🗺 290km of mapped roadway
👀 11,319 tracked objects with raw sensor data
🧠 327,790 sequences of interesting scenarios
🔌 A thoughtful API to interact with the data
Let's explore...
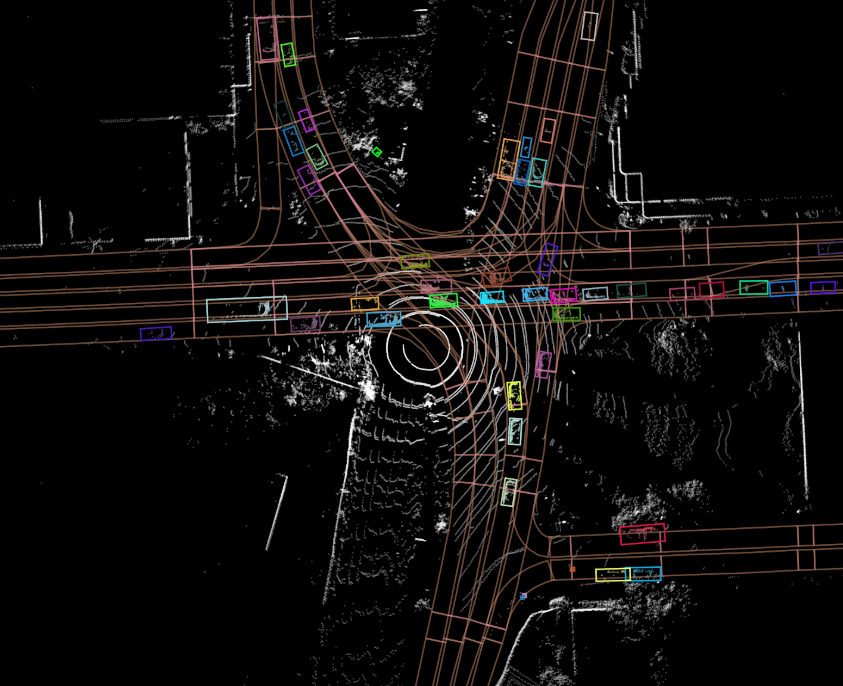
@argoai mined 1000 hours of driving to generate 327k interesting 5-second segments of ground-truth data.
Engineers can train prediction models on this ground-truth data, to better guess what objects (cars, pedestrians, etc.) around the self-driving car will do next.
Better predictions means a smoother, and safer ride.
For motion forecasting, given 2 seconds of motion, can you predict what the object will do in the next 3?
Here is an attempt from @argoai. Red is ground-truth and green is the prediction. Tough problem!
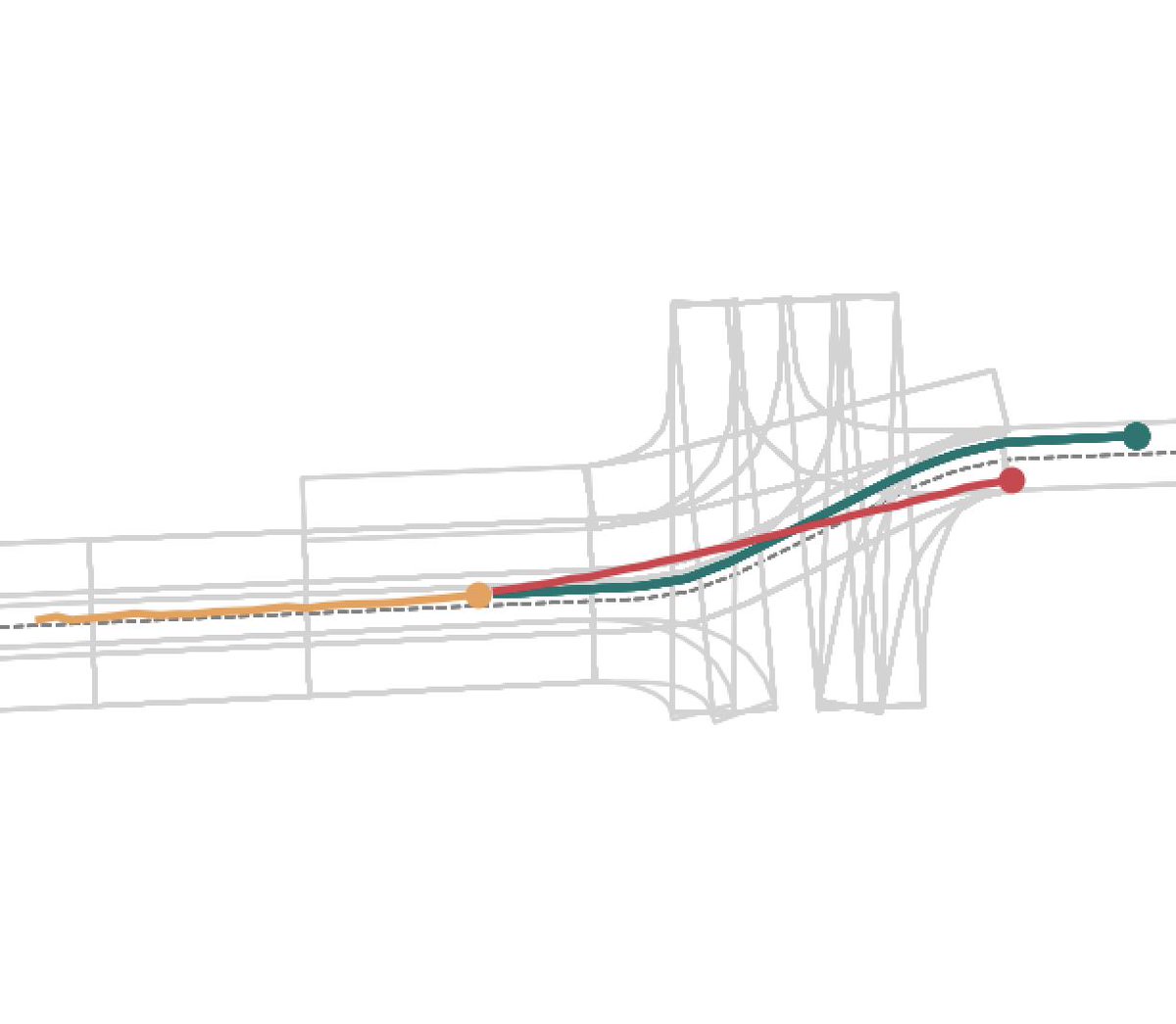
This is where Argoverse's 3D object tracking dataset comes in handy. @argoai has released 11,319 tracked objects recorded across 113 15-30 second segments.
What makes this 3D object tracking dataset even more special is, you guessed it, the included HD map! Utilizing lane-level information can improve your perception accuracy.
Argoverse is going to enable a whole bunch of innovation. The community is grateful 🙏