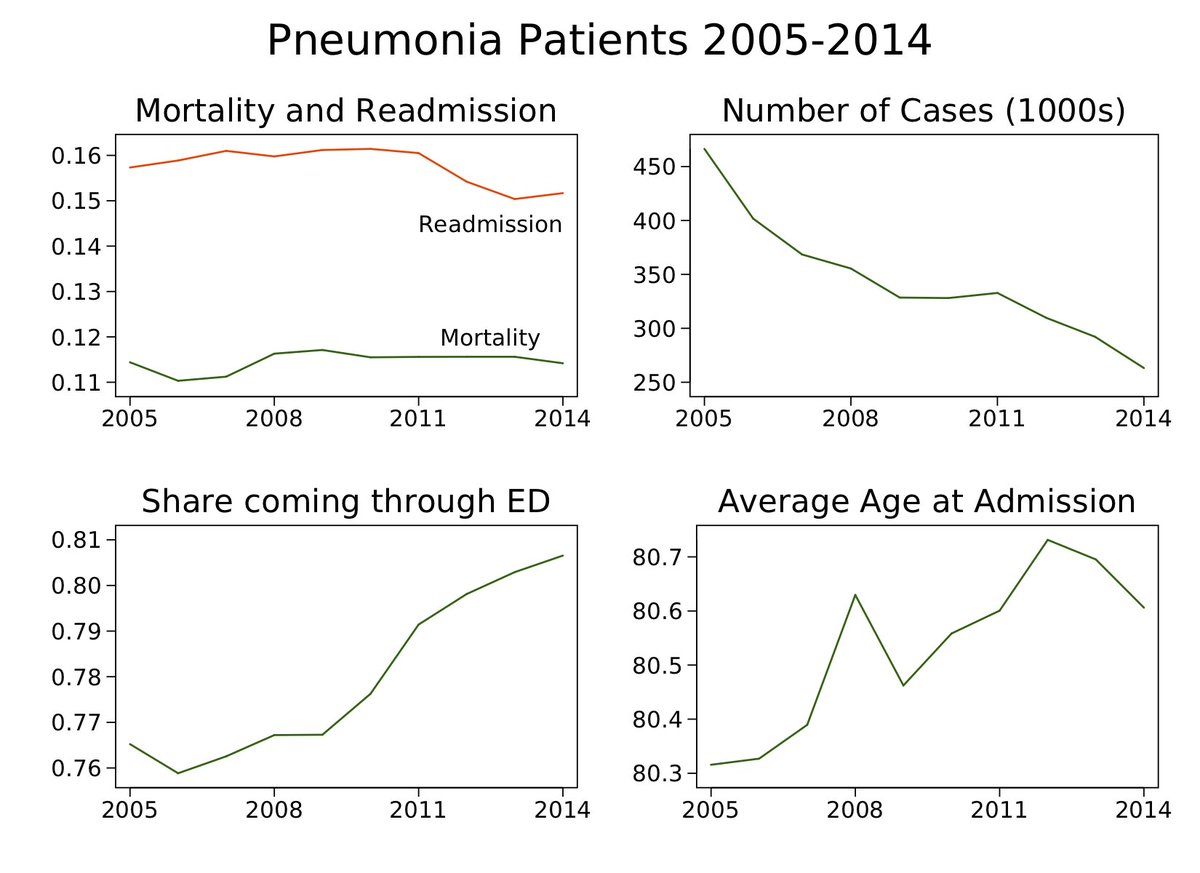
1) is the counterfactual sensitive to how one models the trend?
2) are there signs that confounding factors are changing?
