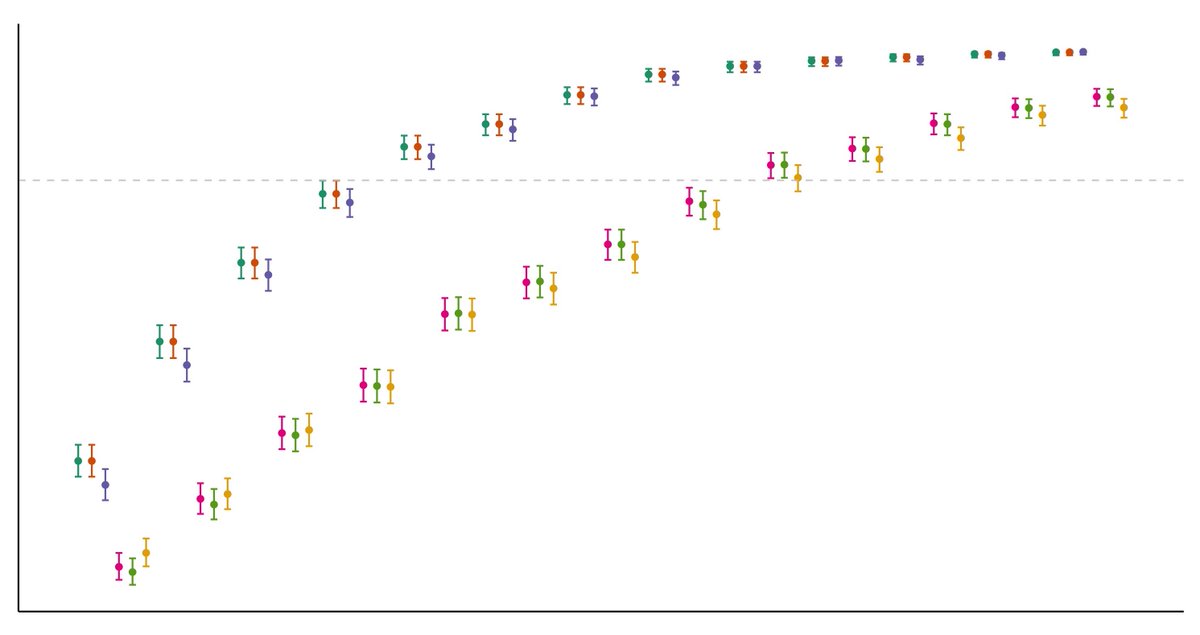
(1) The causal relationship b/w a variable and Y has no impact on its likelihood for being imbalanced b/w randomized groups.
(2) A higher burden of chance imbalances in predictor variables does impart a higher likelihood of imbalance in Y.
12/n
From this point on, each time we sample from the population we'll add a treatment effect of 0.25 to half of the sample and try to recover this value in our models.
13/n
1. No adjustment
2. All imbalanced variables
3. Imbalanced predictors only
4. All variables
5. Strong predictors (C1-3)
6. All predictors (C1-10)
14/n
1. All strategies appear to be unbiased (all est. centered at actual value)
2. Adjusting based on imbalance doesn't help much, esp. if not focused on predictors (power of "imbal. predictors" strategy slightly > "all imbal." strategy) ...
16/n
4. There is a slight disadvantage to excessive/unnecessary adjustment ("all variable" strategy has slightly higher power than the "all variables" strategy).
17/n
Disclaimer: I am not a statistician. I think the reason is, at least in part, the additional degrees of freedom we gain with increased sample size. This changes things for a few of our adjustment strategies ...
19/n
20/n
21/n
22/n
26/n
27/n
1. Predictors & non-predictors of outcome are equally likely to be imbalanced
2. More imbalance in predictors=more likely imbalance in outcome (not true of non-predictors)
3. Best adj. strategy: all predictors (at least the strong ones) regardless of imbalance. 28/n
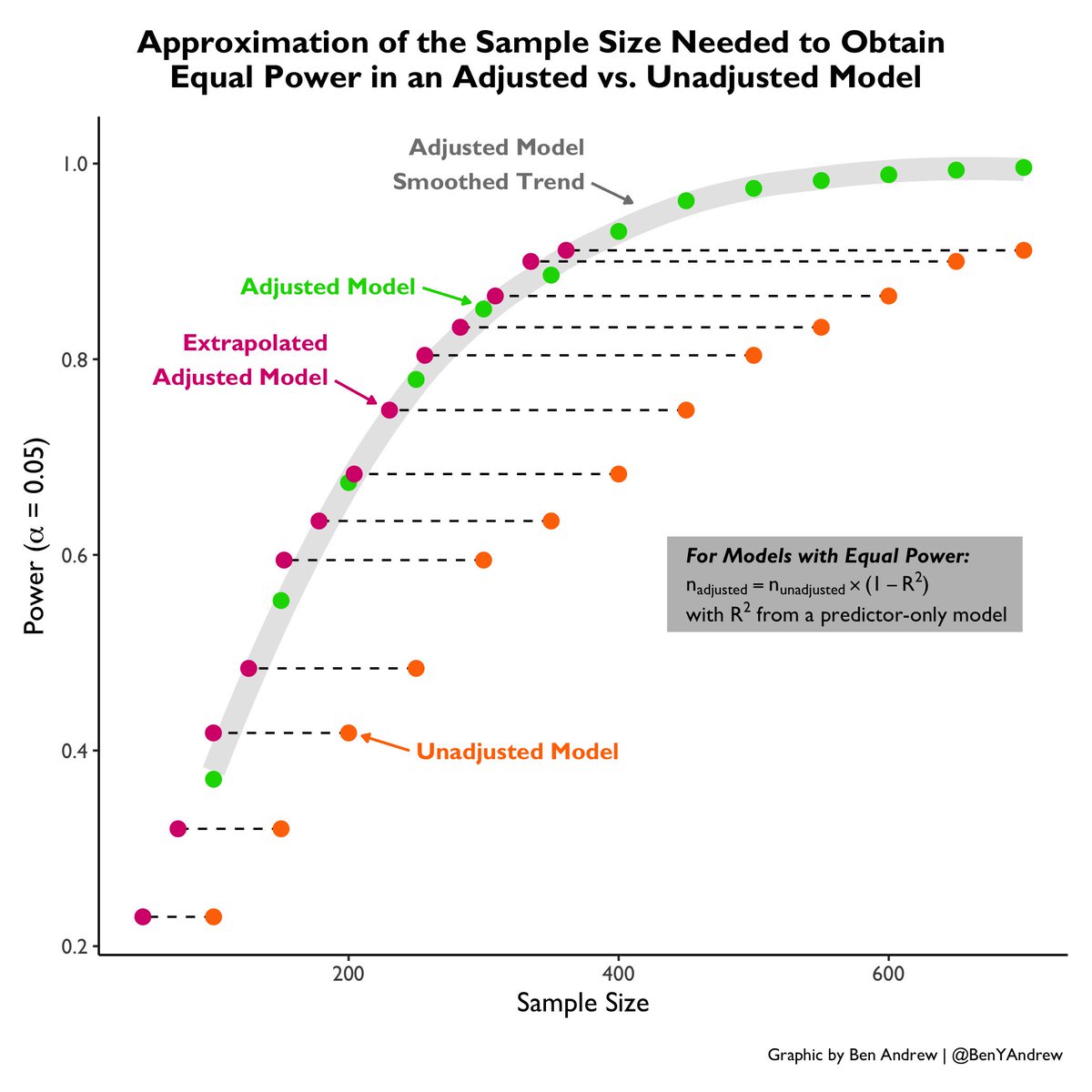