ICYI, have a couple of thoughts to share about this paper
1/n
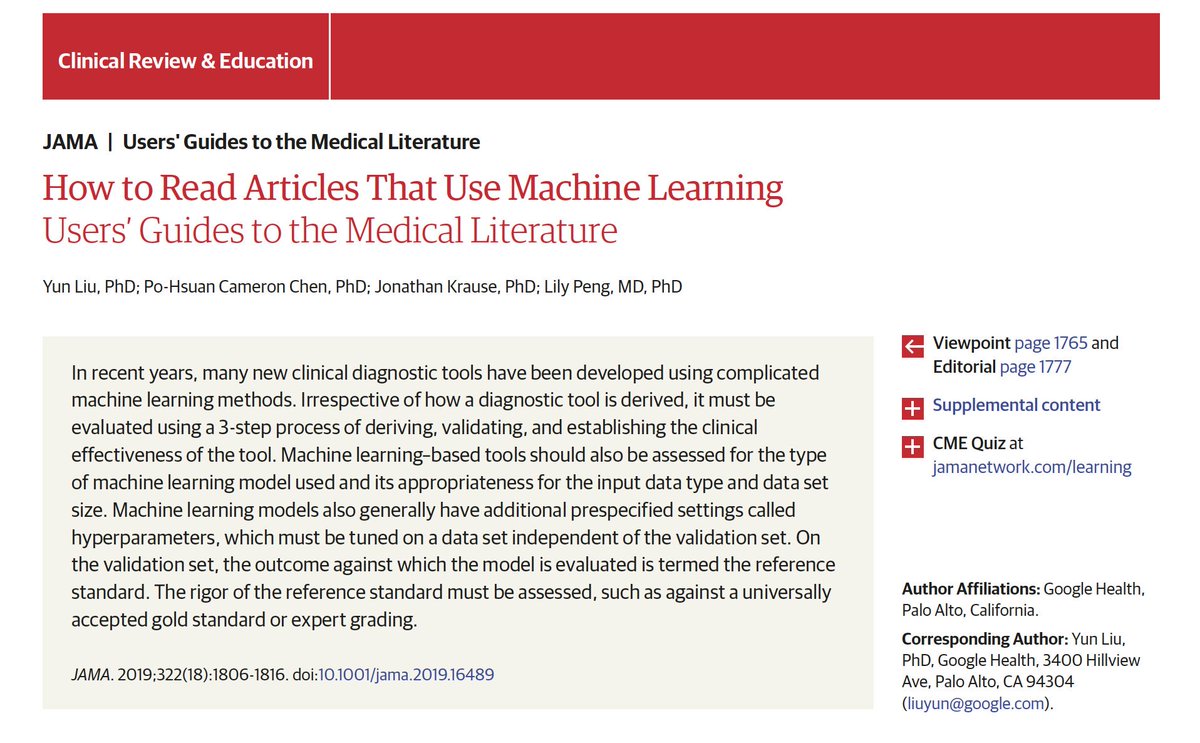
To start, I like the explanation of the terminology and concepts. Nice use of text boxes, imo
2/n
3/n
equator-network.org/reporting-guid…
4/n
thelancet.com/journals/lance…
5/n
6/n
jamanetwork.com/journals/jama/…
7/n
8/n
9/n
10/n
11/n
12/n
But do we really know how much data we need for developing and validating reliable complex algorithms? Probably not
14/n
/end