In this thread I'll experiment to learn more! 👇 #neuralimagen #procjam
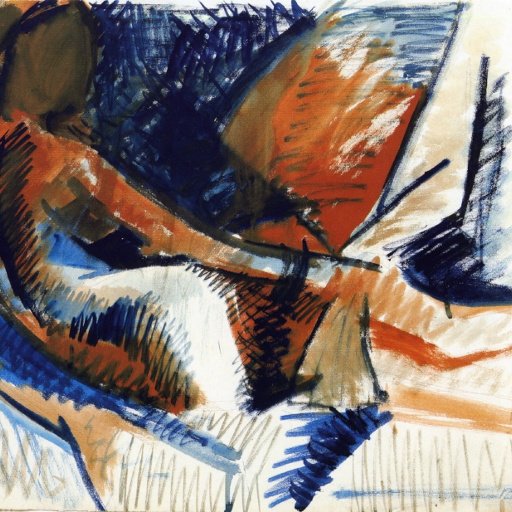
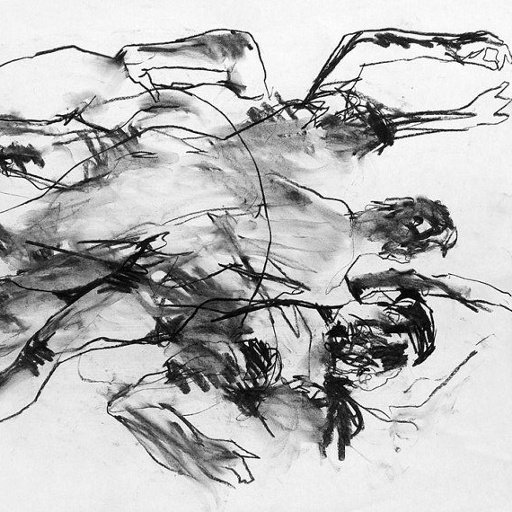
(Left: previous iteration looks OK, Right: pixels go far out of range → clamped)
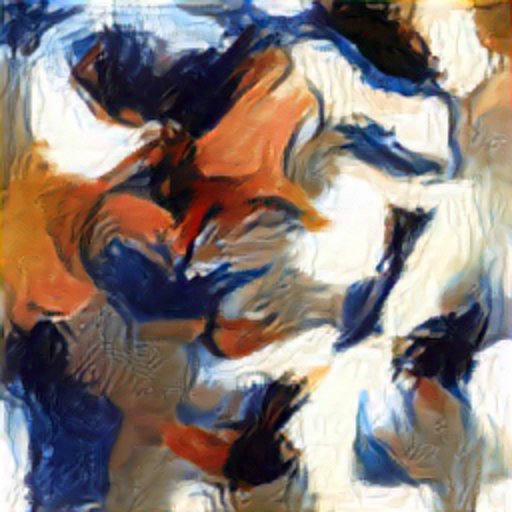

It was ahead of its time: first SaaS for neural style, first with true HD support!
It helps provide a sense of what the model can understand! #procjam #neuralimagen
Other tweaks I made diminished the benefits of this approach. 💡🤔
Here, blending weights between conv3_1 and conv1_1. Nice patterns or correct colors, pick one:
These images are #generative, and it's beyond my expectations:
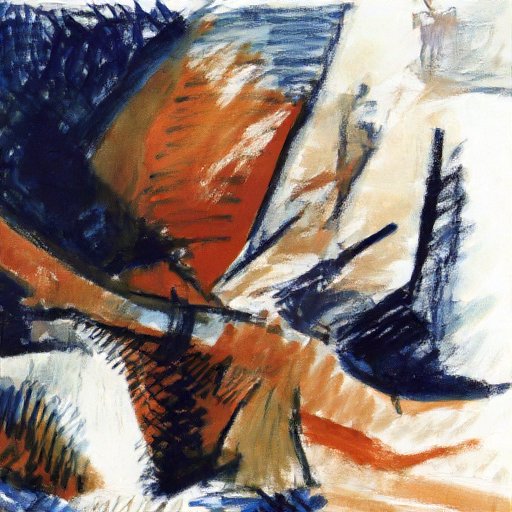
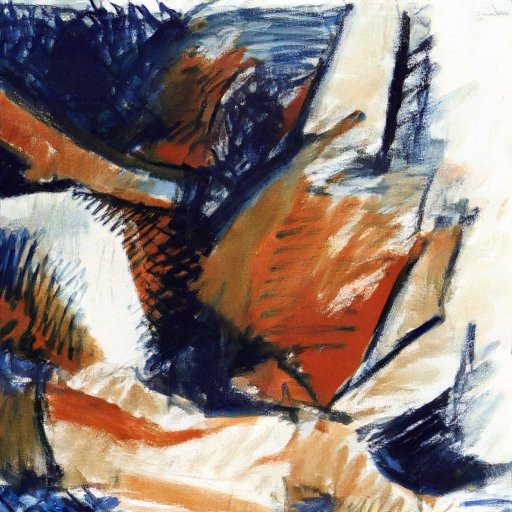
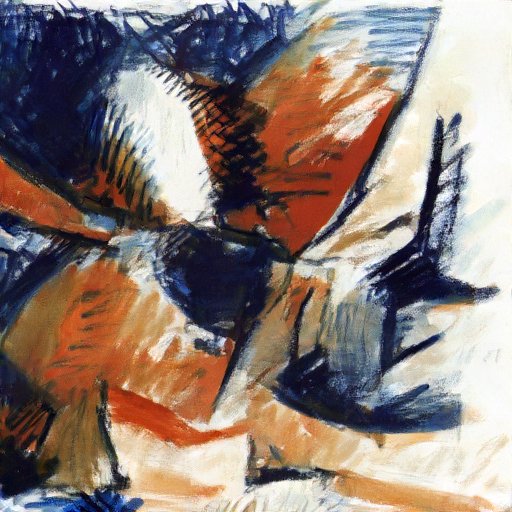
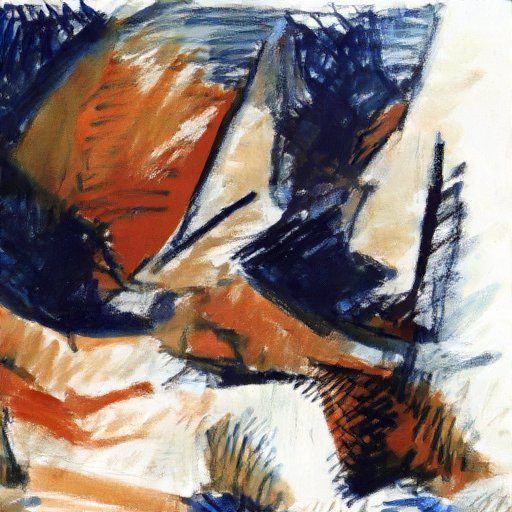
There are clearly image sections that are reproduced from location-independent statistics, but the convnet does a great job of mashing up the elements in new ways—and that's what #NeuralStyle does the best.
Until now, it was unclear to me whether this representation was sufficient for such complex styles.
I don't know how well it does as compression mechanism yet! 🗜️