prisma-statement.org/Default.aspx
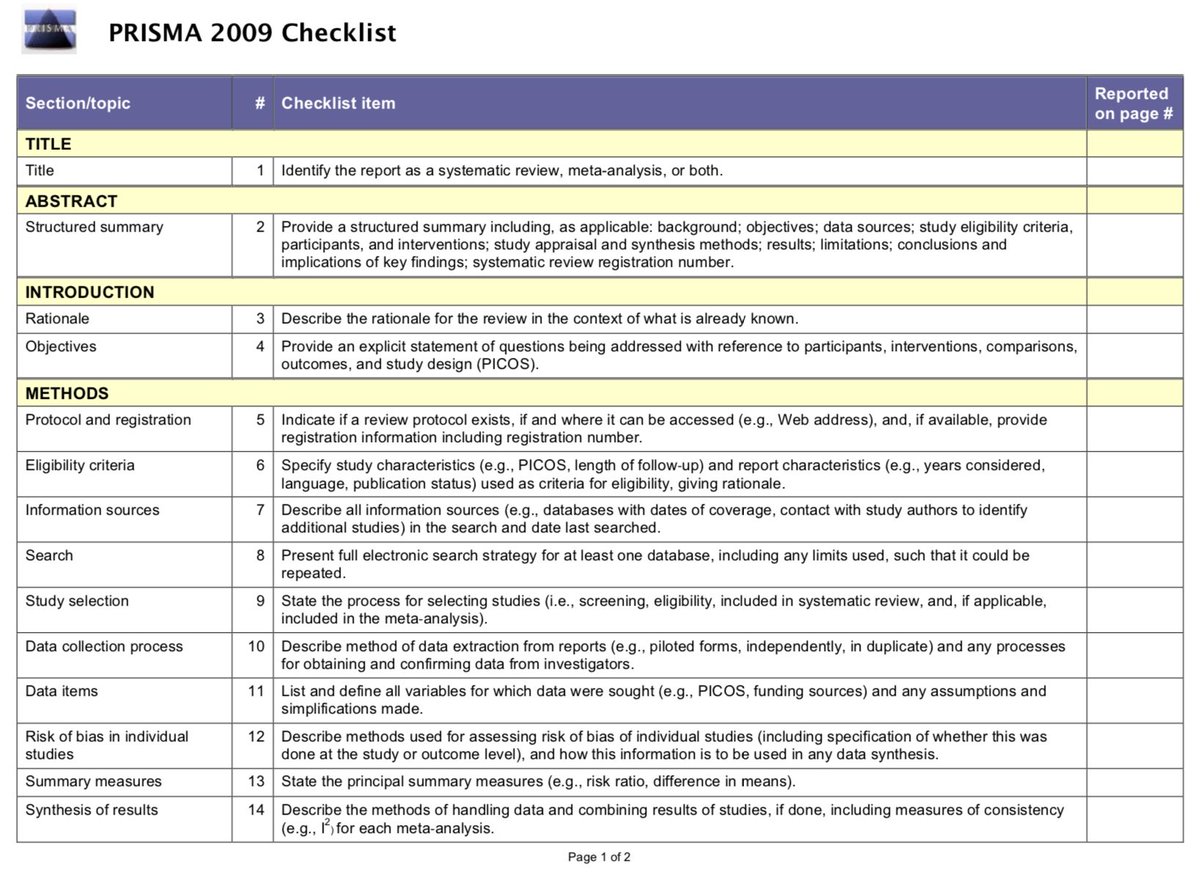
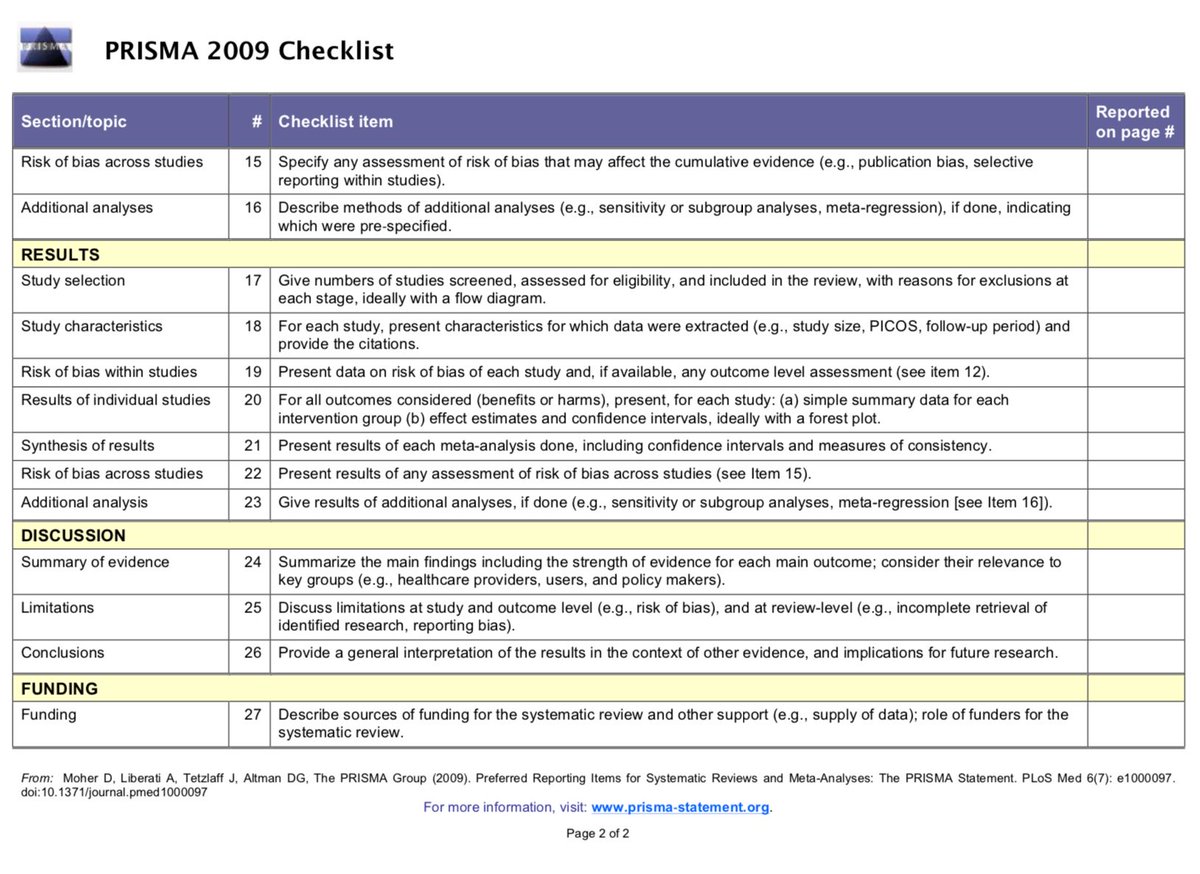
Asymmetry in funnel plots should not be used to make conclusions on the risk of publication bias. Check out what Peters and colleagues have to say on this ncbi.nlm.nih.gov/pubmed/18538991
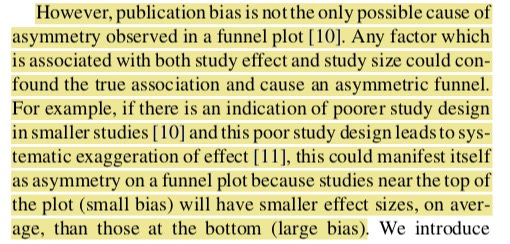
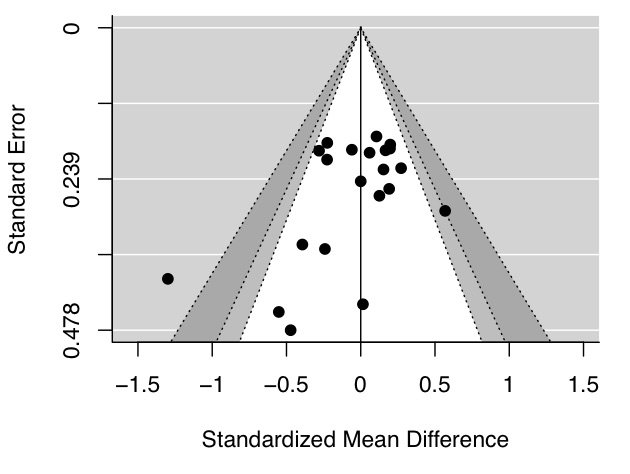
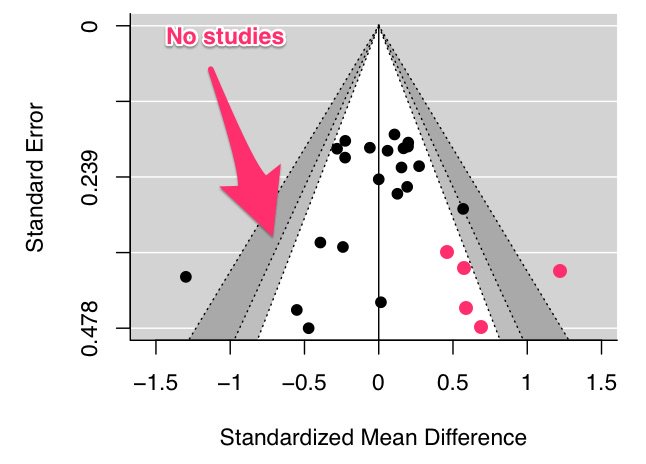
