Universality and individuality in neural dynamics across large populations of recurrent networks
arxiv.org/abs/1907.08549.
With fantastic collaborators @niru_m, @ItsNeuronal, @MattGolub_Neuro, @SuryaGanguli.
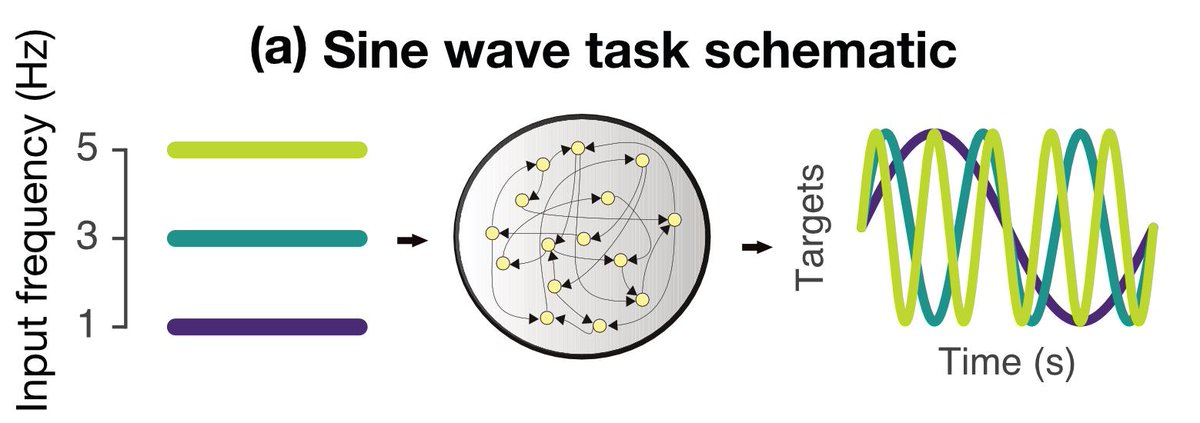
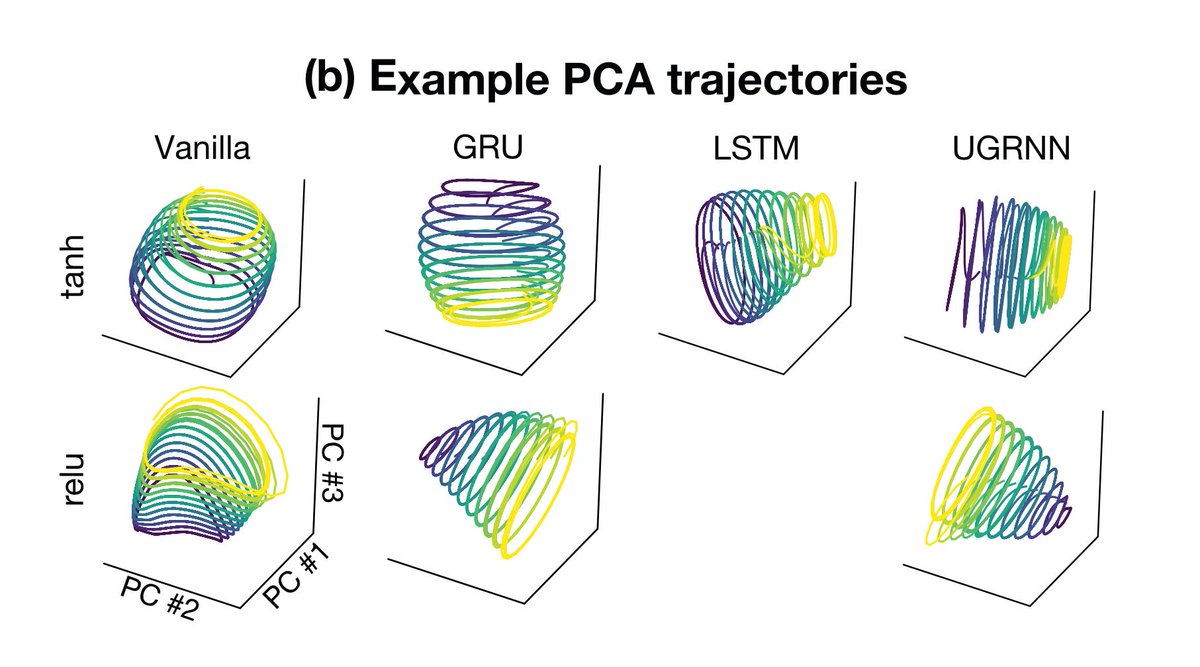
