Let’s have a look at the Lancet’s!
TLTR: again, the surprising stuff is in the appendix.
This is huge, unprecedented. And therefore it must be SUPER reliable, right?
Well, not everyone agrees.
Steven Phillips MD doesn't think that bigger is better.
catalogofbias.org/biases/confoun…
1. You’re missing the chance to see it in action when it could work,
2. You’re observing that it does not work,
3. You gave it to more severely ill patients.
In order to evaluate a possible bias, the first point of interest is the inclusion criteria. Or should we say "exclusion" here.
In most studies of this kind, the inclusion criteria is usually “at least 48h of treatment”.
And precisely, it’s a known fact that hospitalists tend to treat COVID patients with HCQ only when they reach a certain level of illness.
ecowatch.com/coronavirus-sy…

ncbi.nlm.nih.gov/pmc/articles/P…
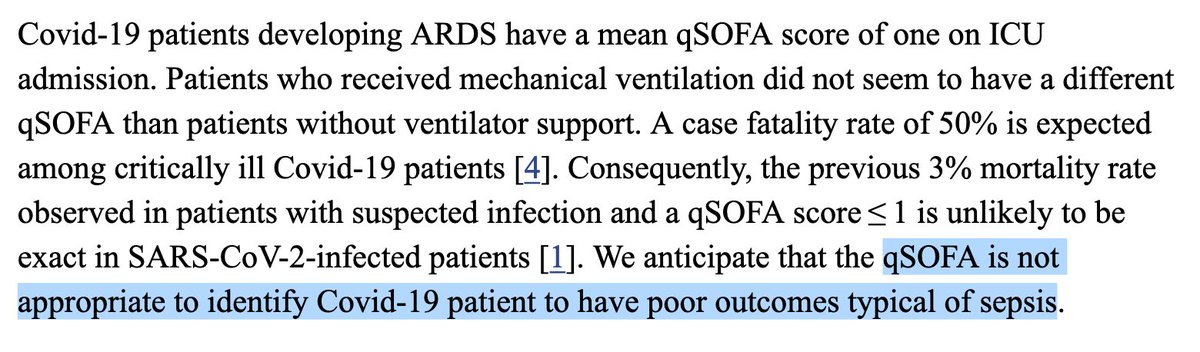
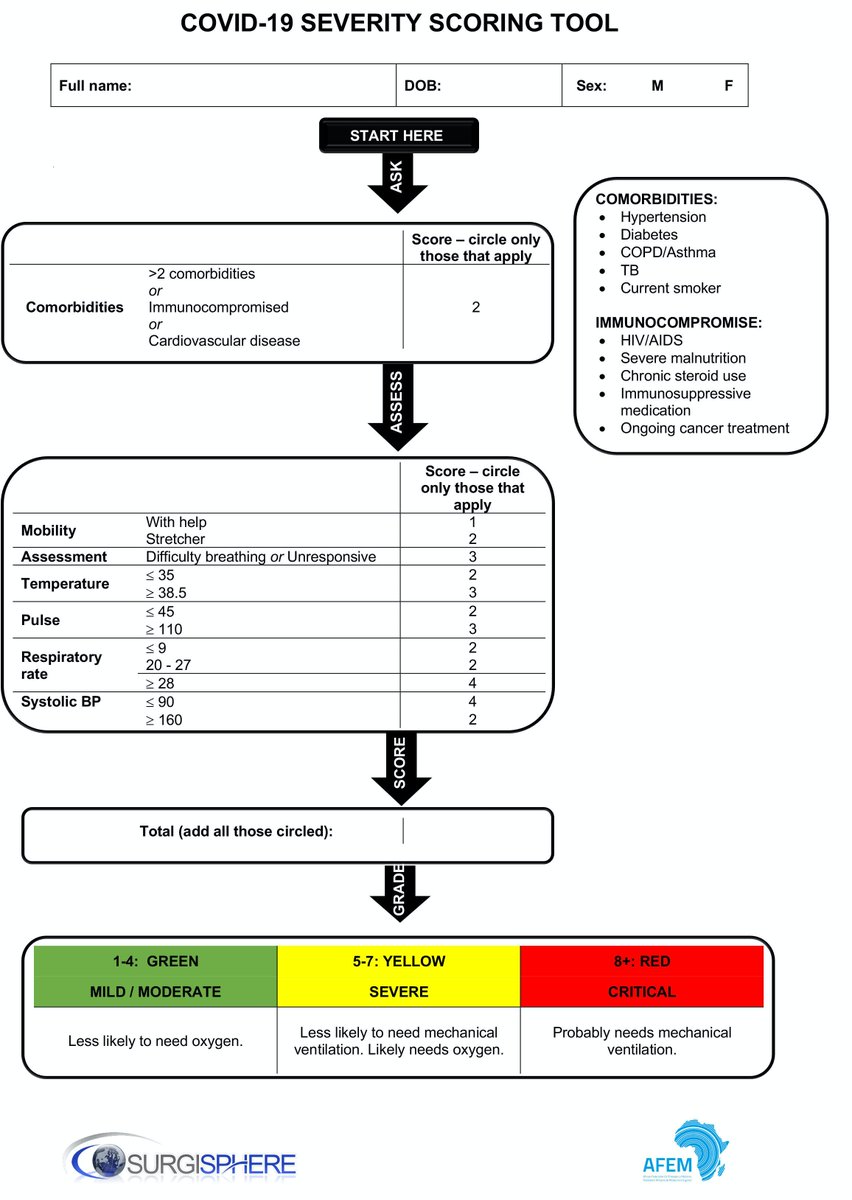
businesswire.com/news/home/2020…
gking.harvard.edu/publications/w…
🤓

There is none.
onlinelibrary.wiley.com/doi/full/10.11…
We’re in front of unprecedented, striking results which were obtained using a touchy statistical tool run on a gigantic, sparse data set exhibiting scarce, unreliable and vague, baseline severity indicators in the context of a known indication bias.
You wouldn’t mind sharing it, would you?
Can you please provide us with it, along with the R code that was used to do the computations?
Thanks. 🙏