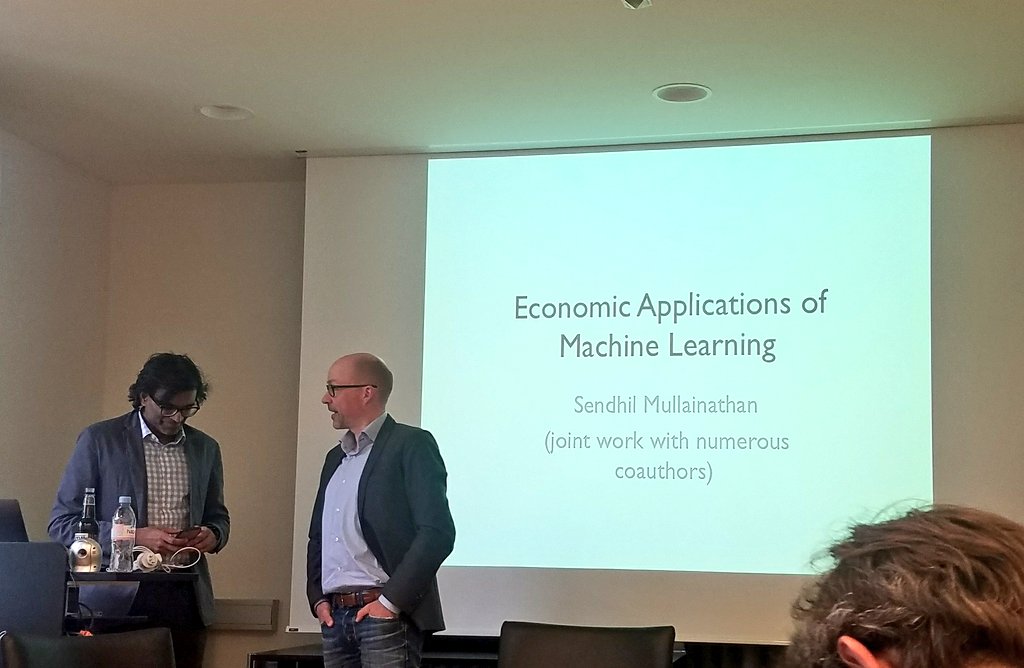
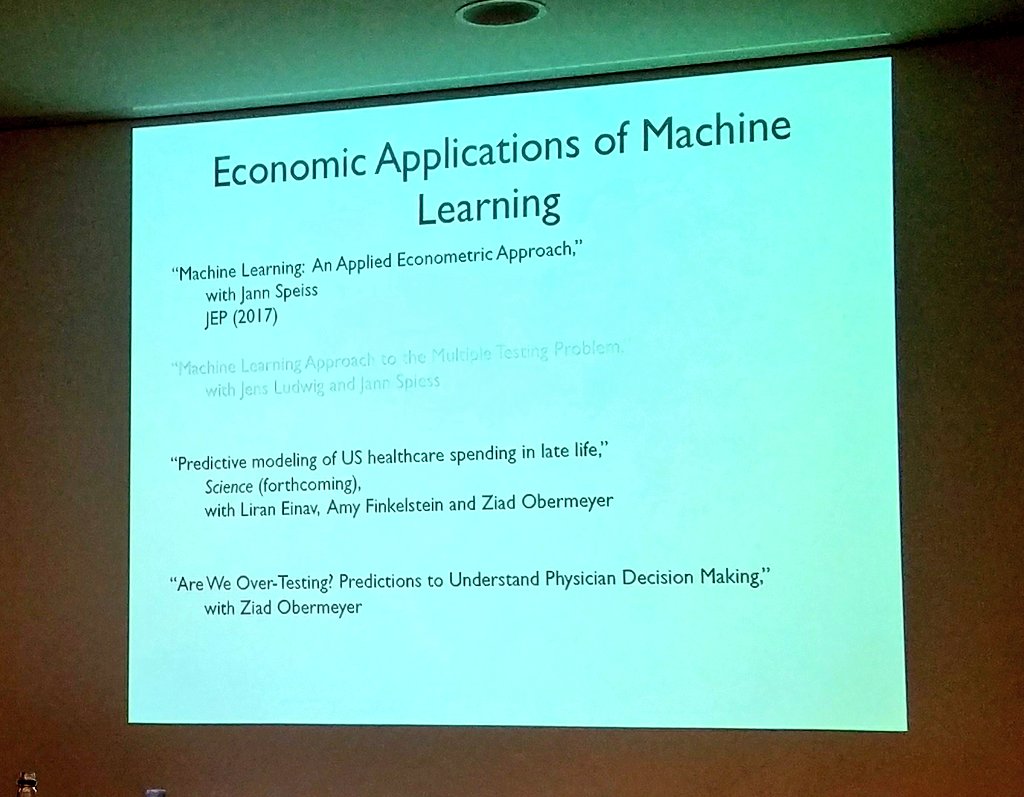
The first step is to transform the engineering problem into an empirical problem. Rather than programming what a face looks like, make it into an empirical learning exercise. Is this a face, yes or no?
A large part is like money ball: application of statistical methods to new areas.
But that's not all.
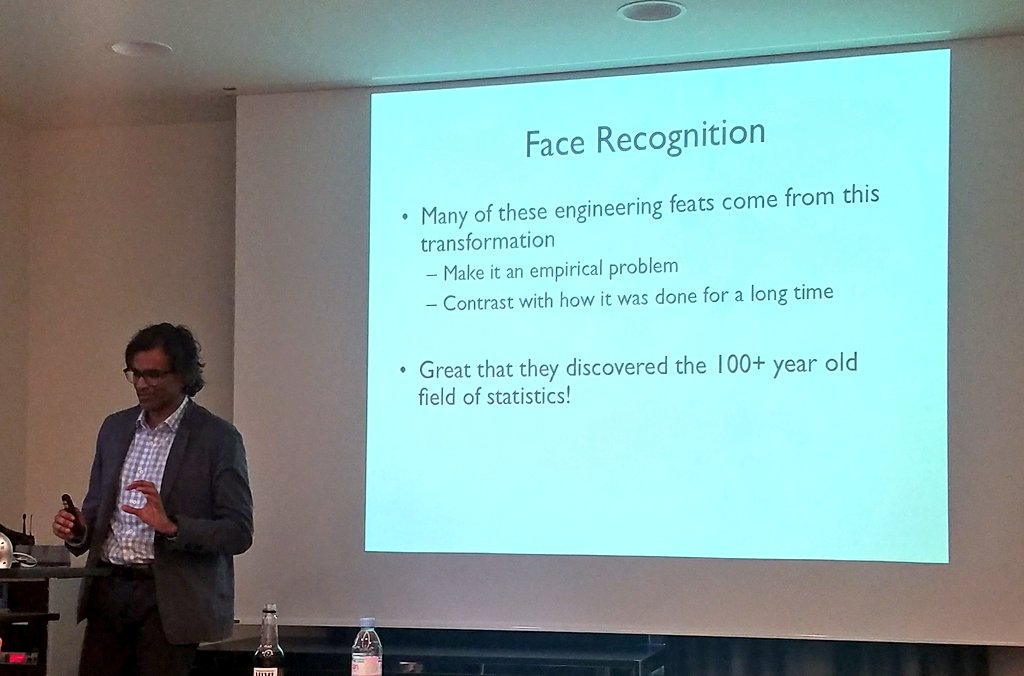
This type of high-dimensionality is relatively new. Especially what is new is that there are now a set of practical tools for this "applied nonparametrics".