Ah, this old chestnut. This method adjusts a meta-analysis by imputing “missing” studies to increase plot symmetry. Here’s the method in action, with the white studies added in the right panel to balance out the plot
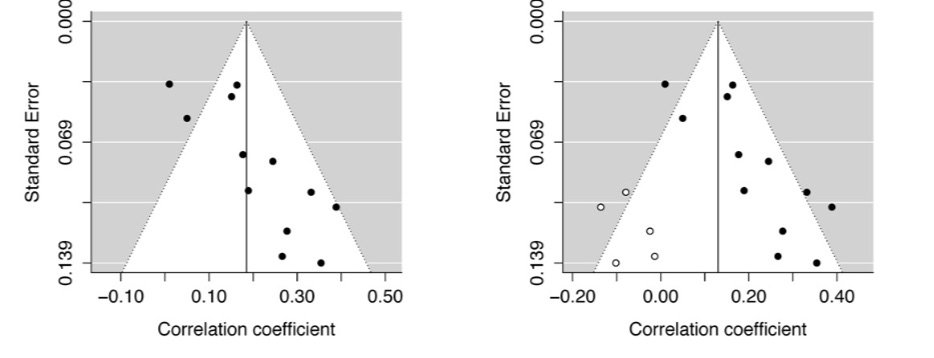
Two effect sizes extracted from the same study are statistically dependent, which can lead to inaccuracies in summary effect size calculation.
Unlike primary research, meta-analyses can be reproduced in many cases as summary effects sizes, and a corresponding measure of variance, are typically reported in a table.
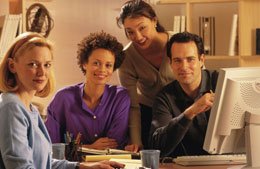
This often happens when comparing two summary effect sizes. You need to make a formal comparison rather then just relying on p-values.
If these aren’t adjusted properly, then some confidence intervals can be chopped off, which can inadvertently (or not) ‘hide’ outliers.
As far as I know there’s no gold standard test for outliers in meta-analysis (but check out the outlier function in metafor). But check out your forest plot for anything out of the ordinary.