Happy to finally share the published version of our paper on hierarchical learning and confidence, out now in @PLOSCompBiol
journals.plos.org/ploscompbiol/a…
TL;DR? Let me unpack it in a bunch of tweet-sized bites.
0/n
You may know them from beautiful papers, e.g. by @beckyneuro or by Powers (both with Mathys) where the authors link specific psychiatric traits to specific parameters in such a hierarchical learning model.
2/n
Non-hierarchical, (flat) models are computationally cheap and can learn very effectively, even in changing environments.
So we ask: how can we test that learners actually use a hierarchical model?
Why is that important? See this nice paper by @StePalminteri et al.
doi.org/10.1016/j.tics… …
4/n
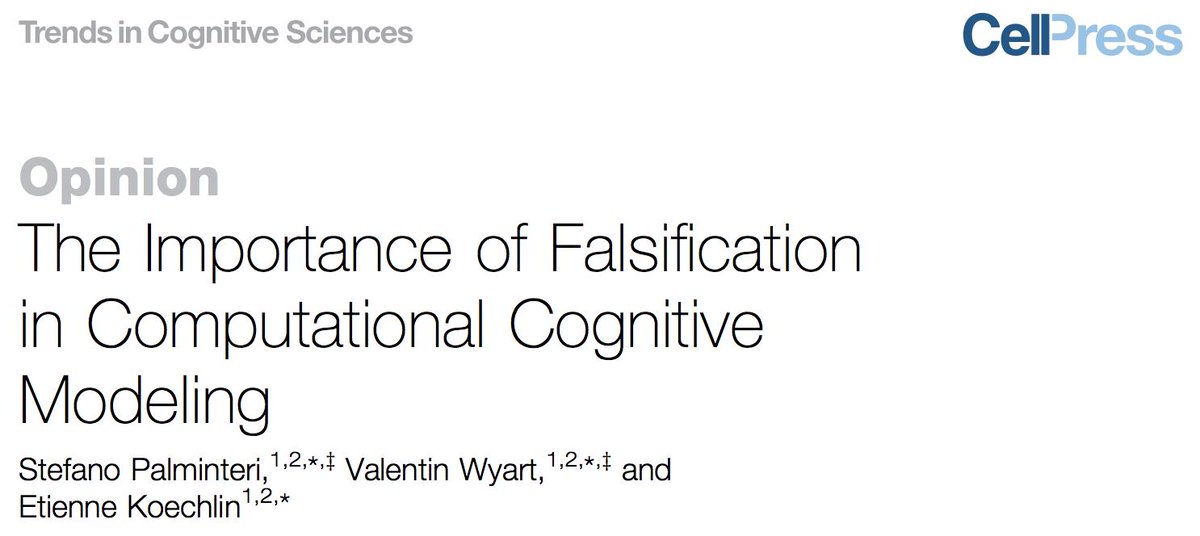
So how can we test more definitively if learners used a hierarchical model?
10/n
.. but participants didn’t know: they were just informed to track the regularities, and thought everything was random.
This dovetails nicely with ideas that hierarchical probalistic inference is central and comes naturally to the brain..
\fin
And shout out to Florent and @maheump who made most of the ideal observer models we used, for an earlier paper, found here:
journals.plos.org/ploscompbiol/a…