Since #AcademicTwitter is great for open discourse, & bad at keeping all in 1 place, I thought I provide this public good..
1/19
From an econ perspective, a good start to get acquainted w/ the idea behind DAGs may be @yudapearl's #BookofWhy or this book amzn.to/2WuWf7d.
(Disclaimer: Haven't read them yet.)
2/19
As a 1st approximation, I found @PHuenermund's and @juli_schuess's slides helpful; to be found here: bit.ly/2uxW3Ze & here: bit.ly/2uwnGBK.
3/19
1st, use formal language of graphs to articulate knowledge abt causal relationships.
2nd, you evaluate the identifiability of the outcome w/ all hands on deck re: assumptions.
4/19
Collider bias & M-bias.
5/19
@cdsamii notes, Heckman's selection problem is just cond on a collider's descendant: bit.ly/2Ue7tzB (p.19).
7/19
As best as I understand, bias introduced by conditioning on a pre-treatment covariate in the presence of a particular M-structure: between a treatment, an outcome, two latent factors, & the covariate as "collider".
(Pic, HT @EpiEllie)
8/19
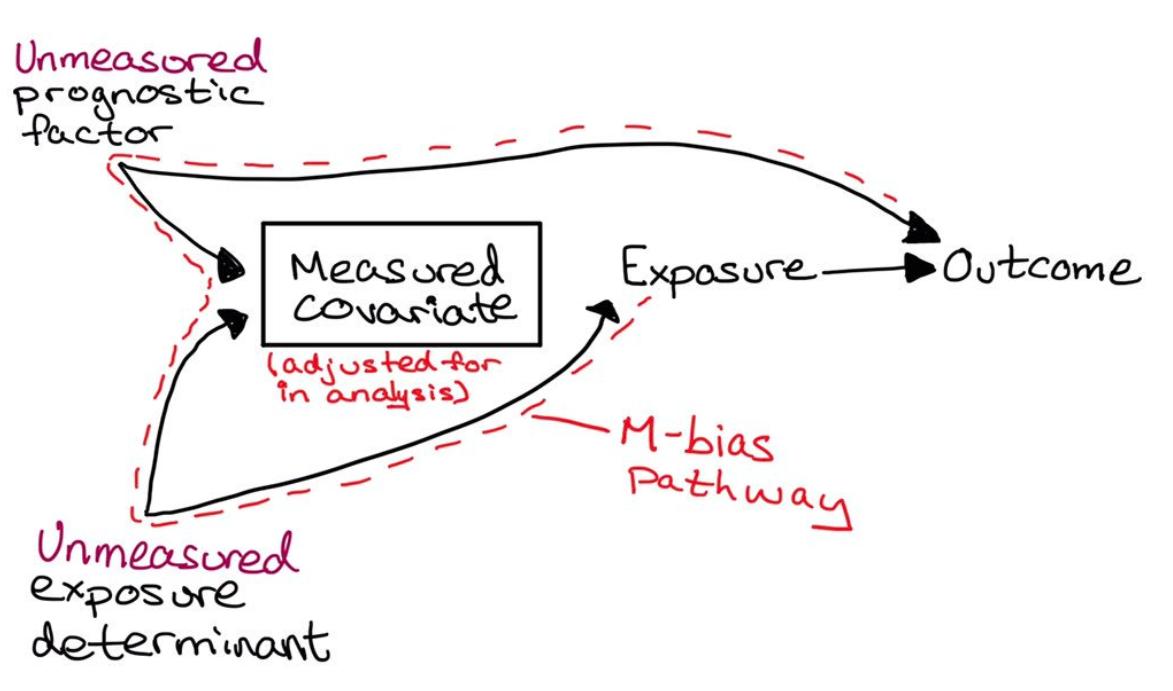
(Helpful explainer of R package ggdag to play around w/ DAGs: cran.r-project.org/web/packages/g….)
9/19
This allusion by @autoregress to Frisch-Waugh is a helpful econ example: .
10/19
i) DAGs help clarify biases in an intuitive way!
ii) DAGs are helpful in teaching causality w/out the need of abstract math.
ii) DAGs can be helpful in checking the assumption of a model that has been built prior by use of contextual knowledge.
11/19
i) DAGs are useful, & that would be enough for paper to be seen as novel. But to make it part & parcel of syllabus is quite another thing. So, what are ways in which great econ papers are flawed that DAGs help resolve? (Along the lines of @Jabaluck)
12/19
13/19
14/19
16/19
@cdsamii had a fascinating application of the collider problem to FEs in panel data, here:
17/19
Happy to stand corrected if not.
18/19
1. I'll read much more on this
2. #AcademicTwitter is the best Twitter
3. I'm massively indebted to all the ppl linked above for their input
4. I now follow a couple ppl on #epitwitter
5. There's a thing called tough love and econs are really good at it..
/FIN