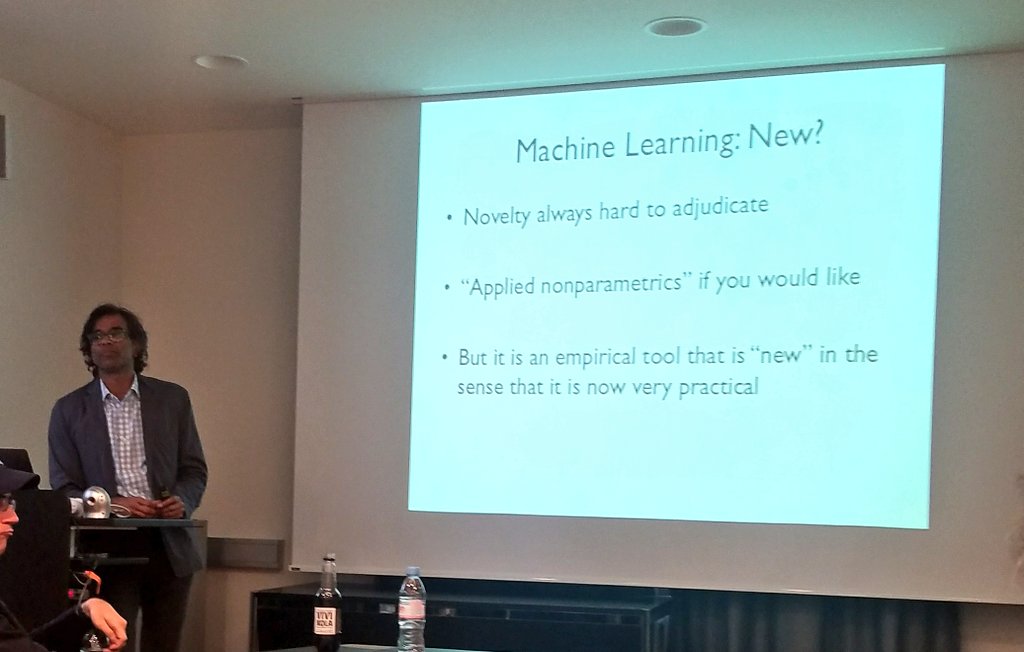
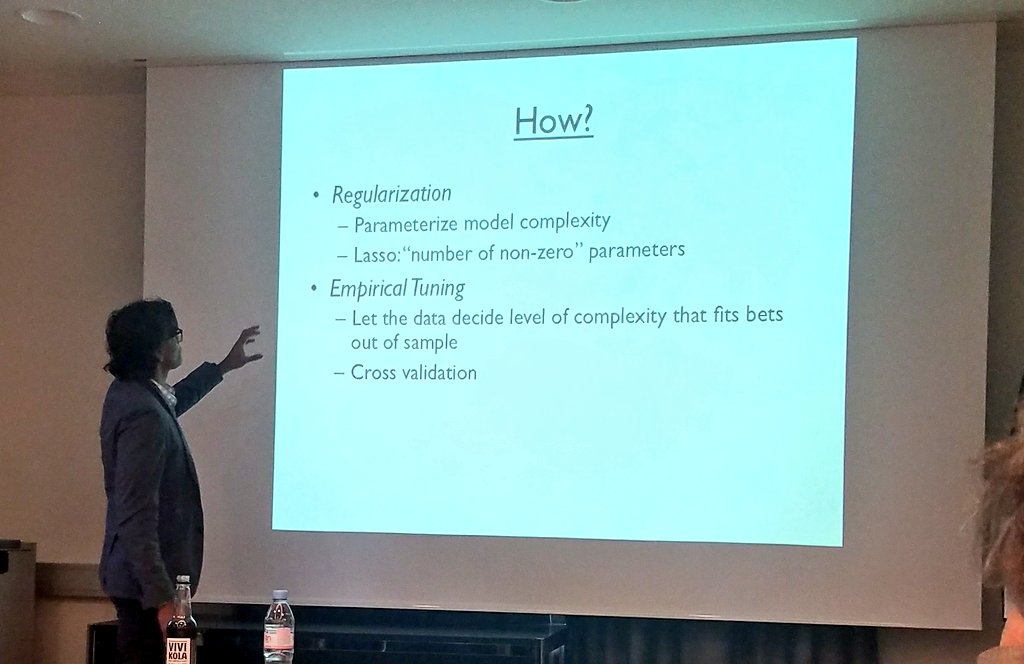
Improvement of R-square from OLS to bettet method from 42% to 46%. Is that a lot? Do we care?
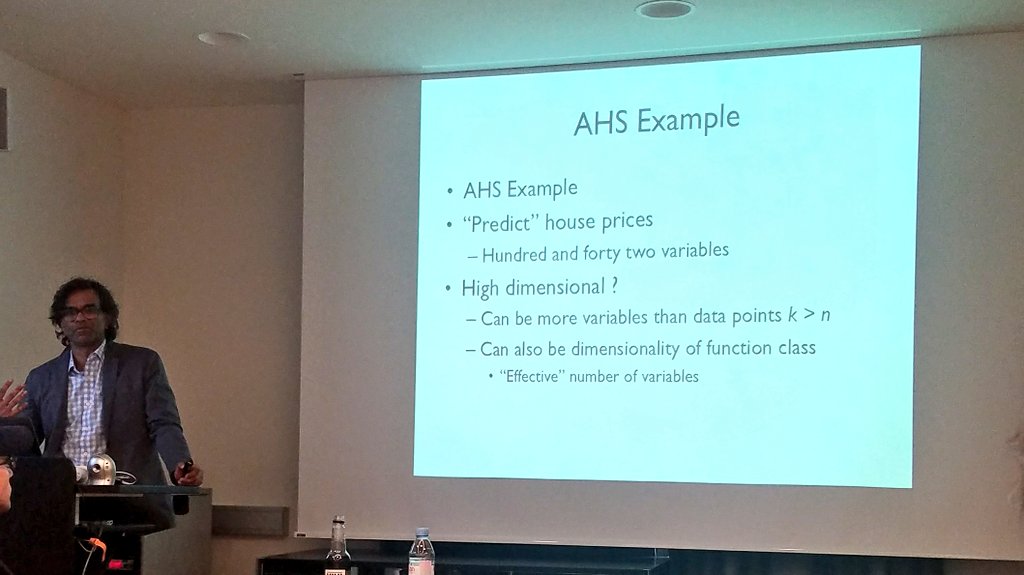
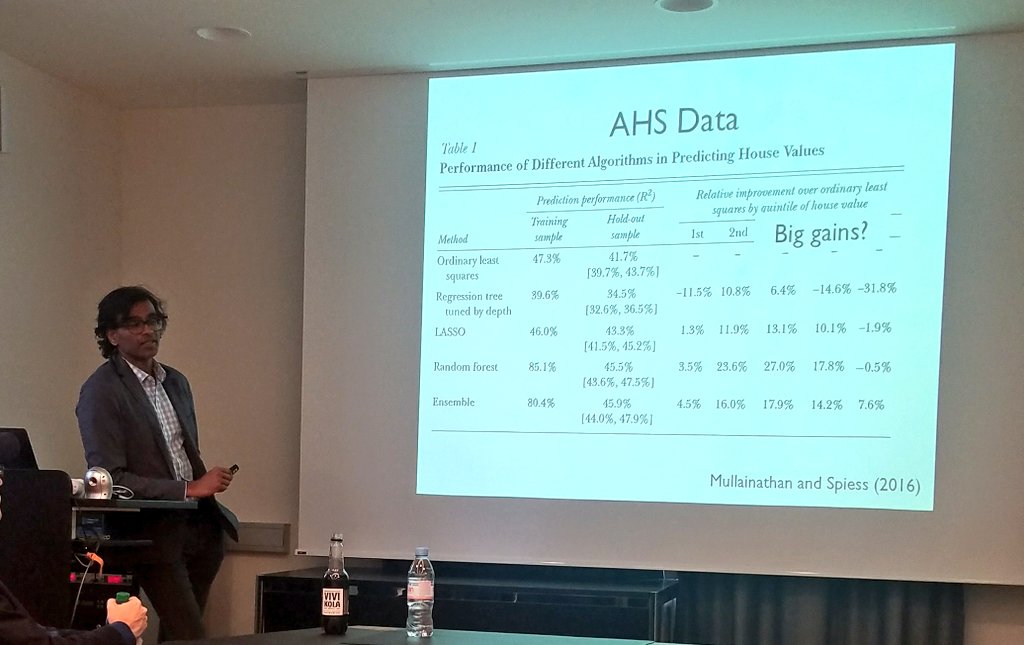
First fits Y to X with low dimensionality. Machine learning does it out of sample and with high dimensionality.
