✓3 non-TCGA datasets (HCC vs CIRR)
✓explanations for parameters & rgCCA choice
✓good at known cancer drivers recovery
✓consistent across cohorts
#SundayMultiOmics 1/n
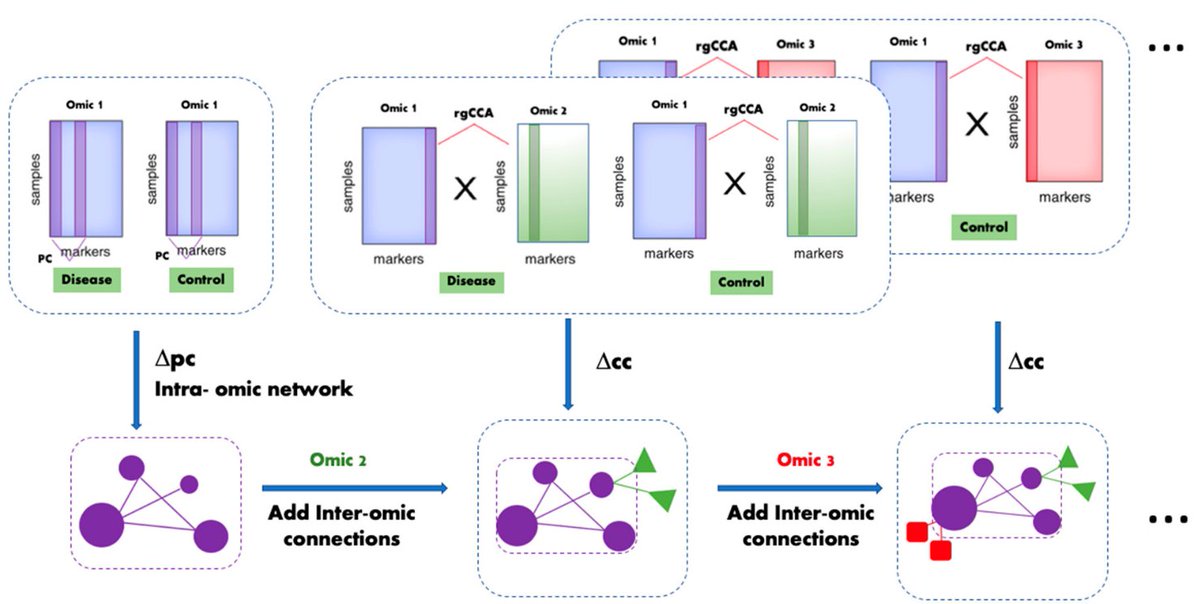
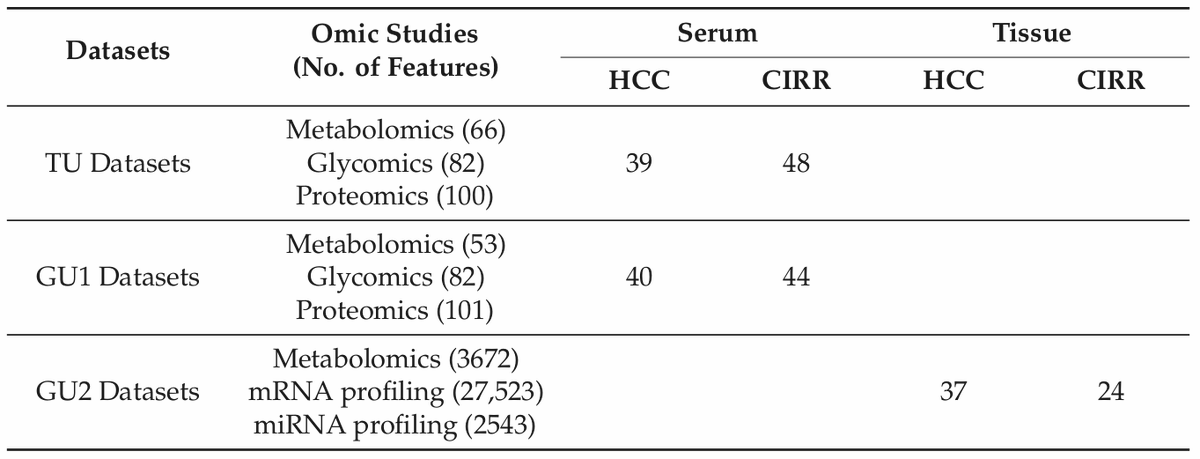
