- Seshadhiri Dhanasekaran
இன்று நாம் காண இருக்கும் டாபிக் Gradient Descent. இது ஒரு optimization (மேம்படுத்தல்) முறை.
#MachineLearningTamil
#Thread
#MachineLearning
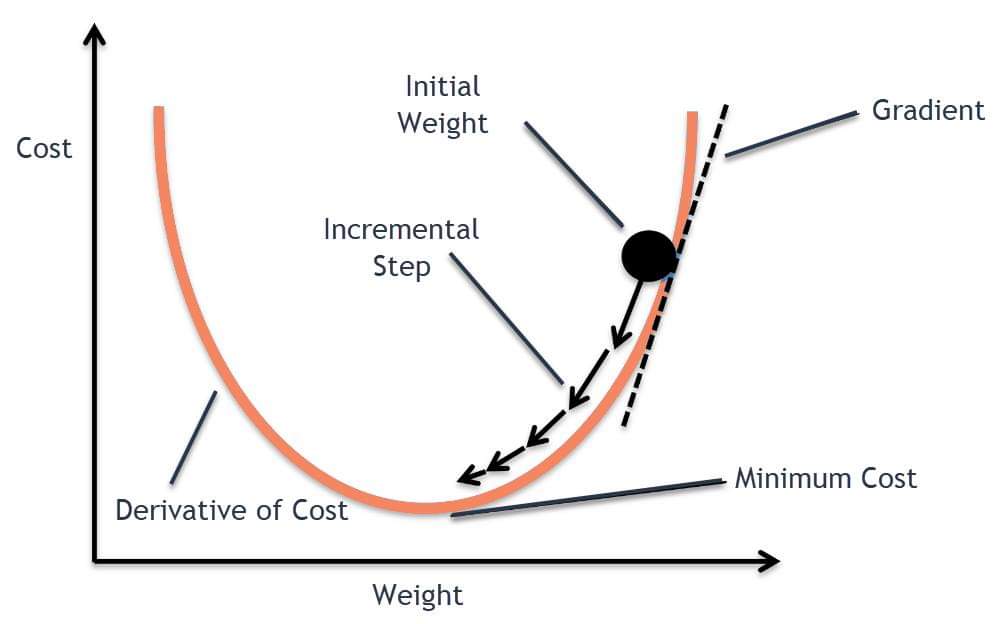
நீங்க இருக்க பொஸிஷன்ல இருந்து மலை உச்சியை பார்த்து கண்னுக்கு தெரிந்த பக்கத்தில் உள்ள பகுதிகளை கொண்டு அப்படியே கீழ இறங்க வேண்டும்.
பின்பு ஒரு தடவை Iteration (கற்றல் ) நடந்த பின் அதற்க்கான cost கண்டுபுடிக்க வேண்டும். இந்த cost எப்படி கண்டுபிடிப்பது என்றால்
cost = f(coefficient)
cost = evaluate(f(coefficient))
Delta = Derivative(Cost)
கற்றல் ஆரம்பித்த பின் இந்த coefficient value மாறும்
மாறின coefficient value கண்டு புடிக்கணும்
ஆல்பா என்பது learning ரேட் (கற்றல் ஆற்றல்) இது 1 மற்றும் 0க்கு இடையில் தான் இருக்கும்
coefficient = coefficient * (alpha * delta)
இதை கொண்டு நாளை ஒரு algorithm performance எப்படி மாற்ற முடியும் என்று பார்ப்போம்.
இதை நீங்கள் சால்வ் செய்தால் x = 9/4.
இதில் plateau point 0 , அதிகம் 9/4.
இதை எப்படி pythonல செய்வது என்பதை இணைத்து உள்ளேன்.
#EndofPartIX
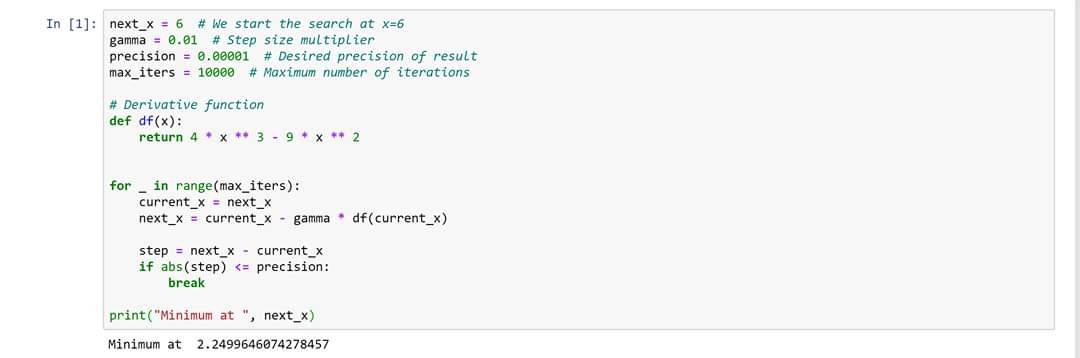