Is machine learning just better?
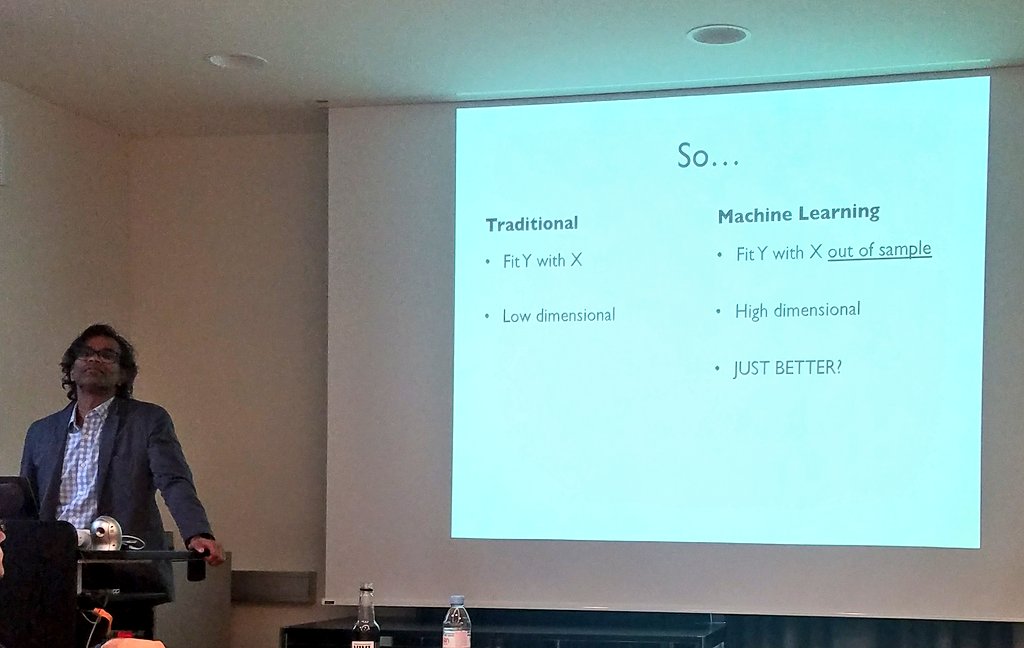
Economist often care less about that & more about the causal role of specific covariates.
E.g. if two covariates are correlated, we care about that for parameter estimation, but not for prediction.
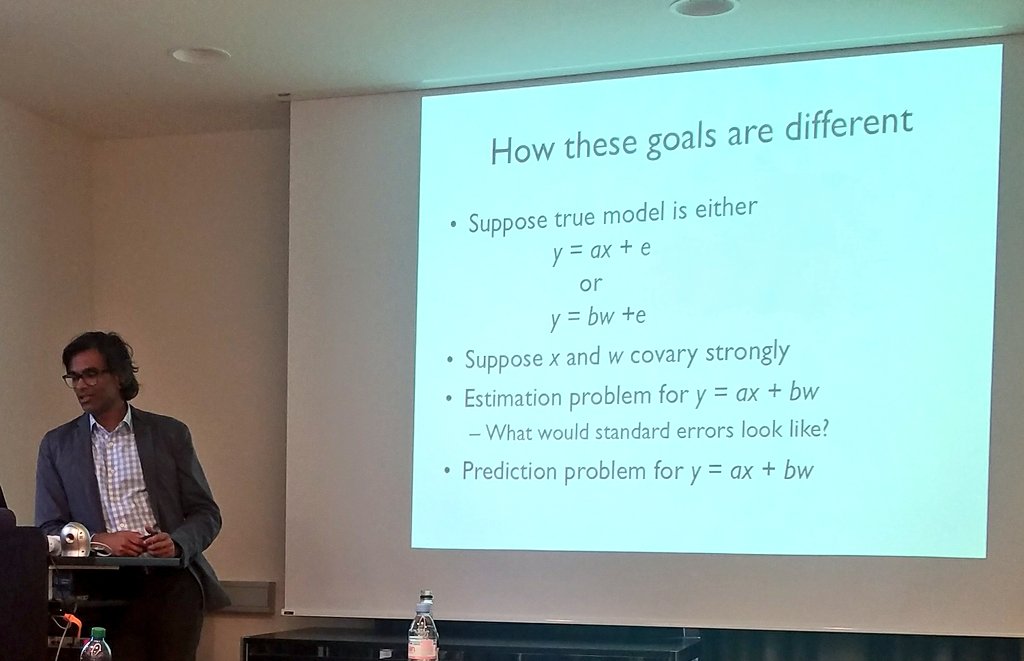
We'll now look at 3 types of application for economics:
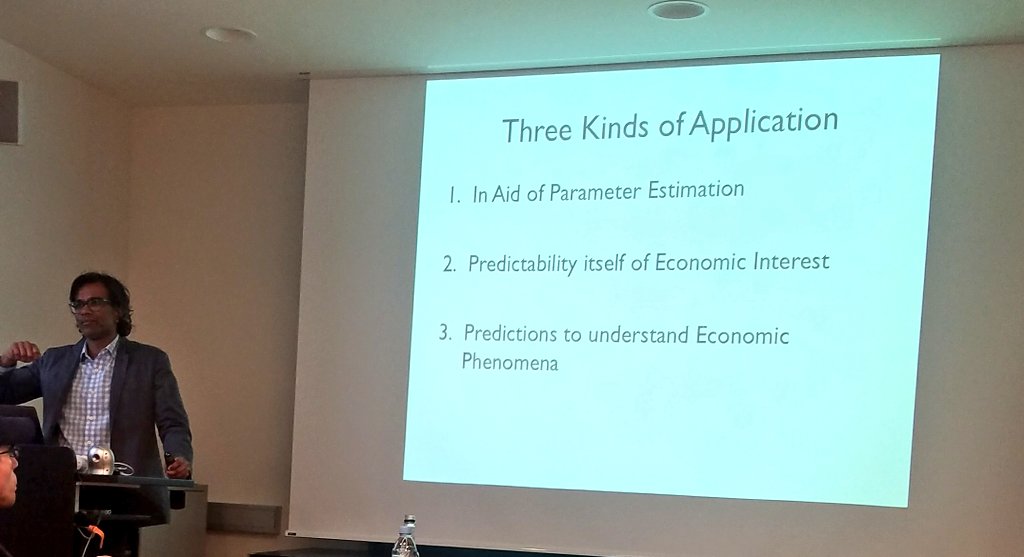
Experimental analysis. Imagine I ran an RCT & I have a large number of outcomes. I want to see if there's an overall effect.
