Here's a a couple dozen tweets on how this happens, and what to do about it. #tweetstorm
That overreliance on data is especially bad with small n, or in complex domains, or in domains with non-predictive theory.
This is basically @nntaleb's case of induction by turkeys - they weren't killed yet, so they are safe. In fact, even with very large n, all data is conditional, not absolute.
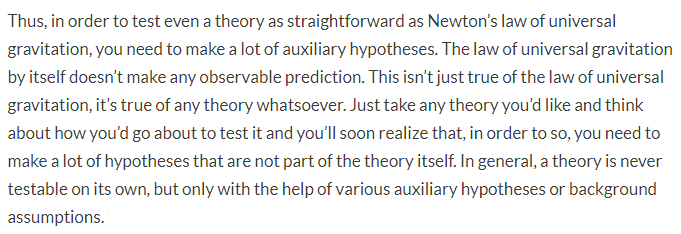
That means we should identify what the key uncertainties about our conclusions are. If possible, we should also find where further evidence would help, consider gathering it.
Theory predicts that X% of height is genetic, conditioned on current distribution of population genetic variation, and on sufficient nutrition as a child, and on age, and on not having osteoporosis.
Without paying attention to the conditions, data can and will be taken to imply things incorrectly. If the conditions have changed, and we don't notice, we will often make sweeping but completely invalid conclusions.
If you liked the tweetstorm, check out my other work!