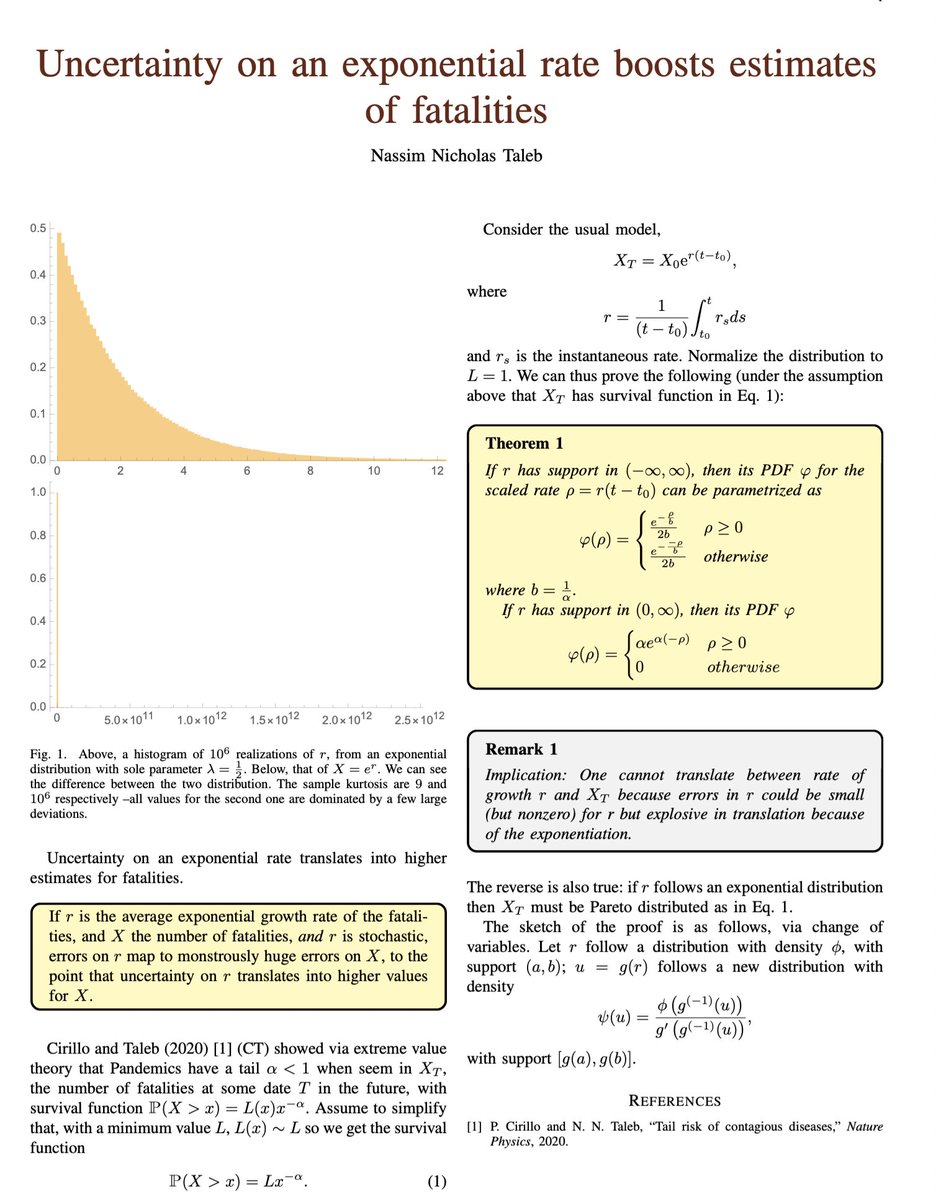