There are also ways to get exact p-values here.
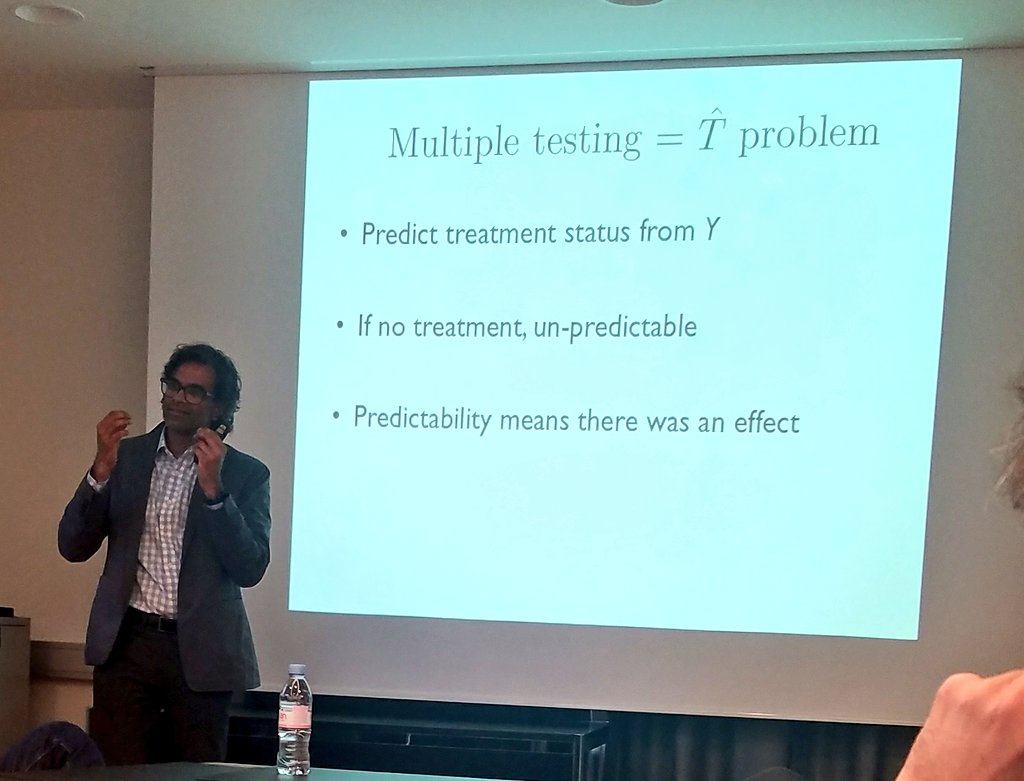
We know already that the question about which group responds more is not causal.
See work by Athey and Imbens on this.
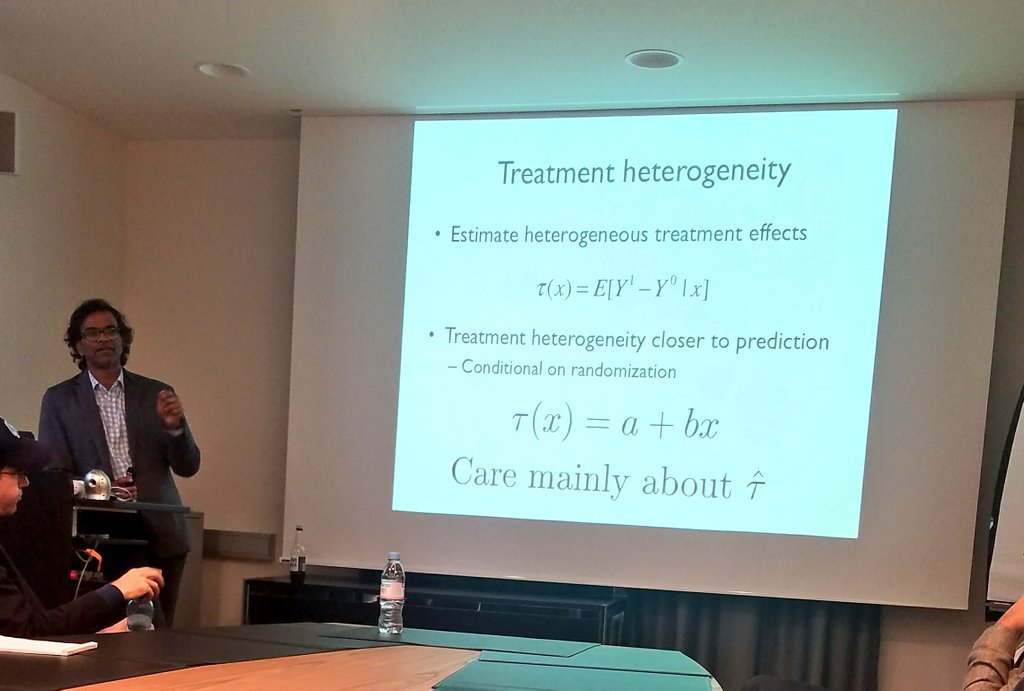
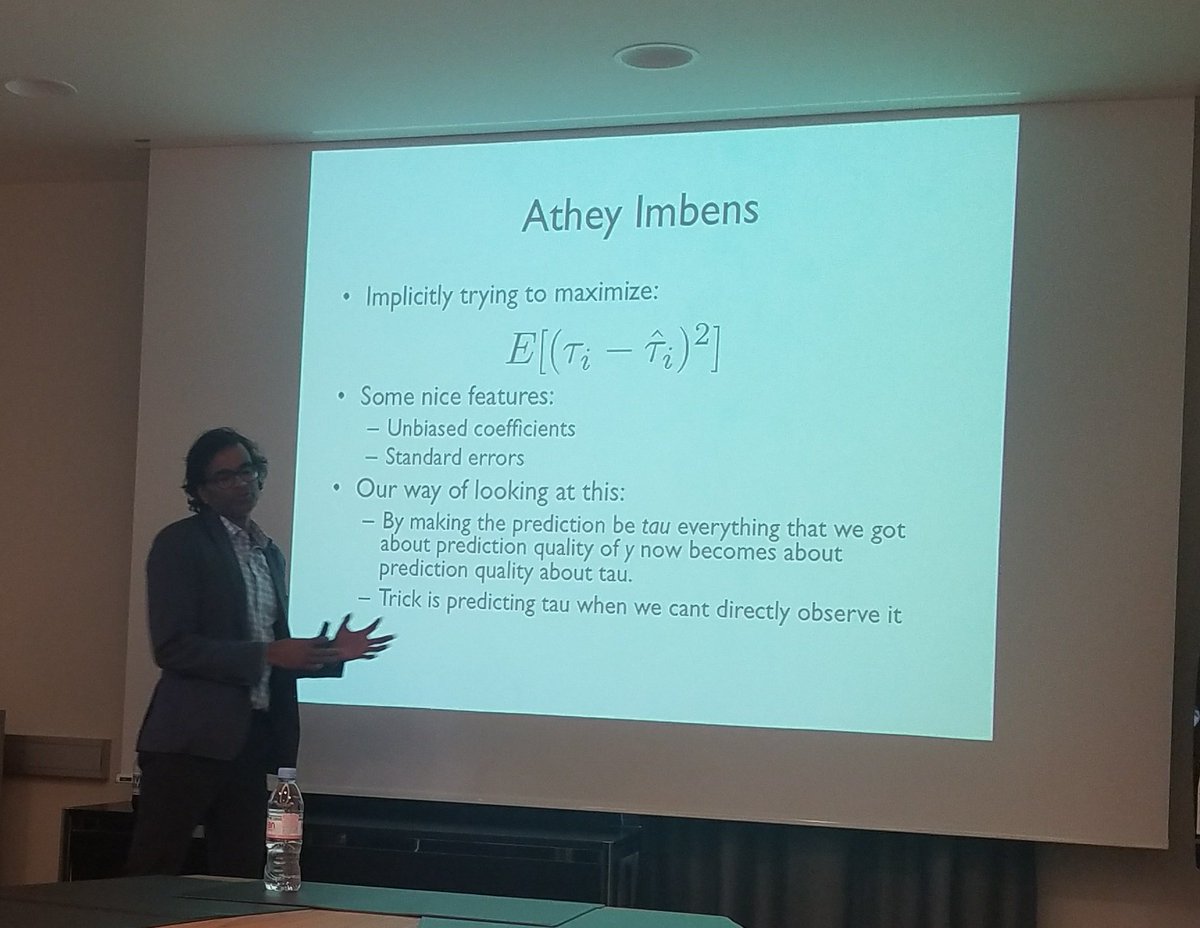
Here's a list:
