thenewatlantis.com/publications/a…
Again, read Tafari Mbadiwe's supremely insightful @tnajournal essay: thenewatlantis.com/publications/a…
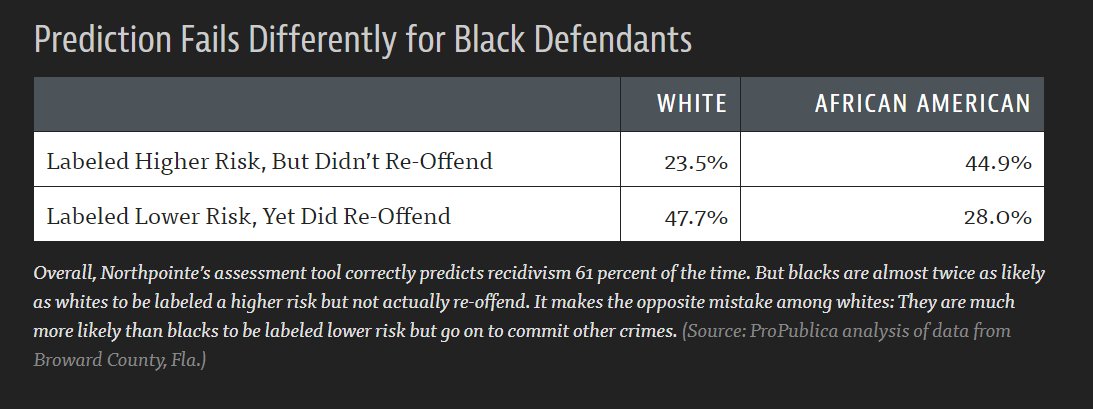
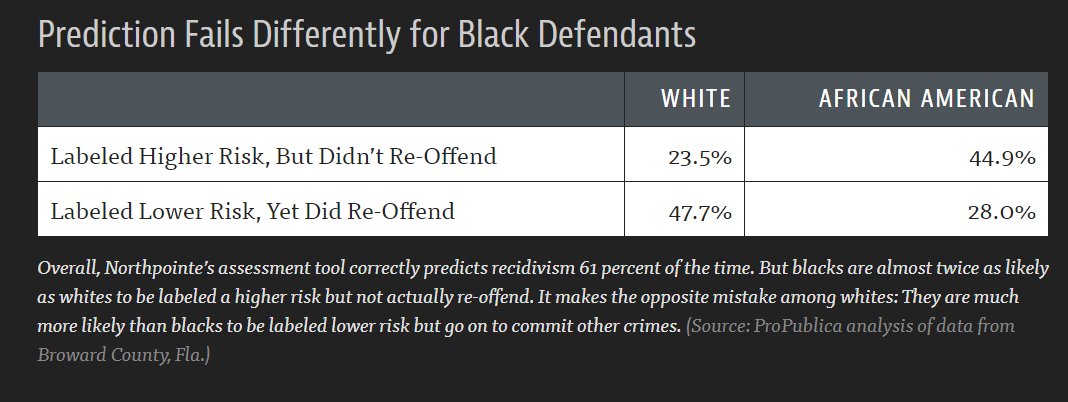
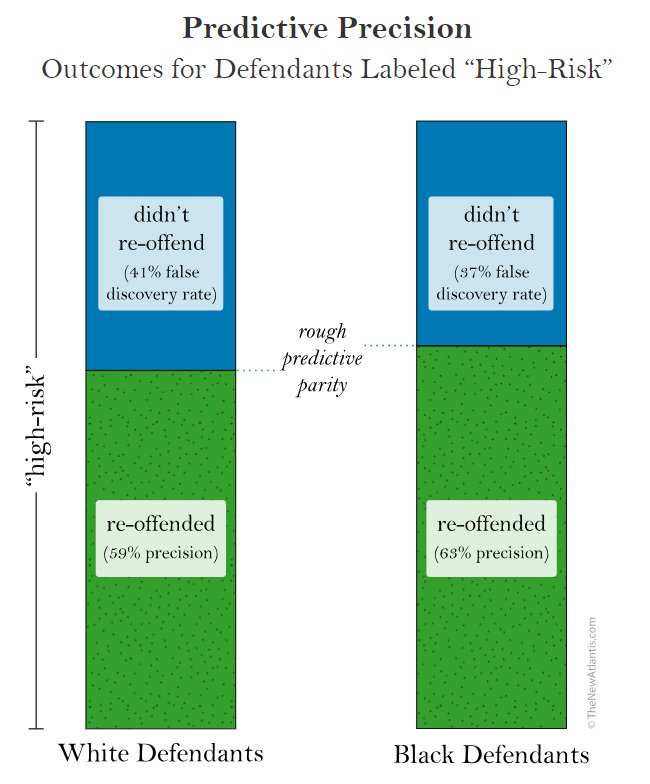
propublica.org/article/how-we…
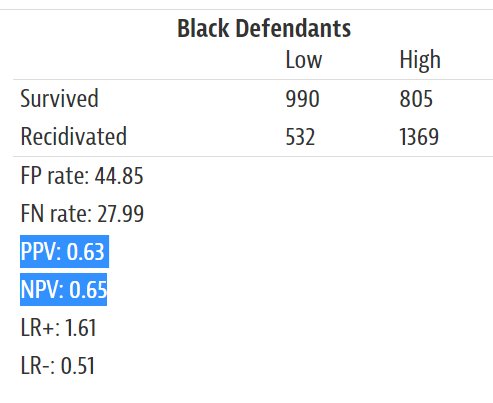
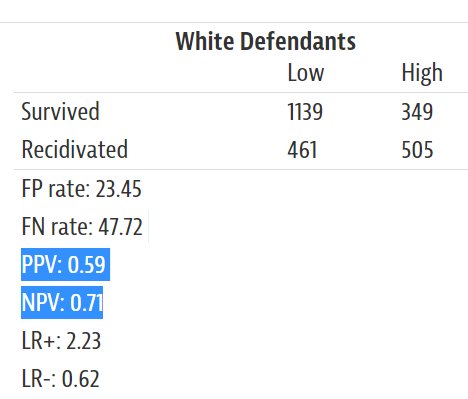
End thread — but read Tafari: thenewatlantis.com/publications/a…
Get real-time email alerts when new unrolls are available from this author!
Twitter may remove this content at anytime, convert it as a PDF, save and print for later use!
1) Follow Thread Reader App on Twitter so you can easily mention us!
2) Go to a Twitter thread (series of Tweets by the same owner) and mention us with a keyword "unroll"
@threadreaderapp unroll
You can practice here first or read more on our help page!