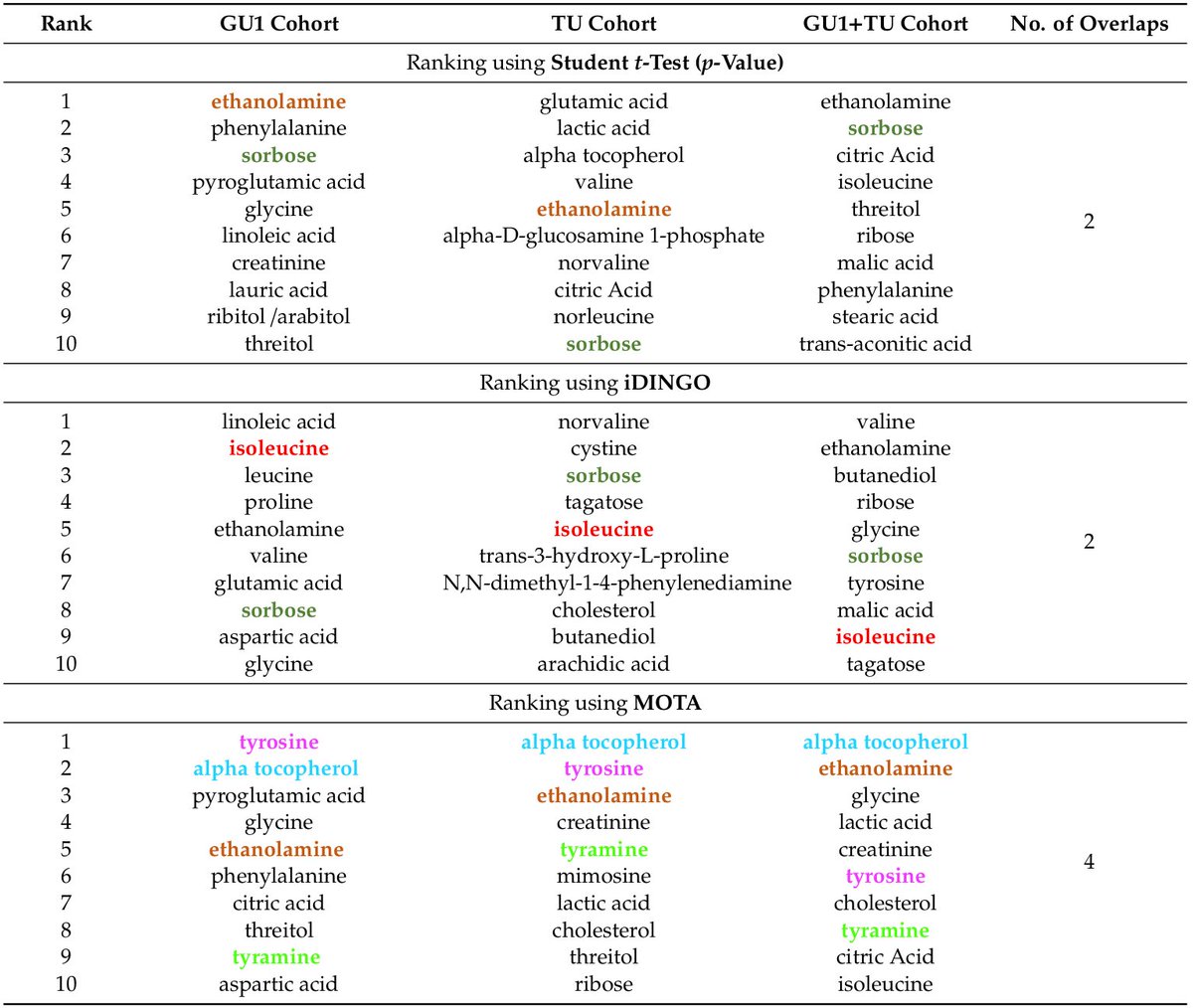
Link: doi.org/10.3390/metabo…
Figures © by authors, reused under CC-BY 4.0
creativecommons.org/licenses/by/4.…
Developed @LombardiCancer @gumedcenter (sorry I could not to find authors on Twitter)