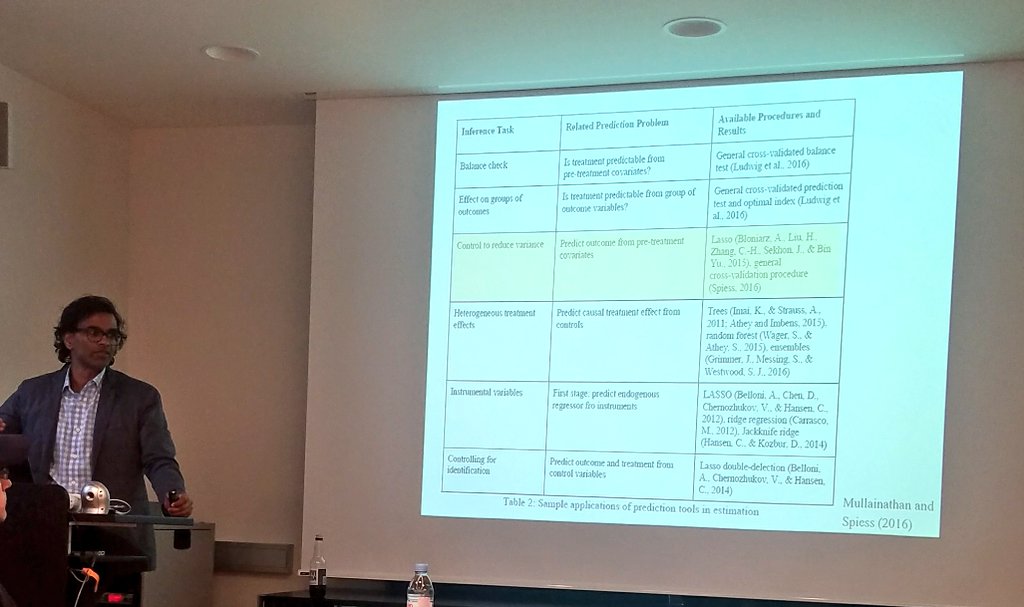
Example: 25% of health spending is in last part of people's life. If we could predict who would die despite of this treatment, we would not need to spend this amount and to make people undergo treatment.
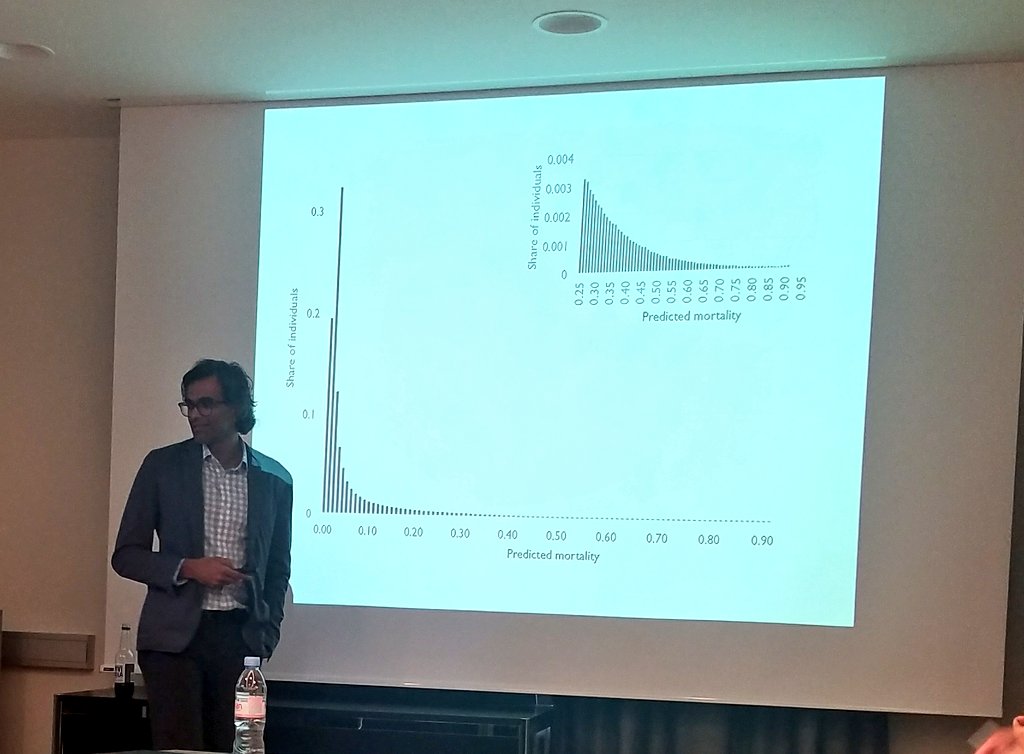
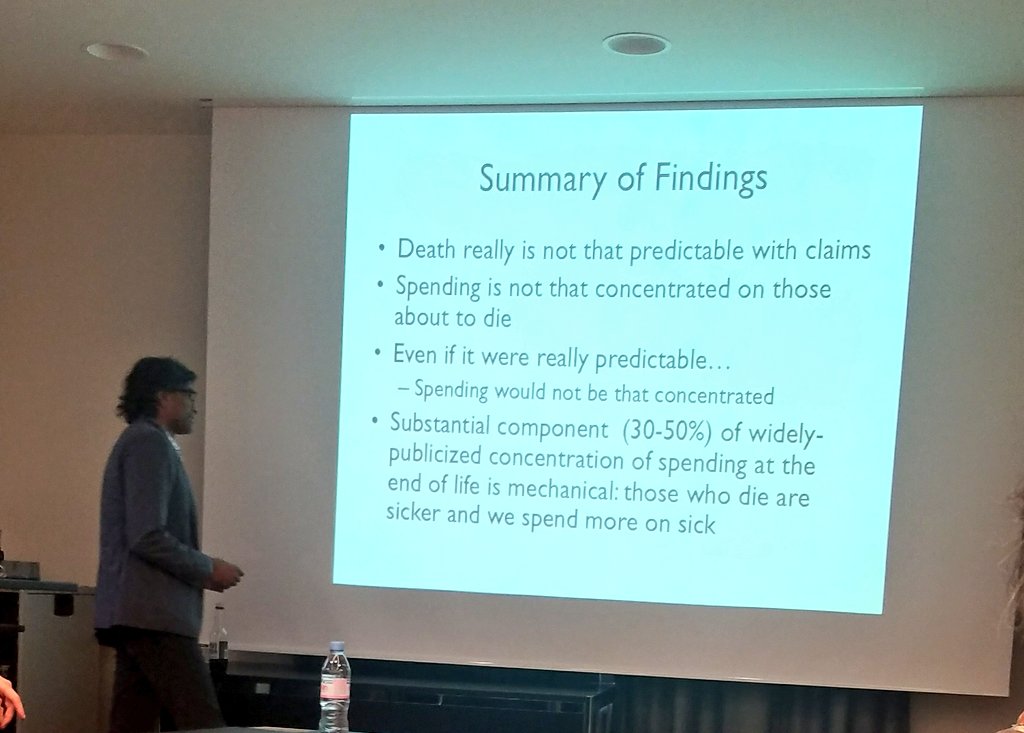
