E(Z1 Z2) = sqrt(N1 N2) rg li / M + stuff
(N1 and N2, the sample sizes, and M, the total number of SNPs analyzed, are known.)
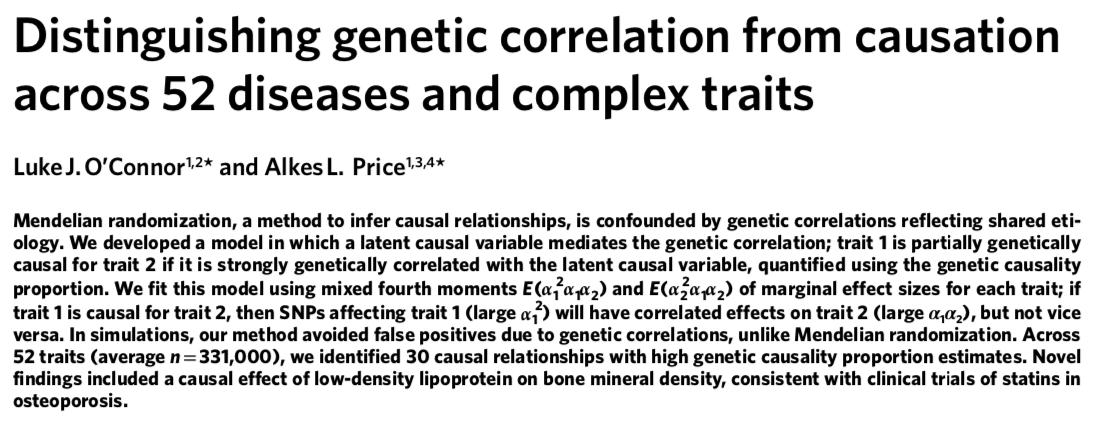
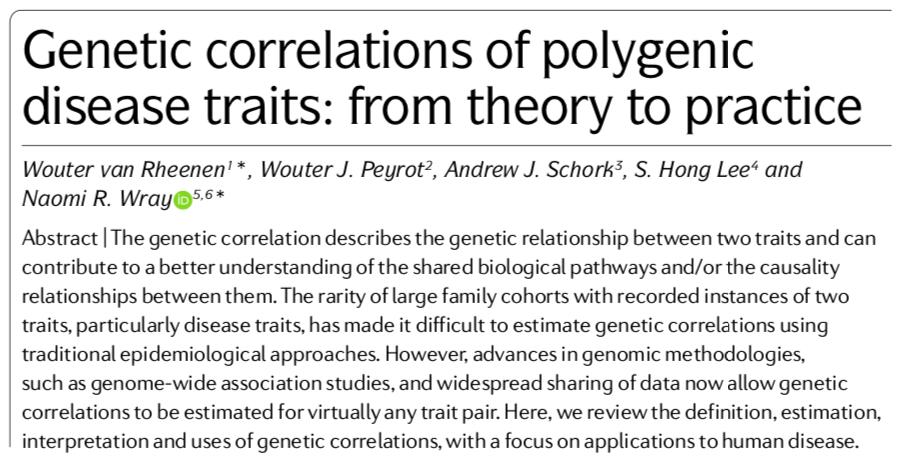
Get real-time email alerts when new unrolls are available from this author!
Twitter may remove this content at anytime, convert it as a PDF, save and print for later use!
1) Follow Thread Reader App on Twitter so you can easily mention us!
2) Go to a Twitter thread (series of Tweets by the same owner) and mention us with a keyword "unroll"
@threadreaderapp unroll
You can practice here first or read more on our help page!