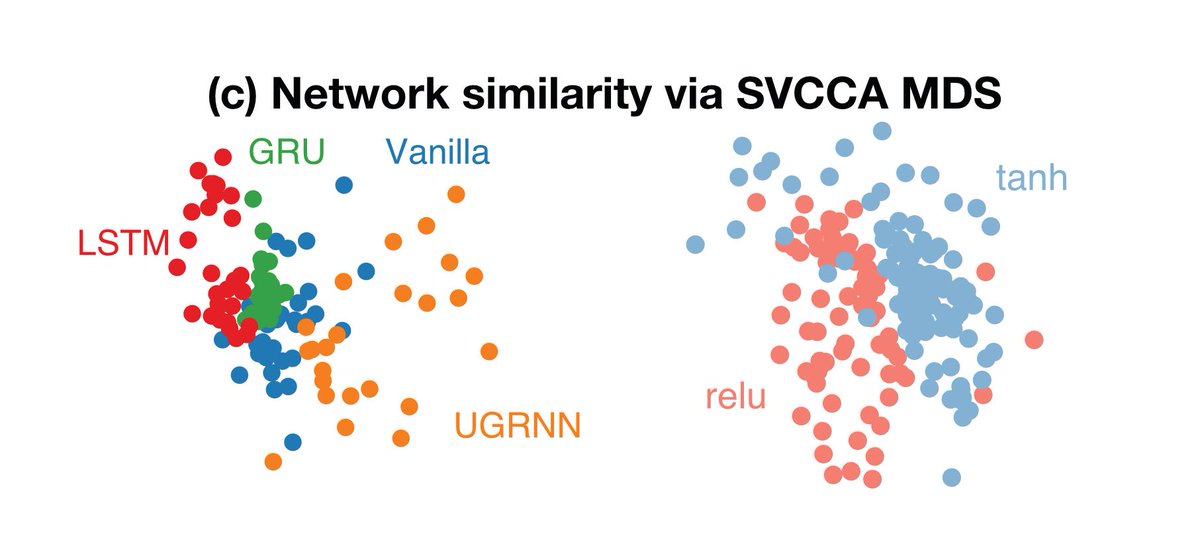
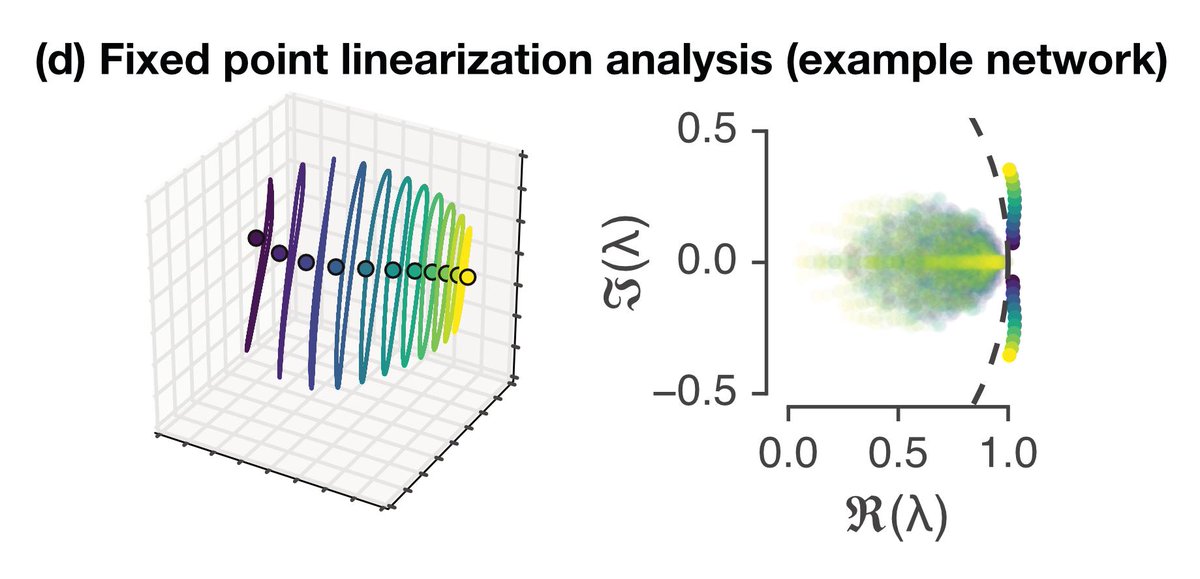
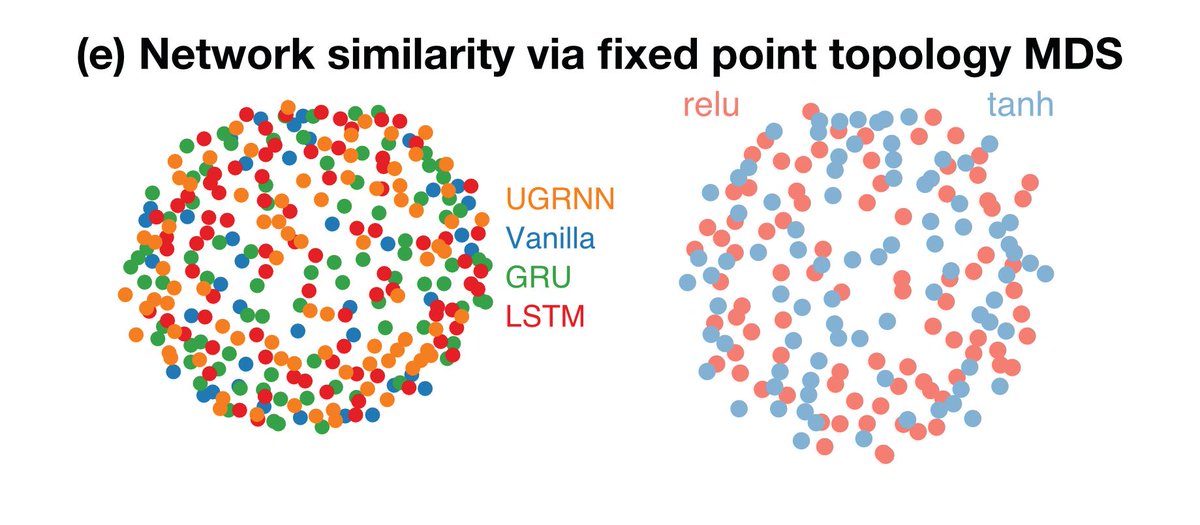
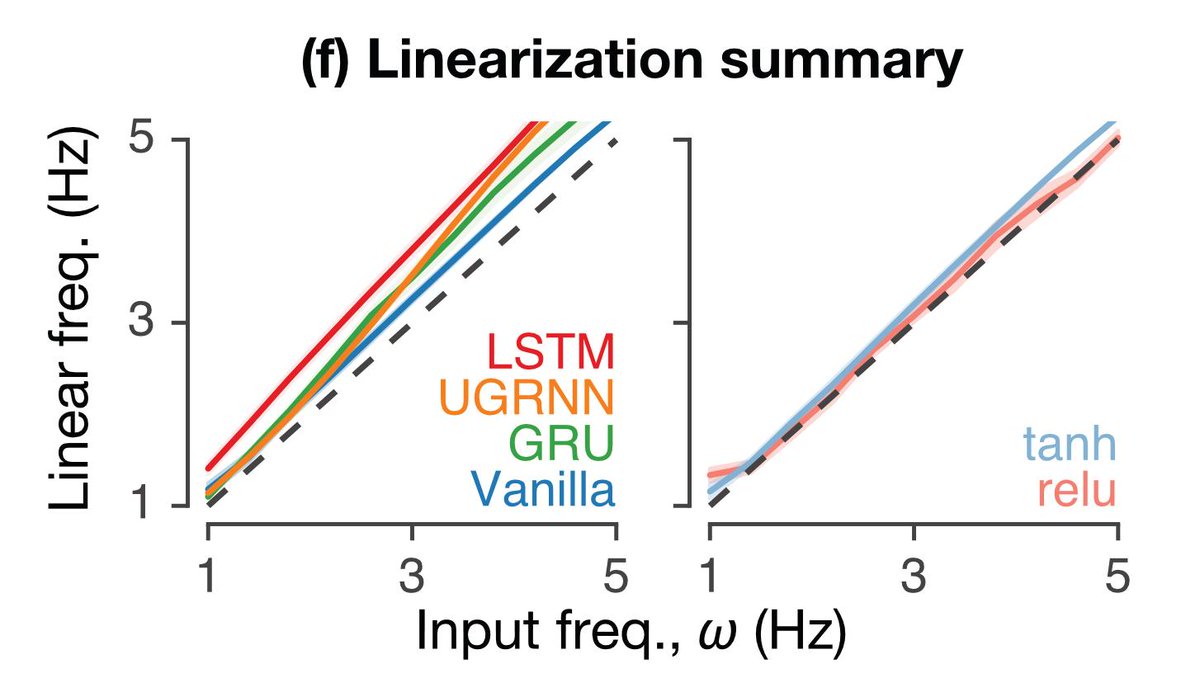
Get real-time email alerts when new unrolls are available from this author!
Twitter may remove this content at anytime, convert it as a PDF, save and print for later use!
1) Follow Thread Reader App on Twitter so you can easily mention us!
2) Go to a Twitter thread (series of Tweets by the same owner) and mention us with a keyword "unroll"
@threadreaderapp unroll
You can practice here first or read more on our help page!