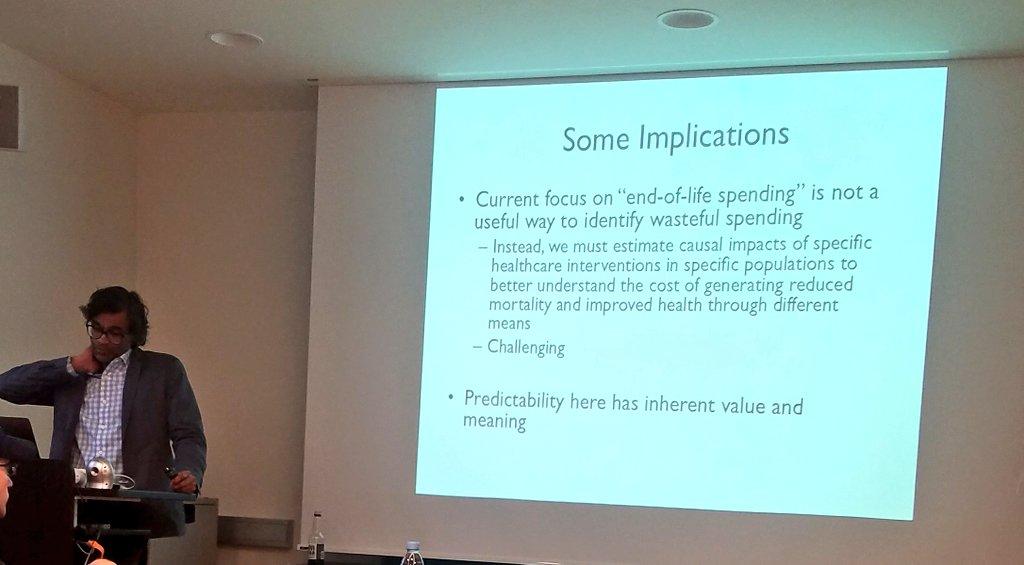
The decision of who to test is actually a prediction problem: I wanna test the people for whom the test will find that they have a heart attack.
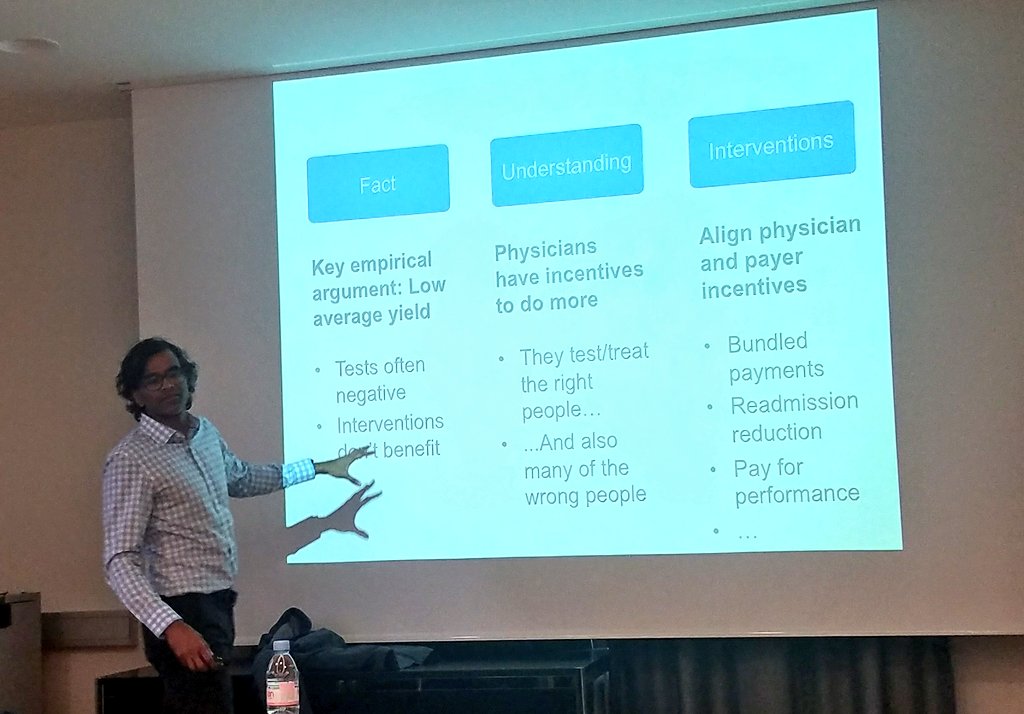
There is under-testing of some people and over-testing of others.
