I'm very excited to share here with you new work from myself, @NeuroNaud, @guerguiev, Alexandre Payeur, and @hisspikeness:
biorxiv.org/content/10.110…
We think our results are quite exciting, so let's go!
- postsynaptic burst = LTP
- postsynaptic single spike = LTD
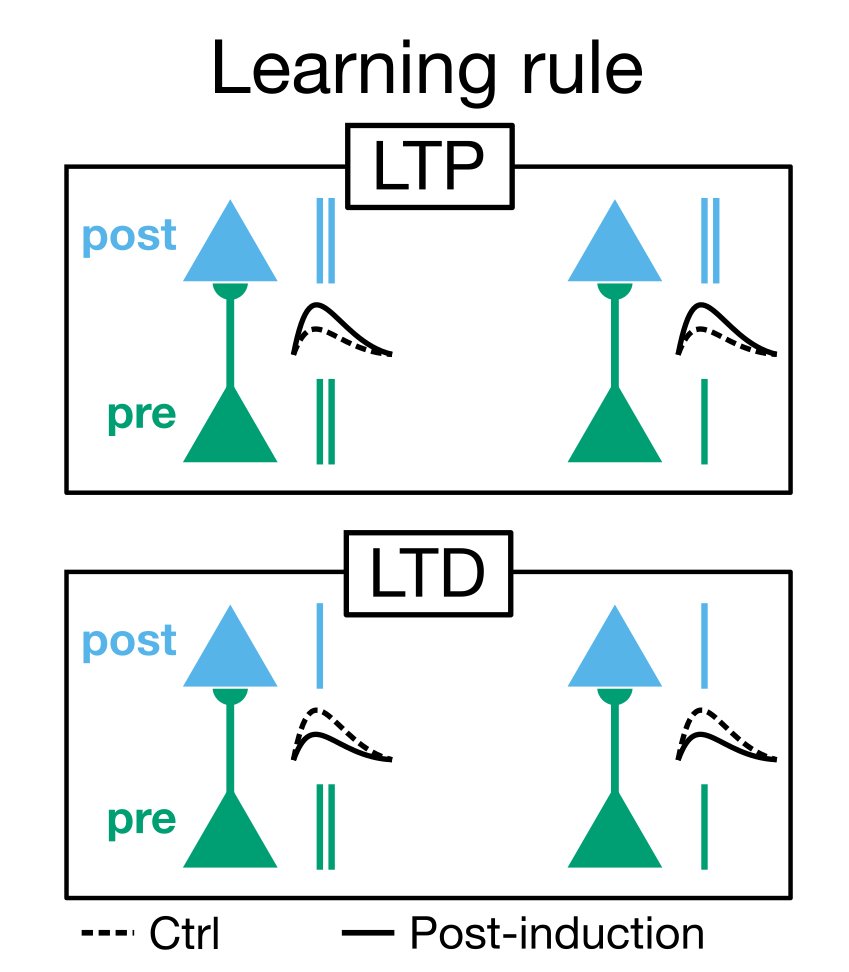
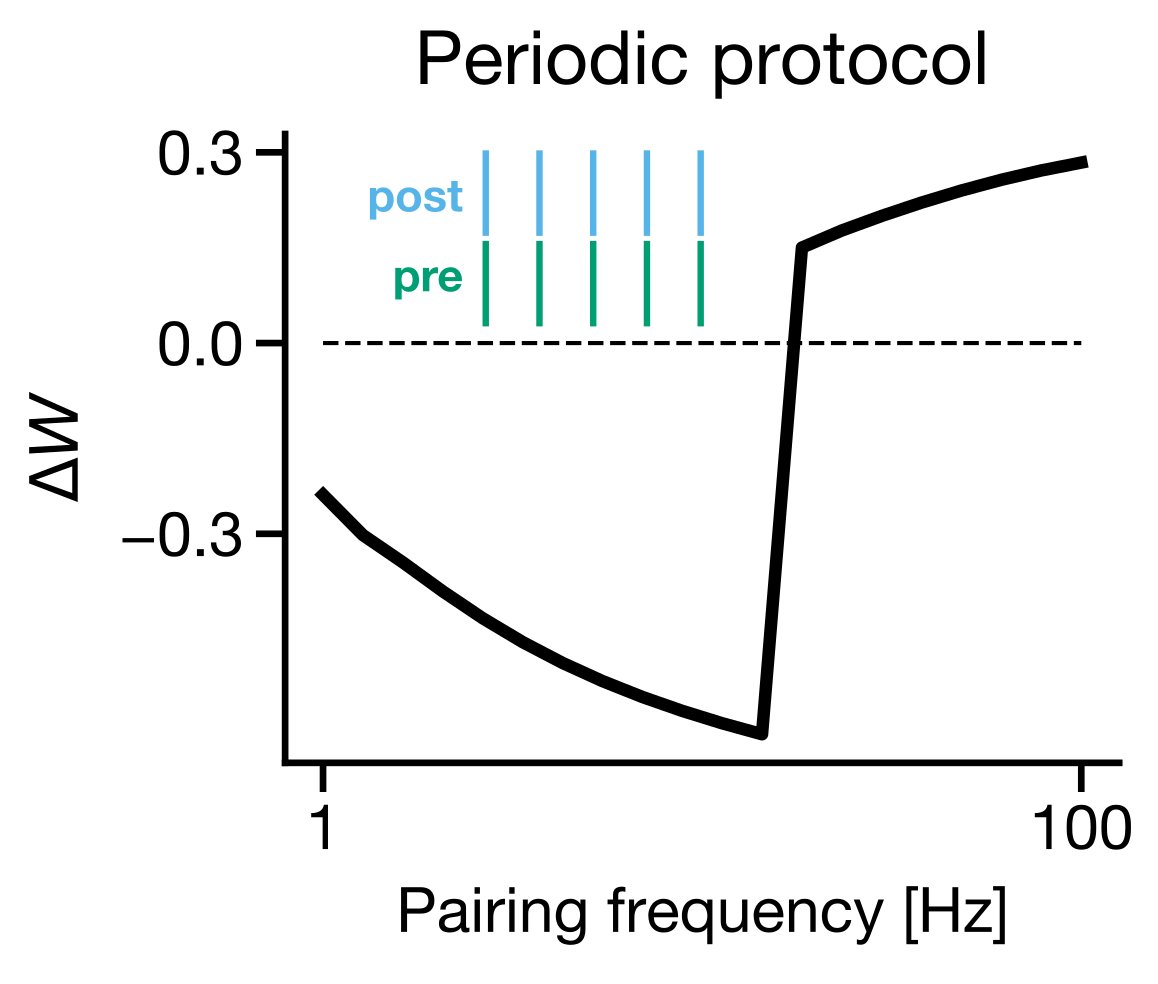
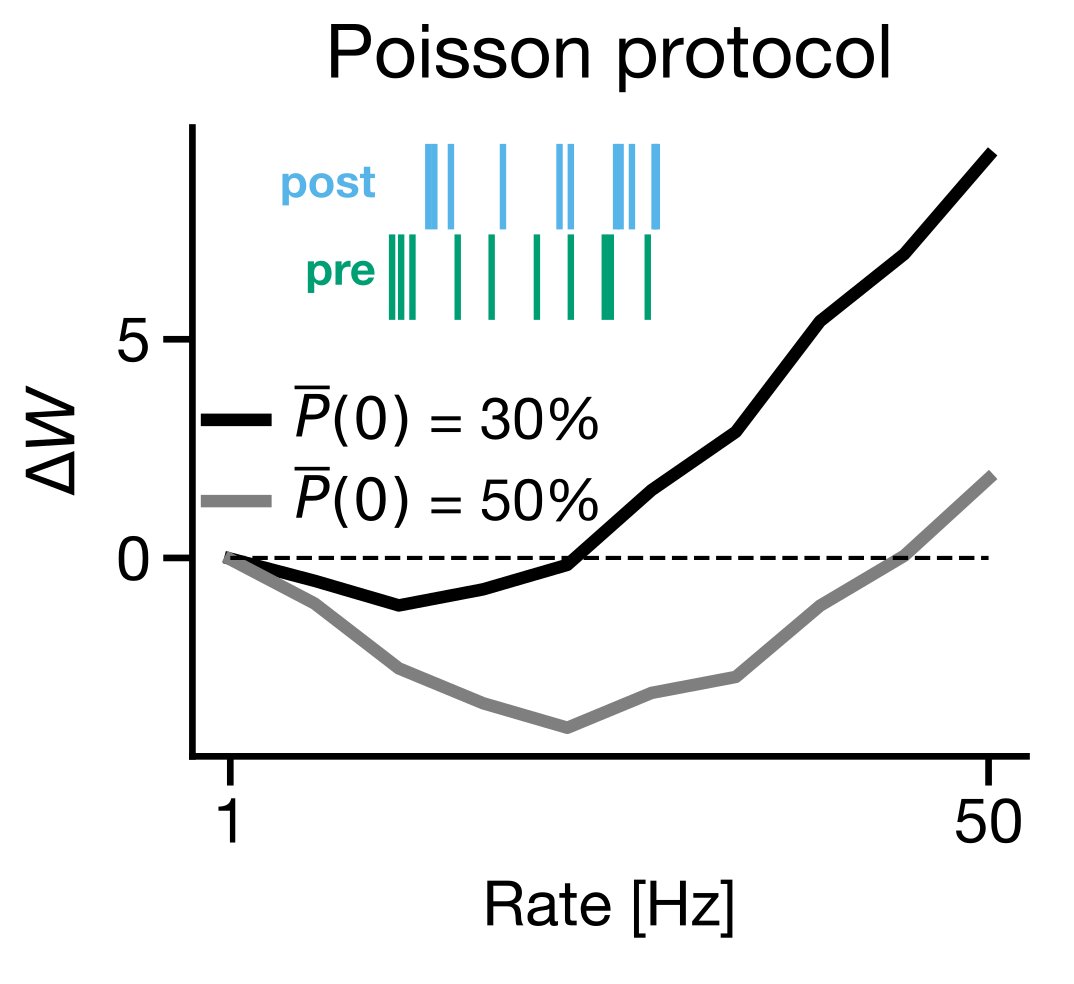
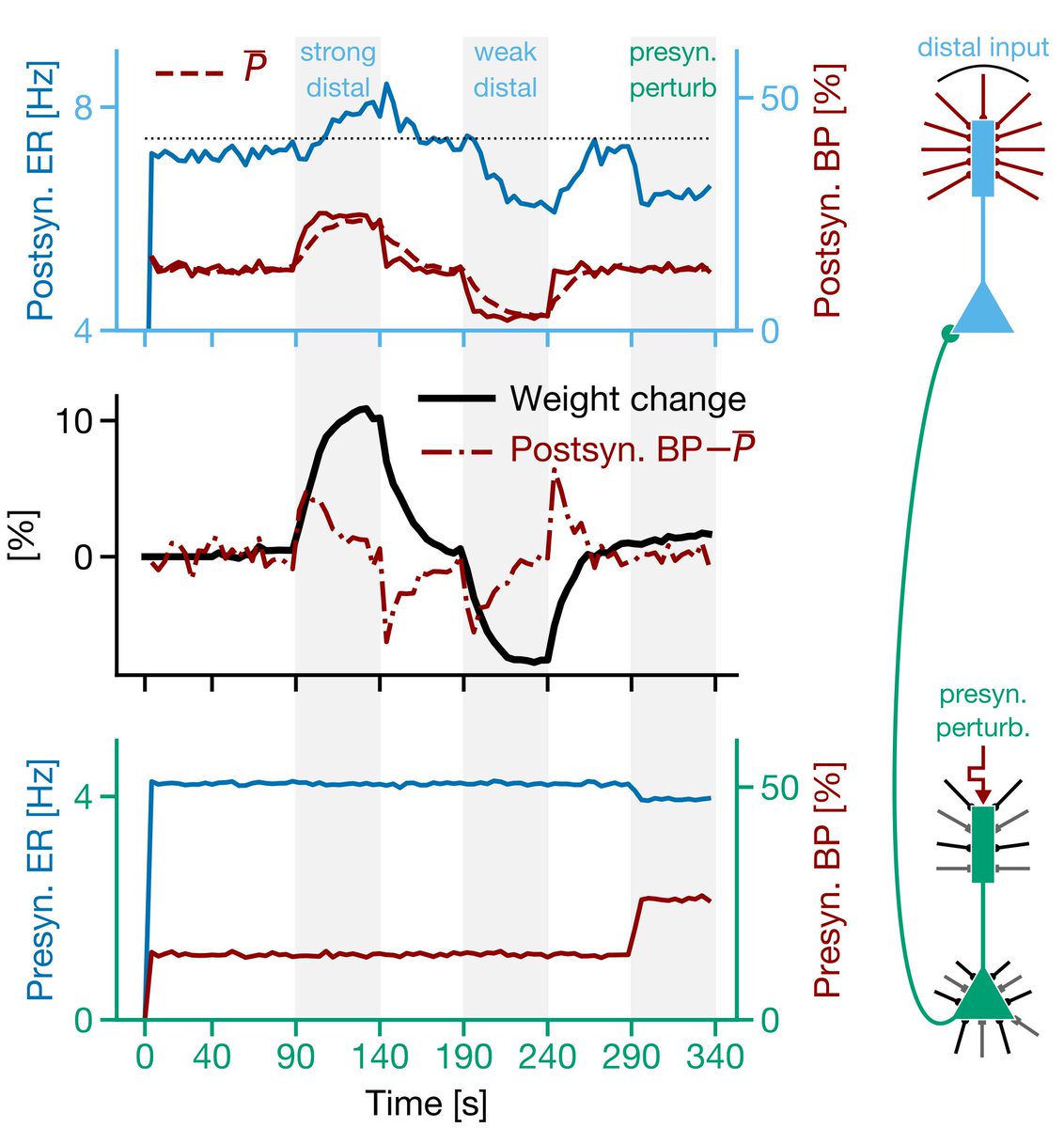
Turns out, if two conditions are met:
1) Burst probs are a linear function of apical inputs
2) FB synapses are symmetric to FF synapses
Then BDSP in the inf limit is gradient descent!
See e.g.: arxiv.org/abs/1904.05391
1) We propose a learning rule motivated by biology (BDSP) that can explain previous experimental findings and replicate other comp neuro algs.
papers.nips.cc/paper/8089-den…
Stay healthy and safe out there!!!