Specifically, we reviewed 50 years of research on *organizational #adaptation*
It's a large literature that uses various labels to refer to the same thing (adaptation, fit, congruence, strategic change)
How did that go?
"how can I be sure you're not biased and projecting your own framework onto the adaptation literature?"
We thus decided to try the #AI route...
at the crossroads of unsupervised machine learning & natural language processing
It's a text-mining tool that clusters similar words as "topics" based on how frequently words co-occur within each text (here, each adaptation research paper)
*brace yourself*
here are the topics
*ta-daaaa*
(You can play with the topic map interactively thanks to this awesome data viz coded by @andrewsarta: sites.google.com/view/andrewsar…)
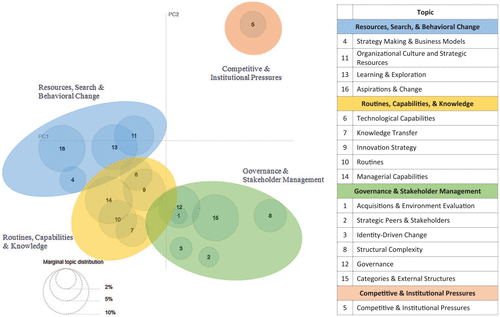
probably because a cluster of studies was published using the same empirical setting
however
the human and machine classifications were *very* similar in our case!
still, human decisions were essential all along (e.g. keyword & parameter choices) and the machine mostly performed the "dumb" tasks
(we were lucky to have an incredibly constructive editor and set of reviewers. It makes all the difference in the world when using a relatively new-ish method)