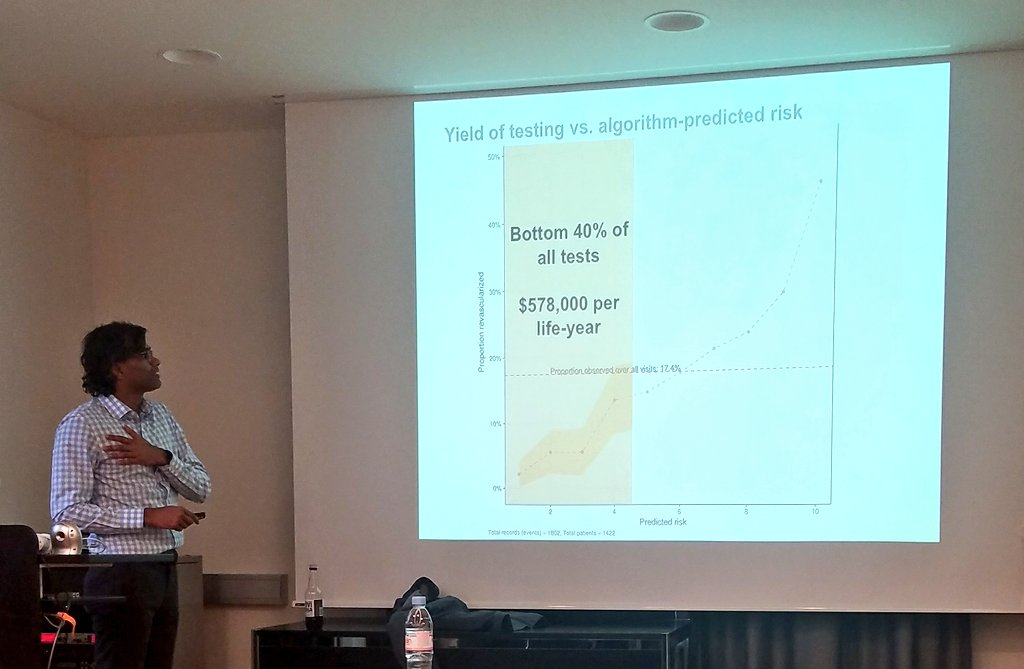
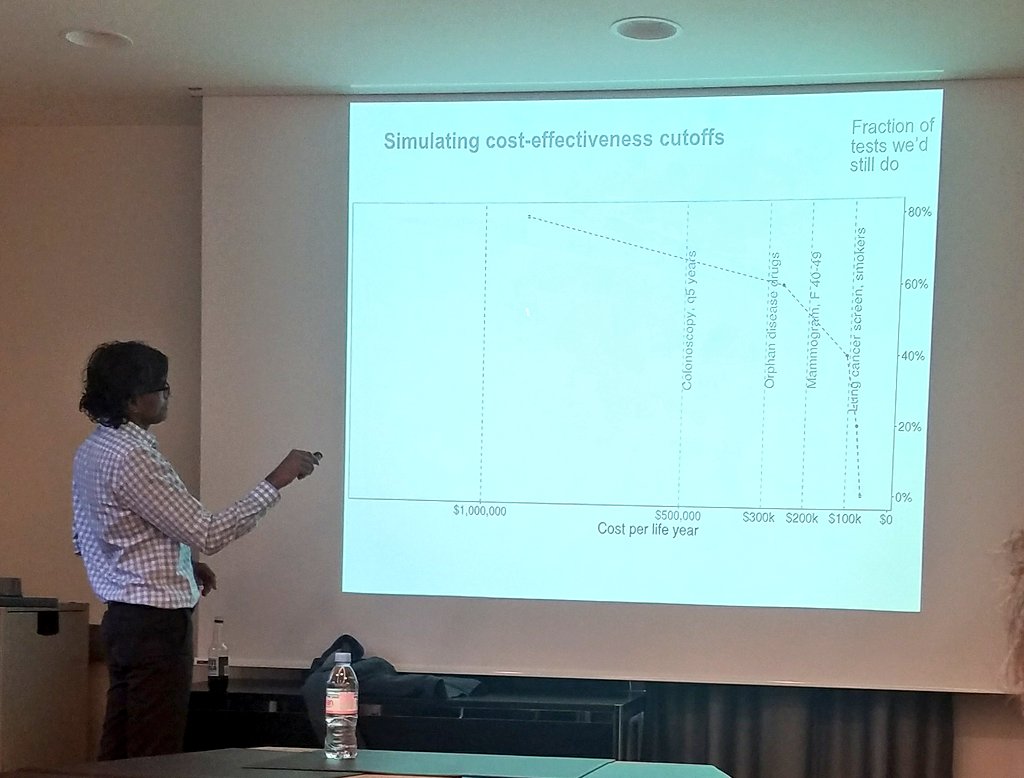
Then testing on that selected sample will not be sufficient.
Need to look at what happened to the untested later on, and see if they got heart attacks.
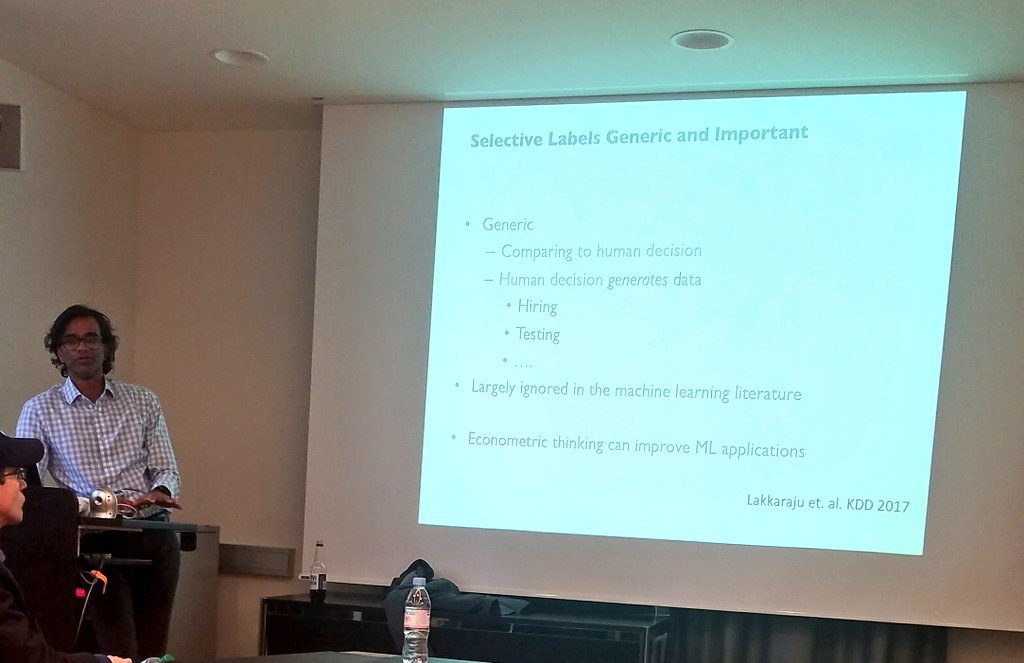
It's a problem of sorting, of not predicting well whom to test. Not a case of generalized overtesting.
