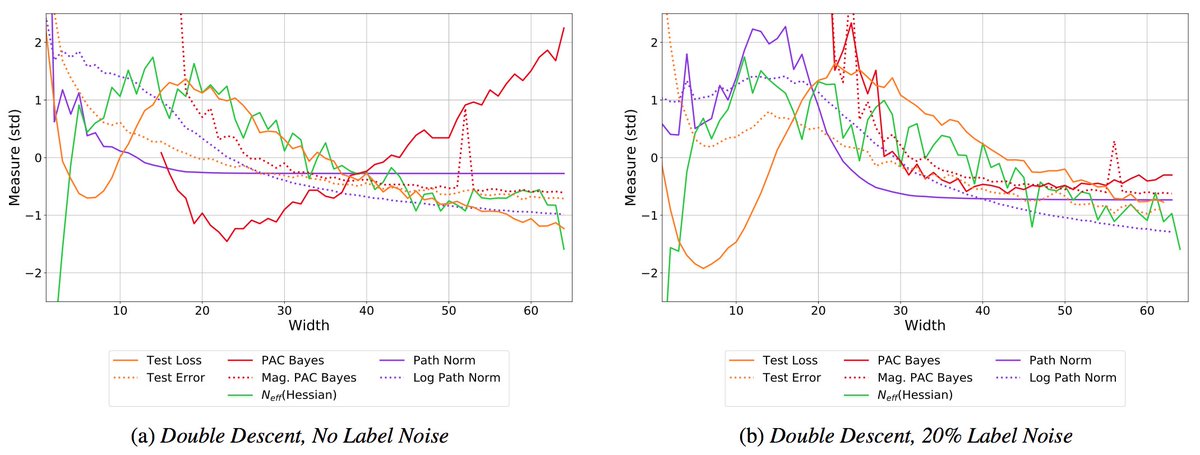
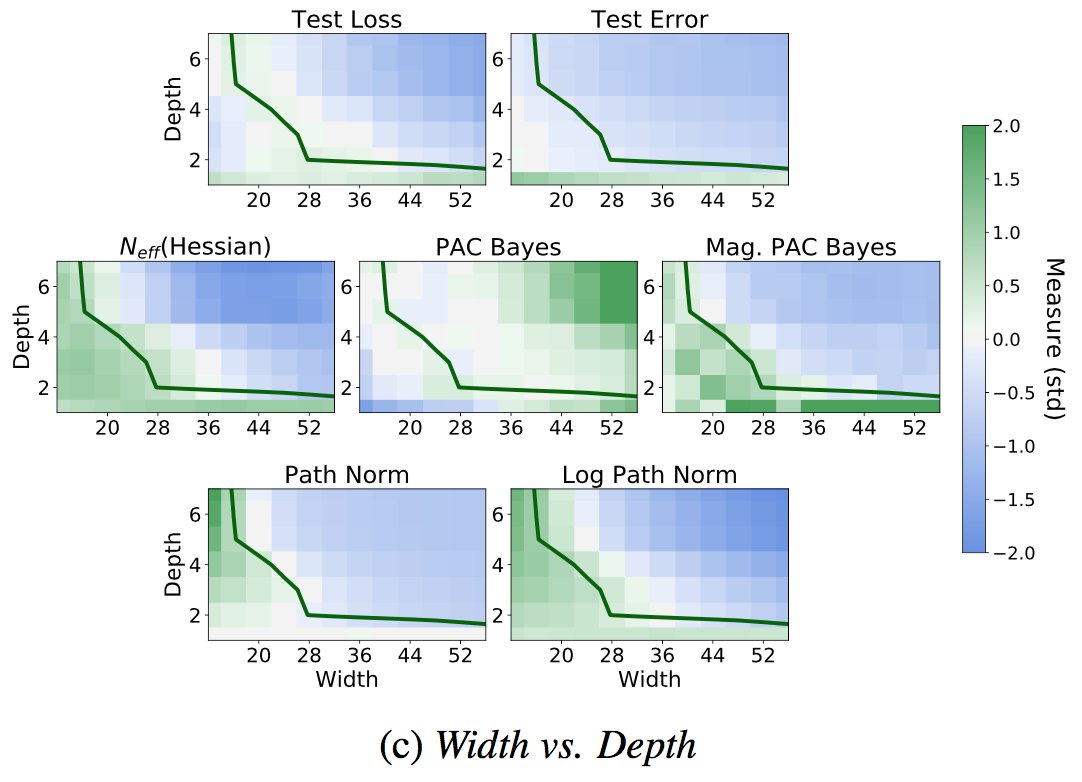
3/16
6/16
8/16
9/16
15/16
Work with @g_benton_ and Wesley Maddox. 16/16
Keep Current with Andrew Gordon Wilson
This Thread may be Removed Anytime!
Twitter may remove this content at anytime, convert it as a PDF, save and print for later use!
1) Follow Thread Reader App on Twitter so you can easily mention us!
2) Go to a Twitter thread (series of Tweets by the same owner) and mention us with a keyword "unroll"
@threadreaderapp unroll
You can practice here first or read more on our help page!