A trained language model generates text.
We can optionally pass it some text as input, which influences its output.
The output is generated from what the model "learned" during its training period where it scanned vast amounts of text.
1/n
2/n
The model's prediction will be wrong. We calculate the error in its prediction and update the model so next time it makes a better prediction.
Repeat millions of times
4/n
GPT3 actually generates output one token at a time (let's assume a token is a word for now).
5/n
In my Intro to AI on YouTube, I showed a simple ML model with one parameter. A good start to unpack this 175B monstrosity
8/n
GPT3 is 2048 tokens wide. That is its "context window". That means it has 2048 tracks along which tokens are processed.
9/n
High-level steps:
1- Convert the word in a vector (list of numbers) representing the word: jalammar.github.io/illustrated-wo…
2- Compute prediction
3- Convert resulting vector to word
10/n
See all these layers? This is the "depth" in "deep learning".
Each of these layers has its own 1.8B parameter to make its calculations.
12/n
The difference with GPT3 is the alternating dense and sparse self-attention layers (see arxiv.org/pdf/1904.10509…).
13/n
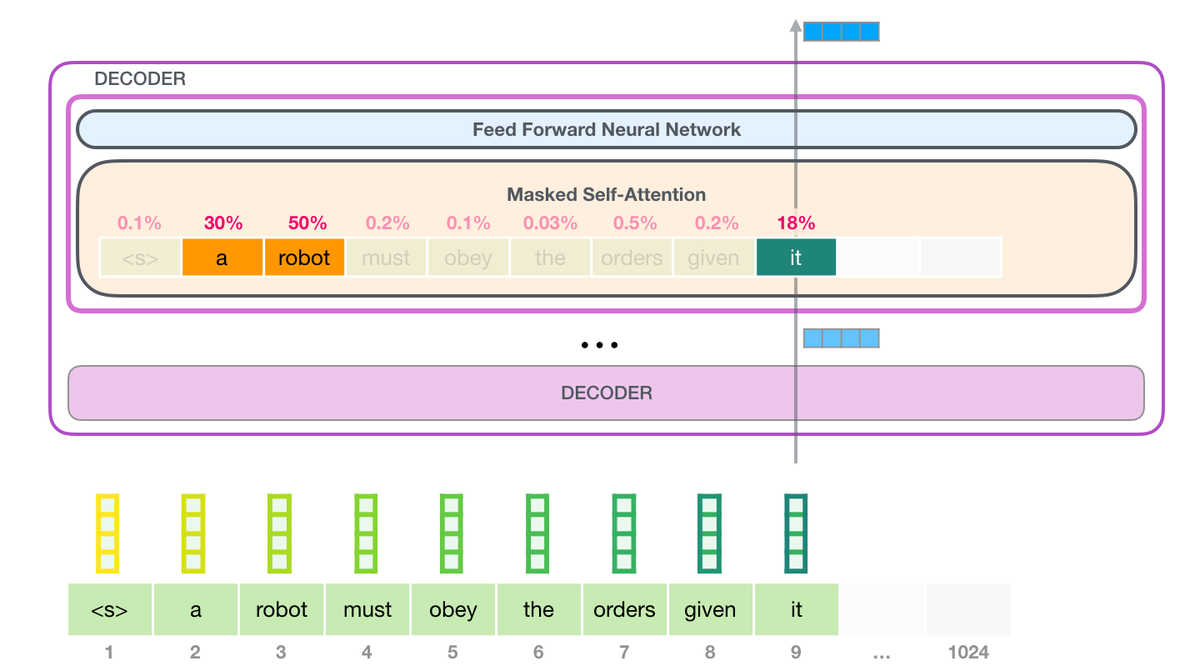
I will keep updating it as I create more visuals.
14/n