1) We have large datasets. 2) We use appropriate models.
KHDRBS2 previously reported as under selection for domestication (nature.com/articles/ng.37…). Our results clarify that this locus is likely under balancing selection for local adaptation.
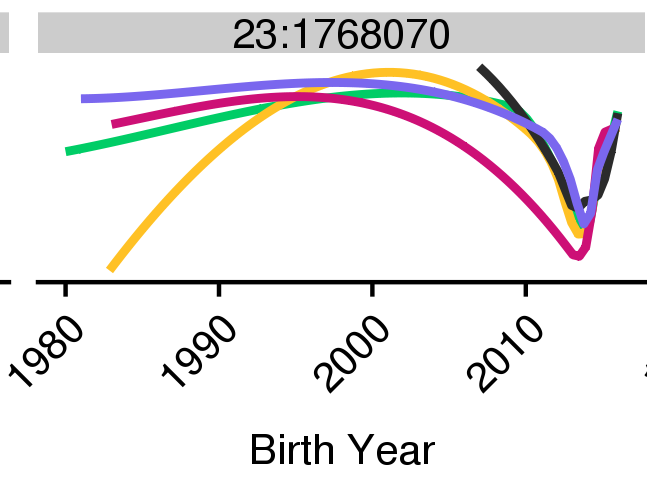
biorxiv.org/content/10.110…
Congrats to @TroyNRowan for great work.
Thanks to all of our collaborators and to @USDA_NIFA for funding.
Thanks to @RedAngusAmerica @ASASimmental & @GelbviehNews for sharing large datasets!
Check out their #rstats package AlphaSimR!