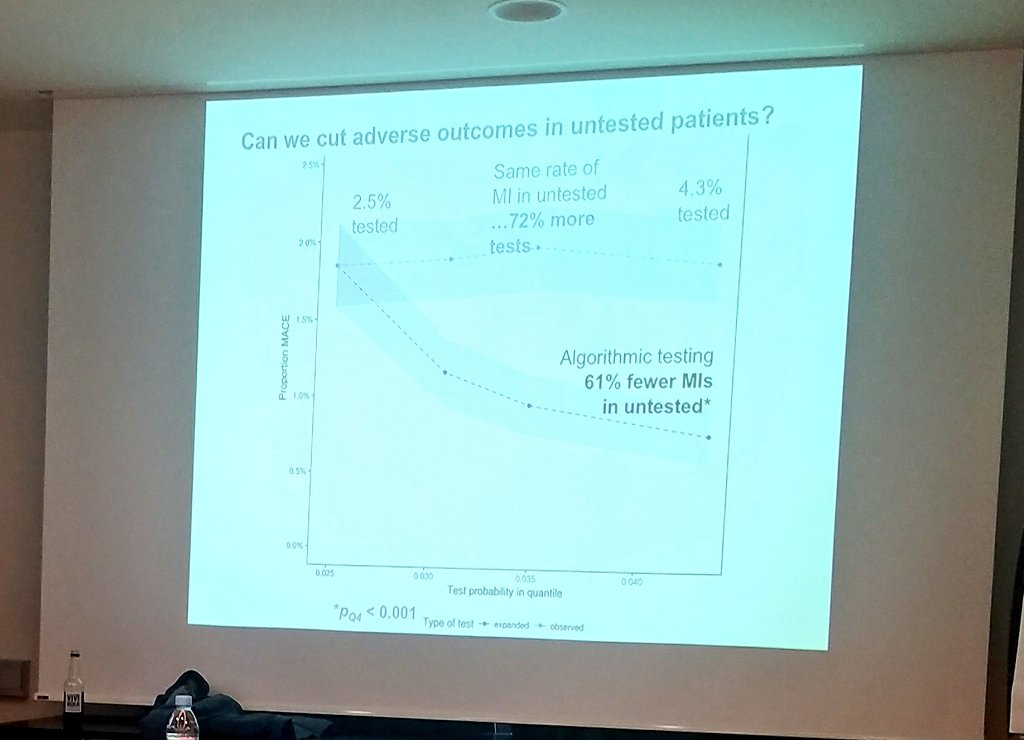
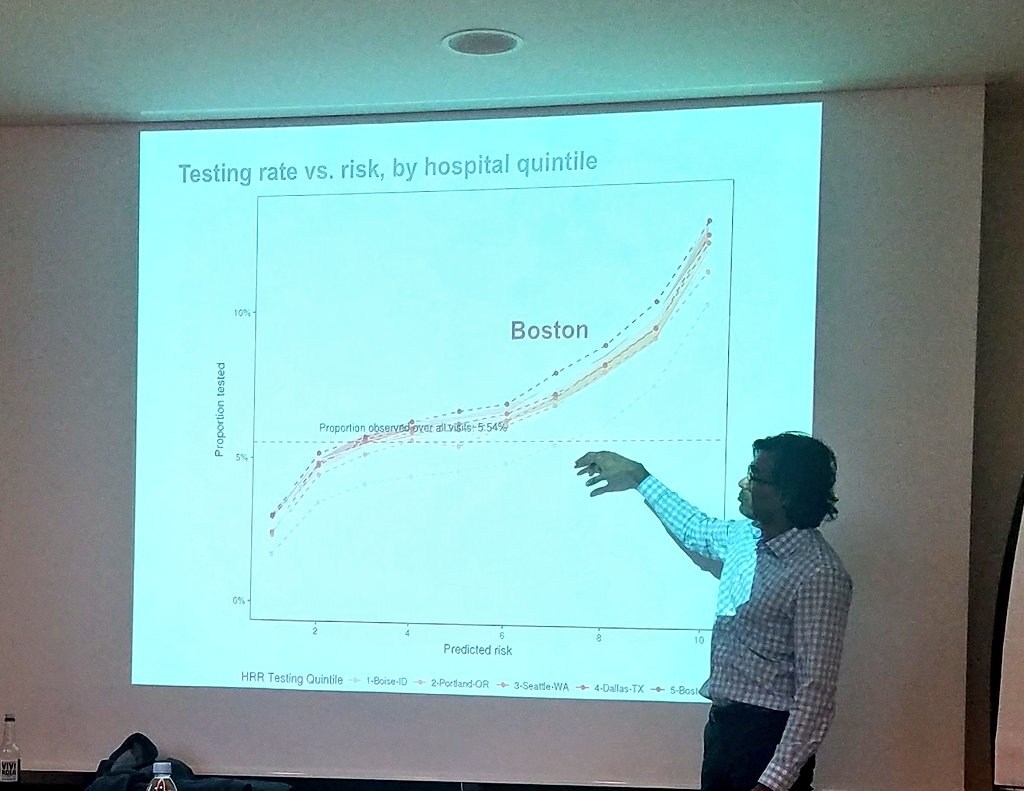
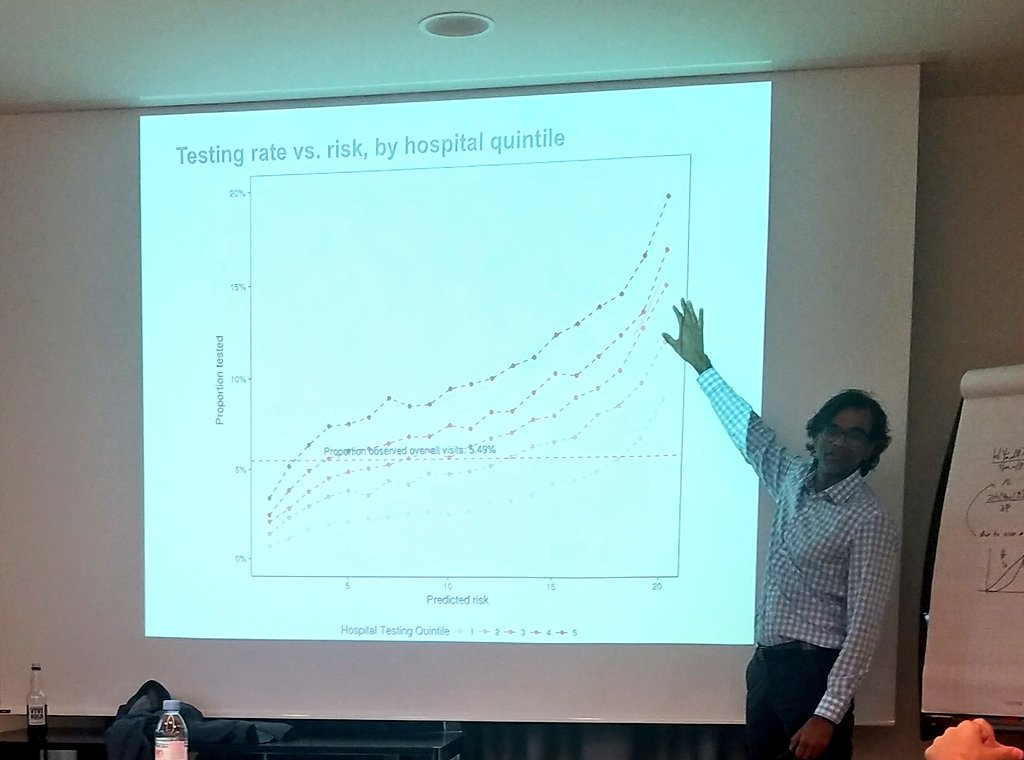
Machine learning may help us improve in answering certain questions that economist already ask.
In addition, it allows us to ask entirely new questions.
/end