In our recent paper arxiv.org/abs/1912.02757 with @stanislavfort & Huiyi Hu, we investigate this by using insights from recent work on loss landscape of neural nets.
More below:
We measure the similarity of function (both in weight space and function space) to test this hypothesis.
Given a reference solution, we plot diversity vs accuracy to measure how different methods trade-off diversity vs accuracy.
@stanislavfort will also be giving a contributed talk about our work on Dec 13 (Friday) 9-915 AM and presenting a poster at the Bayesian deep learning workshop (bayesiandeeplearning.org) at #NeurIPS2019
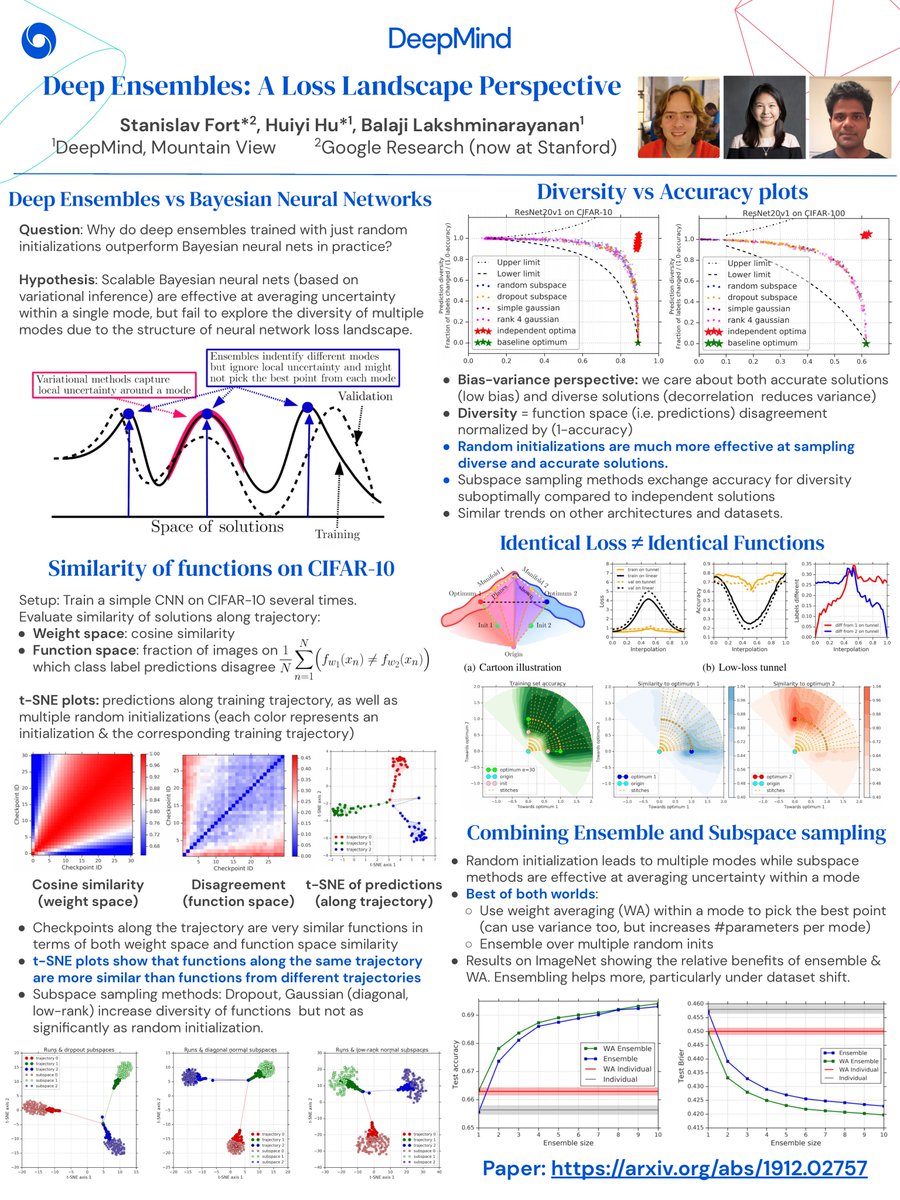