For the journalists etc. attempting to understand what’s going on behind the scenes at large social media companies wrt censorship, there are a few conceptual tools you must equip before making statements in either support or suspicion...
Most of these approaches analyze the category of first-order signals to which the public has access...
But this is a fool’s errand.
You will never get to the heart of what’s either happening, or not happening, by analyzing these data points.
Why not, you ask?
Well the superficial answer is plausible deniability...
The show is not the trick itself.
To understand what tricks may be in play, we must briefly detour through the land of Network Theory...
This is especially true when it comes to how information flows through the network in question.
For our purposes we’ll focus on power laws...
Now, one key thing to understand is that within scale-free networks, hub-users act like signal amplifiers, rapidly spreading the content they decide to share...
Circling back to the beginning of the thread, this is what people like @timcast have tried to detect.
But this is exactly what a magician hides...
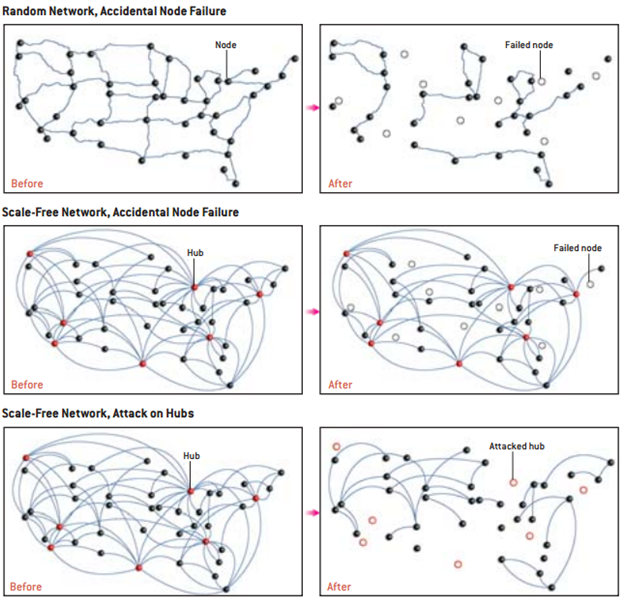
Alter what hubs see, but more importantly: alter what they *don’t see*.
Now we may subtly change how content flows the network without detection by crude analysis...
Because you’d need to know how each person’s feed has evolved across time, then subject the data from those feeds to some very advanced network-statistical analysis.
This is why we shouldn’t expect journos to detect such patterns...
This phenomenon is known as “self-organized criticality”, and is contributes to the virality and addictiveness of social media increasing across time...
The learning systems will then need to be fed many examples of such “hateful” content in order to be capable of detecting it w/o aid...
Who decides the definitions of concepts such as “hate” used to train these learning systems?
And at present, the answer is:
Whoever writes the code, or manages those that do...
Those who obsess over bias in other spheres are most likely to encode their own here...
- You're not going to detect these changes from the outside, using unsophisticated approaches.
- This kind of "network flow management" is all around you...
Until we ensure the transparency of the processes and algorithms by which our information flow is managed, we will continue to witness the emergence of tools more powerful than humanity has ever known, capable of changing thought patterns at scale...
We're now allowing algorithms to establish the boundaries of acceptable thought..
"When fascism comes to America, it will be wrapped in the flag and carrying a cross."
But at present, it appears far more likely that our contemporary thought police will cloak themselves in dopamine-fueled clicks and carry a Network Science textbook.