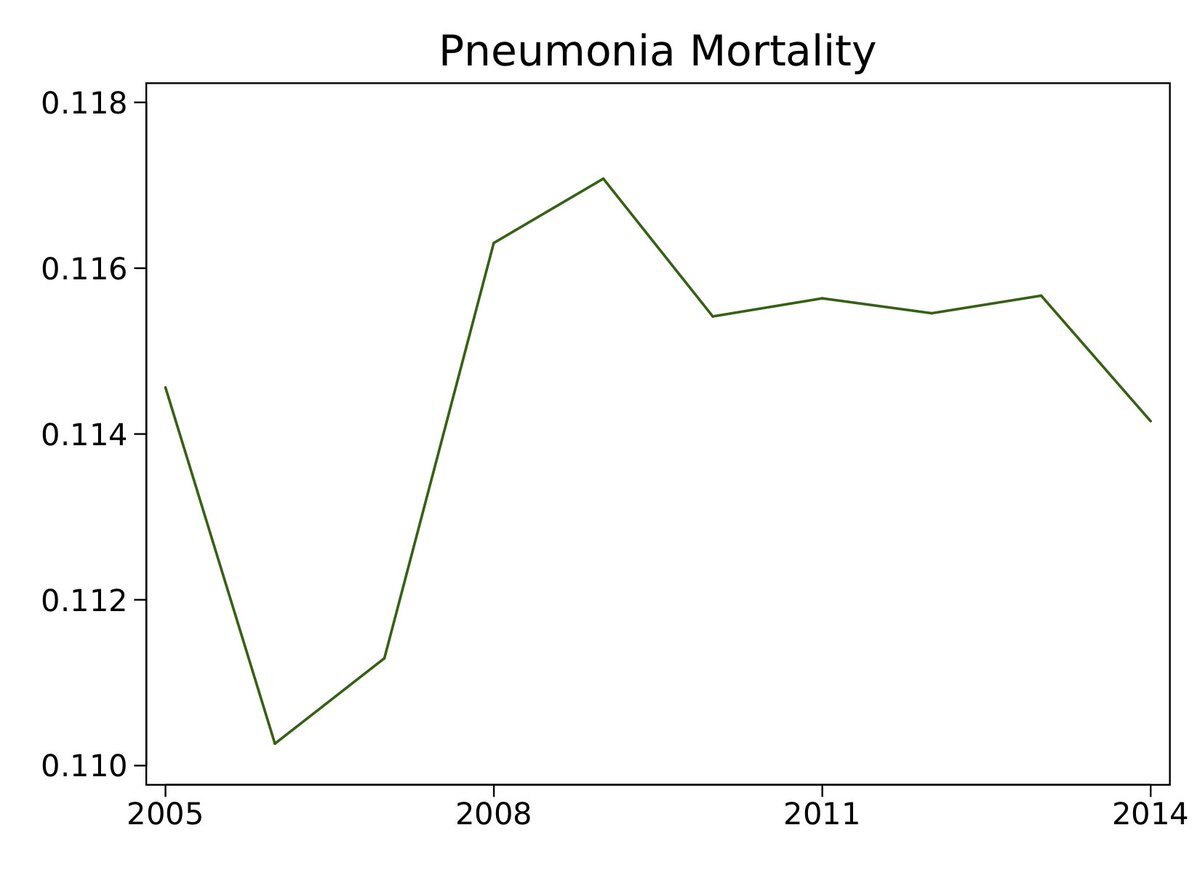
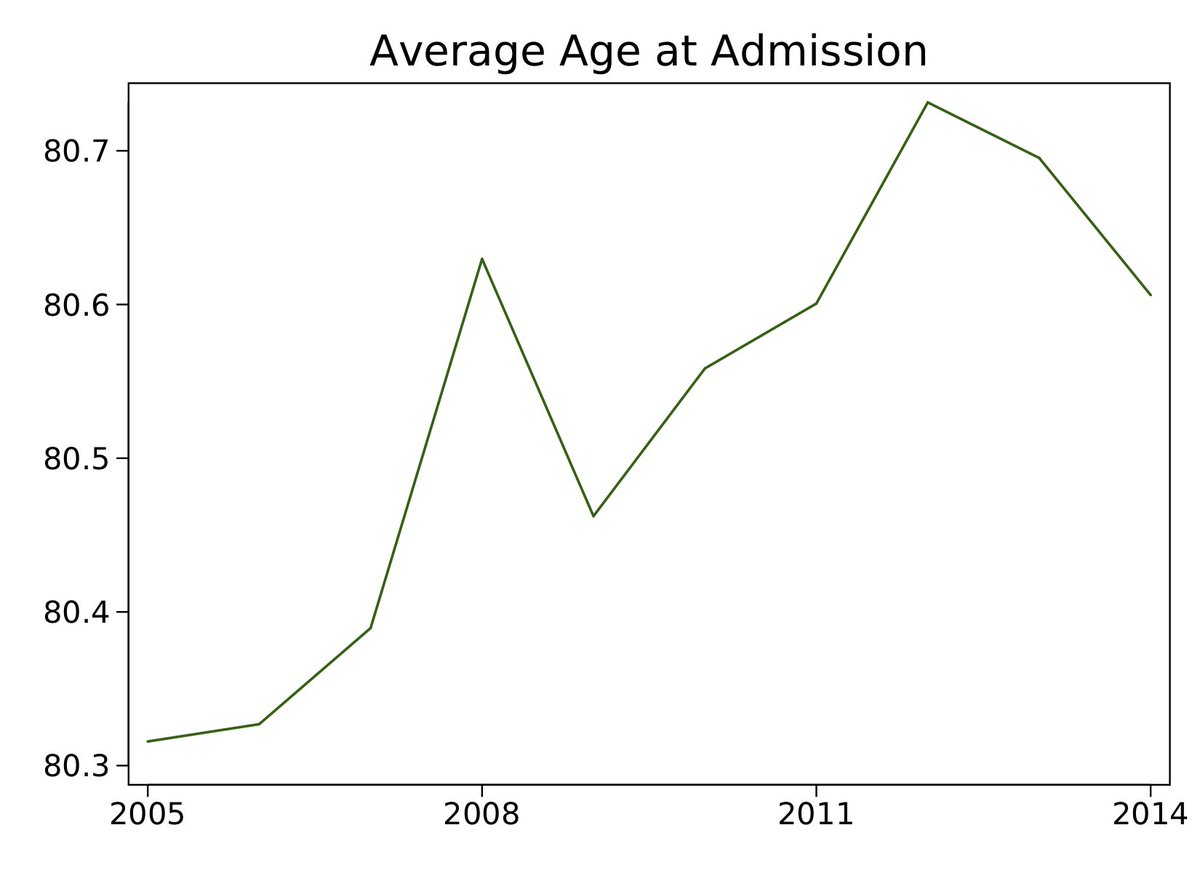

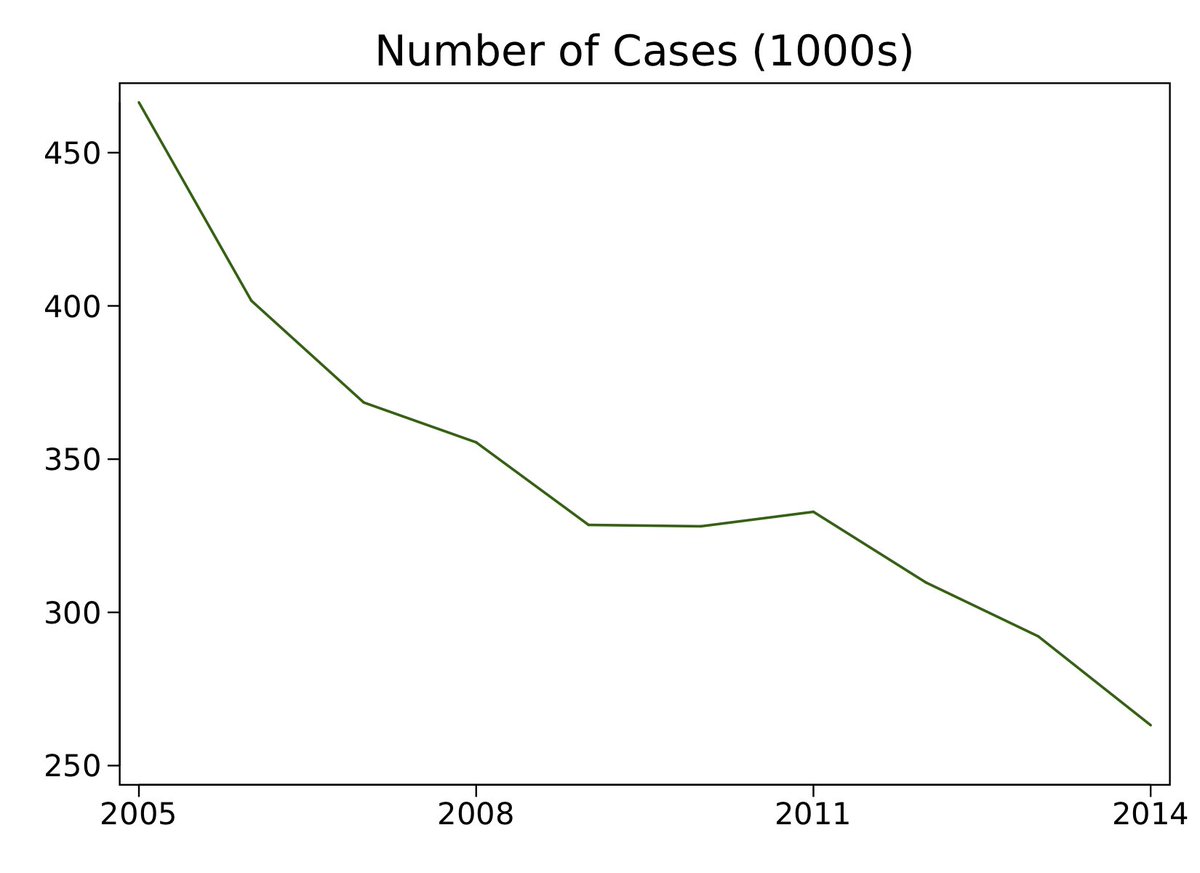
