Implementing experiments that are poorly designed. 🔬
📊Study doesn't have enough power to call meaningful differences statistically significant.
👎Includes concluding that the null hypothesis is true - should be "not enough evidence to say that the null is false".
🎣 Fishing for significant results.
In plain terms: performing several different hypothesis tests on the same data set, but only reporting on the ones that worked.
Note, too: if alpha=0.05 and you perform 20 tests on the same data, you'll likely make 1 Type I error.
⚖Spurious correlations!
And heck, this one is so well-known it even has a website. 😁
tylervigen.com/spurious-corre…
In plain terms: overstating the results of an observational study. Saying that one variable "caused" another instead of is "associated" or "correlated". 📈
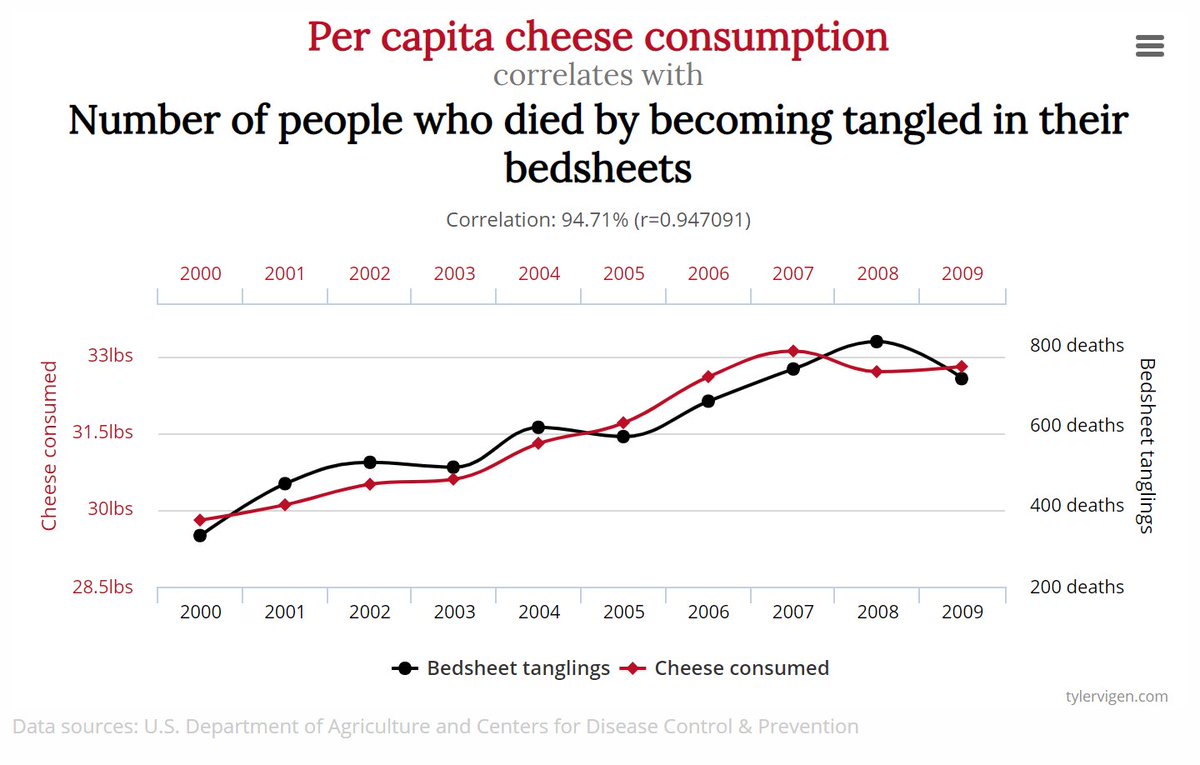
Use known best practices when designing and implemting your experiments.
🎲Randomization = assign by chance, not by choice
⚖Blinding = mask information from participants+tester
👩🔬Controlling = minimize the effects of variables other than the independent variable
If it was, will try to do more of these tweet-storms for machine learning and machine-learning-adjacent topics (linear algebra, statistics, etc.)..
Always remember to keep your course notes! And huge shout-outs to Dr. King, @hodgesse, et al. 📊