@ml_barnett @andrewolenski & i are doing a study where we already know our confidence intervals but have no idea what our point estimates are! and here’s why i think that’s so cool.
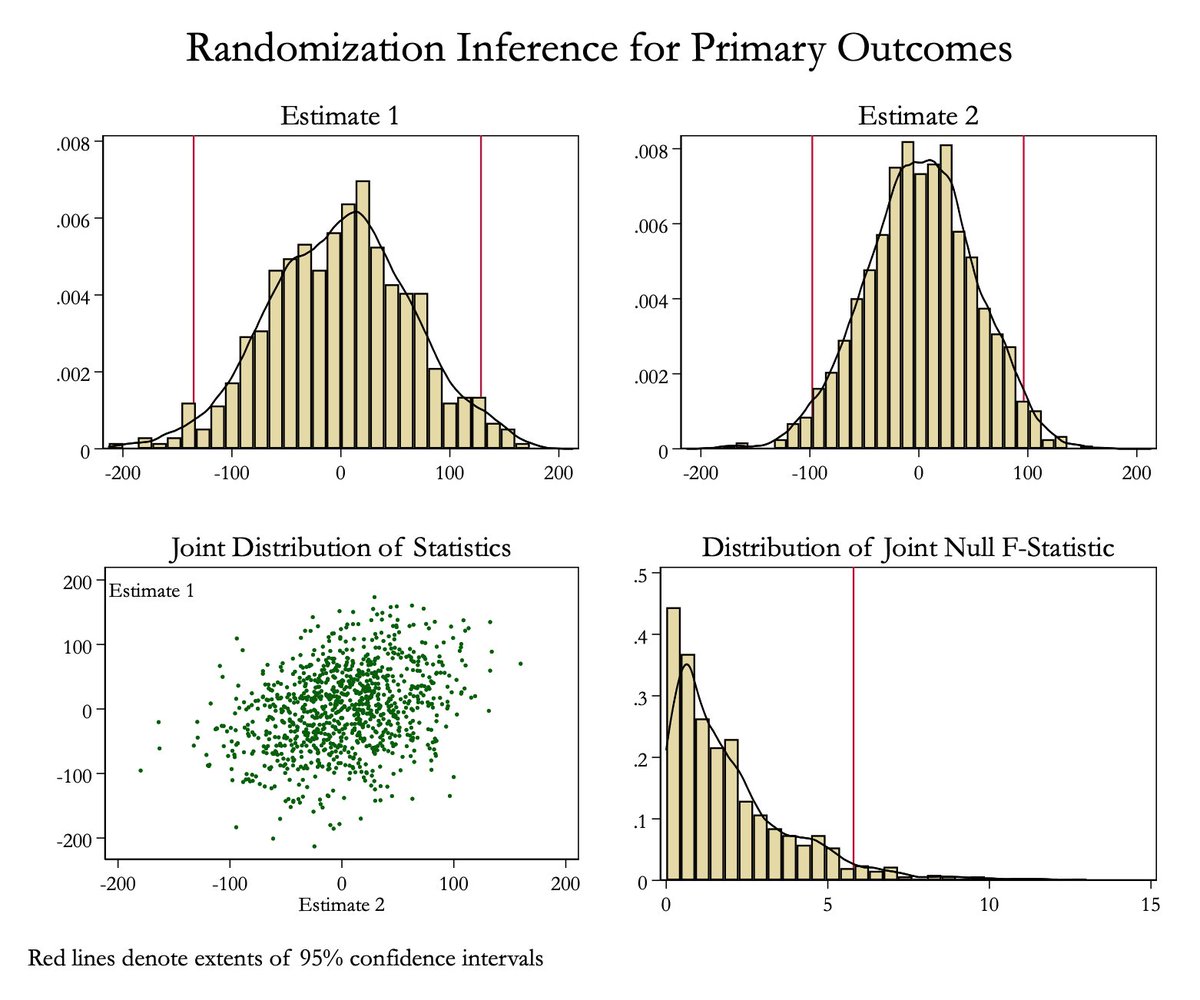
now we're studying if the letters had peer effects, i.e. did they affect doctors who worked with the ones who received a letter?
for (1), we loaded up our data and blanked out the true treatment status. this is called blinding. it’s hard (~not impossible) to p-hack when you don’t see the variable of interest.
then, we pretended these randomizations were real and estimated, 1,000 times, the supposed treatment effect of having a doctor peer get a letter.
it’s weird to already know what we’re up against.