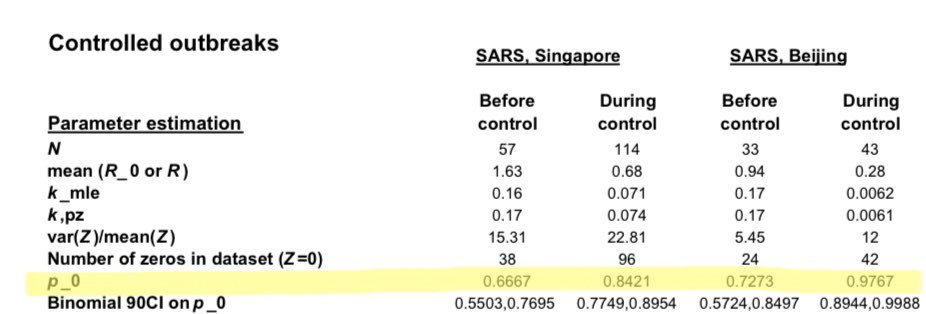
Banning large gatherings (and smaller ones) probably big part of #shutdown success
Working from home probably helps a lot with that
Masking everyone could help because then “superemitters” also wear masks and that might reduce spread from them
Keep Current with Kai Kupferschmidt
This Thread may be Removed Anytime!
Twitter may remove this content at anytime, convert it as a PDF, save and print for later use!
1) Follow Thread Reader App on Twitter so you can easily mention us!
2) Go to a Twitter thread (series of Tweets by the same owner) and mention us with a keyword "unroll"
@threadreaderapp unroll
You can practice here first or read more on our help page!