Today’s topic is the Target Trial Framework for #causalinference and how to apply it to improving observational studies.
#epiellie
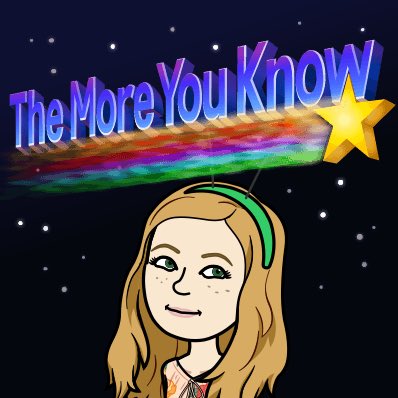
Well it’s not a new method! Instead think of it as pedagogical device that provides a structured way to build your research question and study design for observational studies and minimizes the potential for bias.
Plus, the target trial needs two protocols — the trial you wish you could do, and the observational study you can do!
Our August issue has this great paper by @MissLodi, @_MiguelHernan, et al. which uses an actual trial as a starting point for the target trial, & then tries to emulate this as closely as possible from observational data.
academic.oup.com/aje/article/18…
•different inclusion/exclusion criteria
•different target populations
•different treatment strategies
•different causal estimands!
So let’s dive into the paper!
(expand for poll)
An observational study using the HIV-CAUSAL database reported a 7-year (per-protocol) risk ratio of 0.66 (0.6,0.8).
1) harmonize study protocols using the actual trial as the guide for the target trial
2) harmonize data analysis to estimate same measures & target parameters
3) sensitivity analyses for mismatches
Randomized trials can potentially allow estimation of intention-to-treat and per-protocol effects. But intention-to-treat effects don’t make a lot of sense in observational studies, and we mostly want per-protocol effects.
Have you ever used it or do you think you’ll try? (expand for poll)