used PS with caution instead of banning is a solution. In the next tweets, I’m going to specify some critics on PS methods.1/
1) PS matching
2) Stratification
3) Inverse probability weighting
4) Multivariable regression using PS as a covariate 4/
1-PS deal with confounding not outcome heterogeneity
2-matching algorithm is possibly arbitrary and not reproducible
(7/)
4-many PSM user do not take interaction/non-linearity into account
5-many PSM user ignore the non-collapsability of OR/HR (8/)
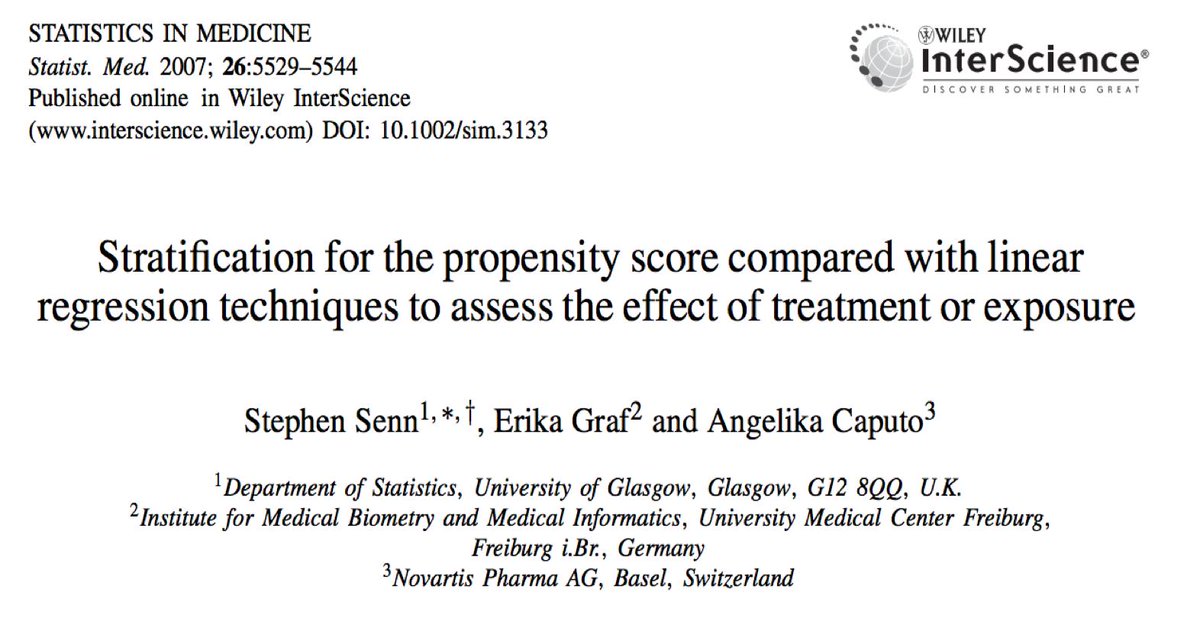
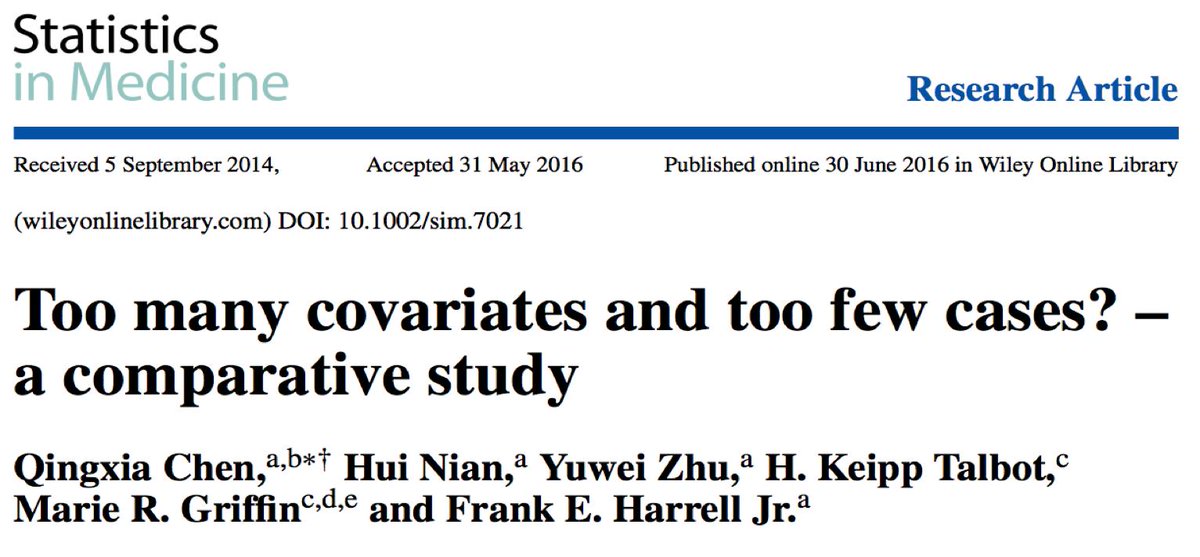
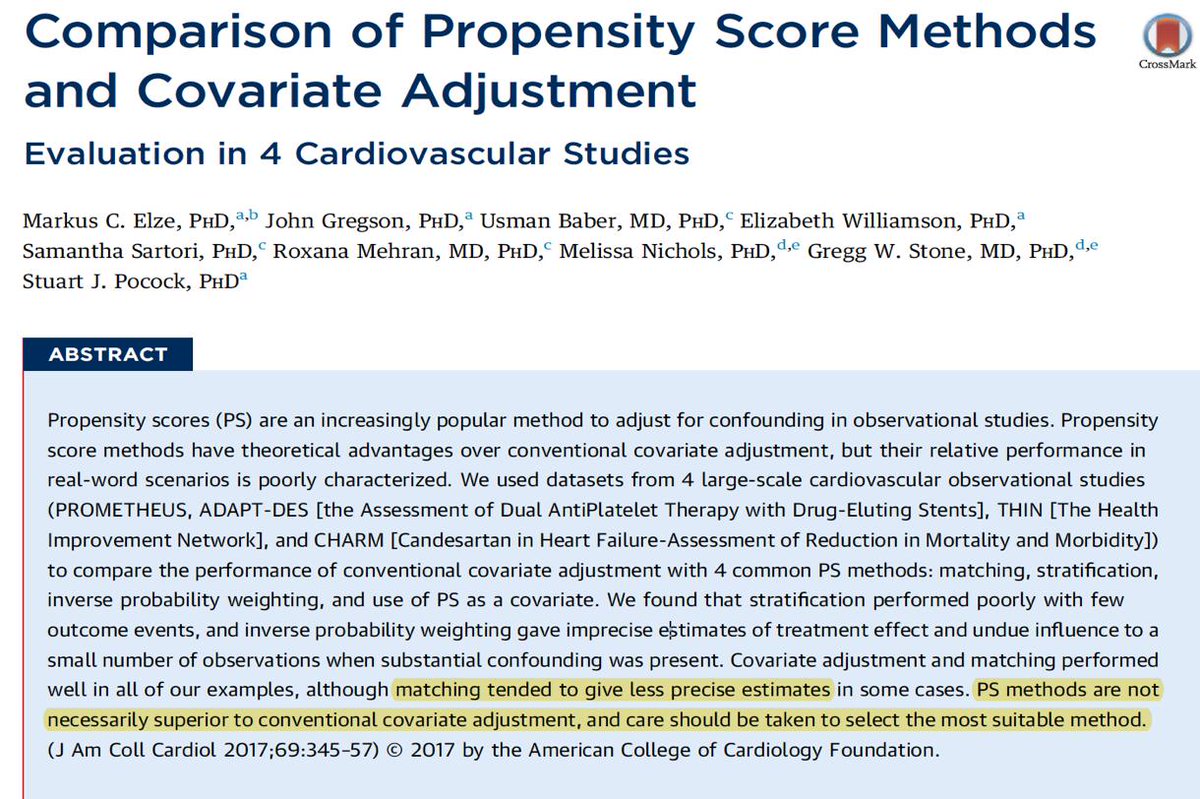
1-Select large list of potential confounder variables to generate PS (gray).
(12/)