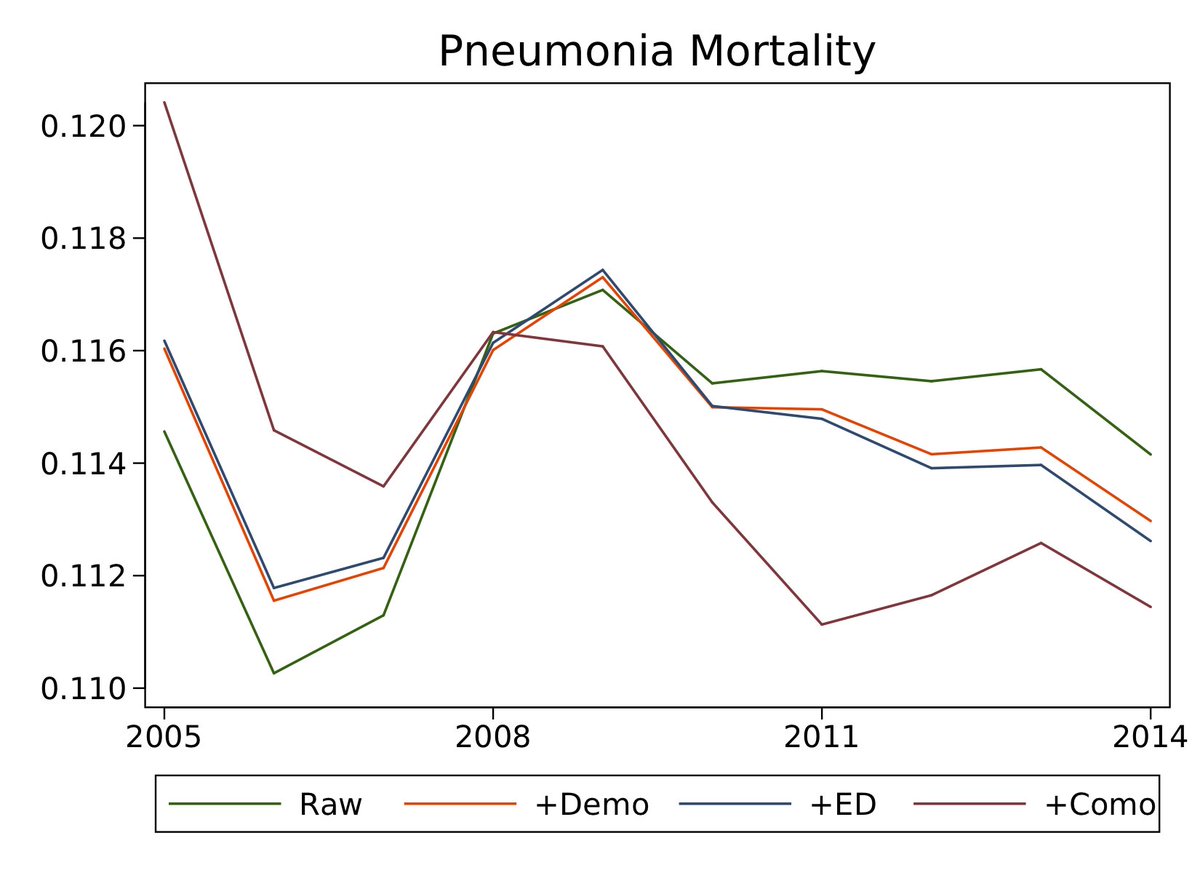
see @rkwadhera nejm.org/doi/full/10.10…
and Finkelstein & Taubman science.sciencemag.org/content/347/62…
* any faults of this analysis are my own!