@arxiv manuscript: arxiv.org/abs/2005.04704
Code: github.com/aapeterson/pow…
1/n
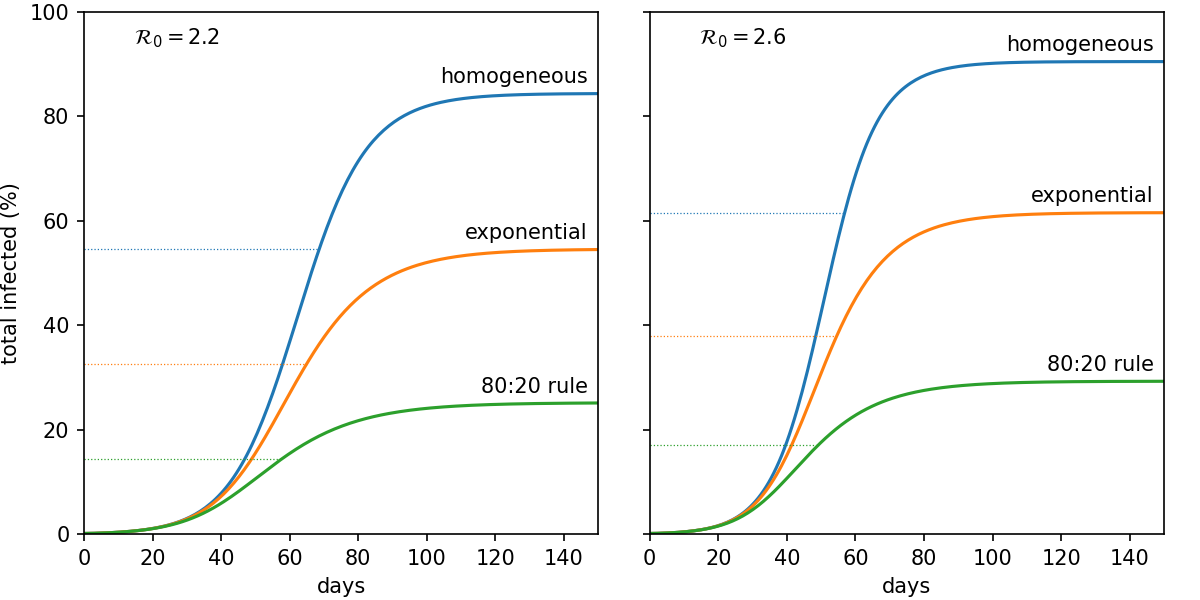
@TheAtlantic:
theatlantic.com/health/archive…
Gomes et al.
doi.org/10.1101/2020.0…
Britton et al.
science.sciencemag.org/content/early/…
This means that not just the *number* of susceptible people decreases as the disease progresses, but also the *average susceptibility* of those uninfected.
Christopher Rose, Andrew J. Medford, C. Franklin Goldsmith, Tejs Vegge, myself, Andrew A. Peterson
Manuscript: arxiv.org/abs/2005.04704
Code: github.com/aapeterson/pow…
Feedback welcome.