(But really just using logistic regression)
I recently learned about Thomas Cover's game from @fermatslibrary & really liked it! Its a great example for learning about games, probability, ML & RL. Lets use ML to "beat" the game.
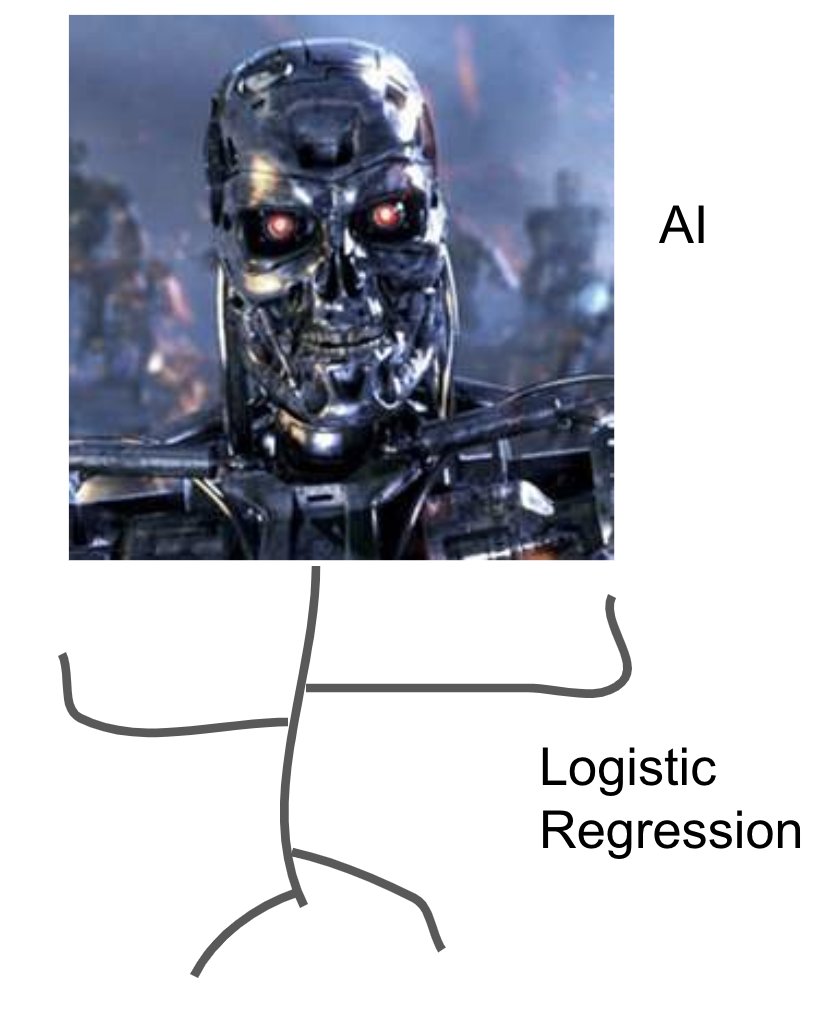
Mark picks two numbers and keeps them hidden from Sally. Sally randomly selects one of the numbers.
We will generate 2 random numbers from a random number generator & only show one of them to the classifier. If the other hidden number is bigger than the shown number, then this is a “positive” example. Otherwise, its a “negative” example.
- violation of statistical independence: Mark picks the two numbers strategically (eg: gonna pick 2 numbers super close!)
- what if instead of Sally picking the number, Marks selects which number to reveal?
- change the rewards: winning or losing for Sally has equal reward/loss. What if we change this? Make Sally lose more when she loses?
This is not just better than random, but also better than the strategy that Thomas Cover came up with!
See the Google colab notebook to get more details :)
colab.research.google.com/drive/1wyuWRGy…