Follow this tread for updates.
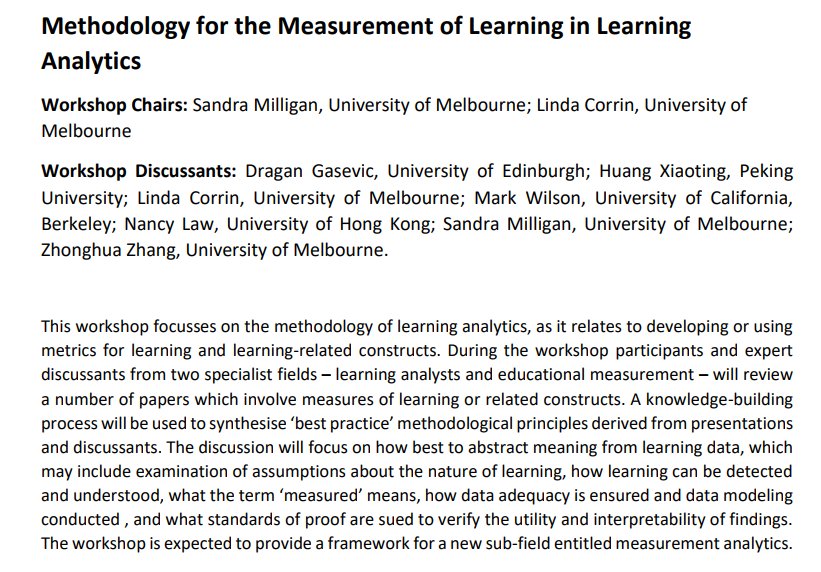
- how to get actionable analytics?
- issues of data triangulation
- what do we measure?
- what do we NOT measure?
- quantity/quality of data
- lots of real-life education *doesn't* have big data
- data =! evidence
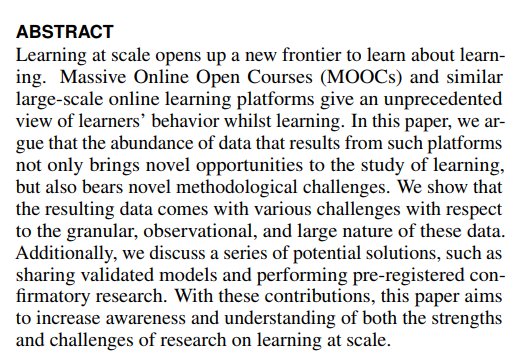
- predict writing quality & essay scores
- distinguish skilled from less skilled writers
- to determine boredom and engagement
- to assess mental ability
- to determine the tasks' cognitive load