Thread reviewing the Bank of International Settlement's paper on Fintech & BigTech lending
bis.org/publ/work779.p…
⬇️⬇️⬇️
(1/15)
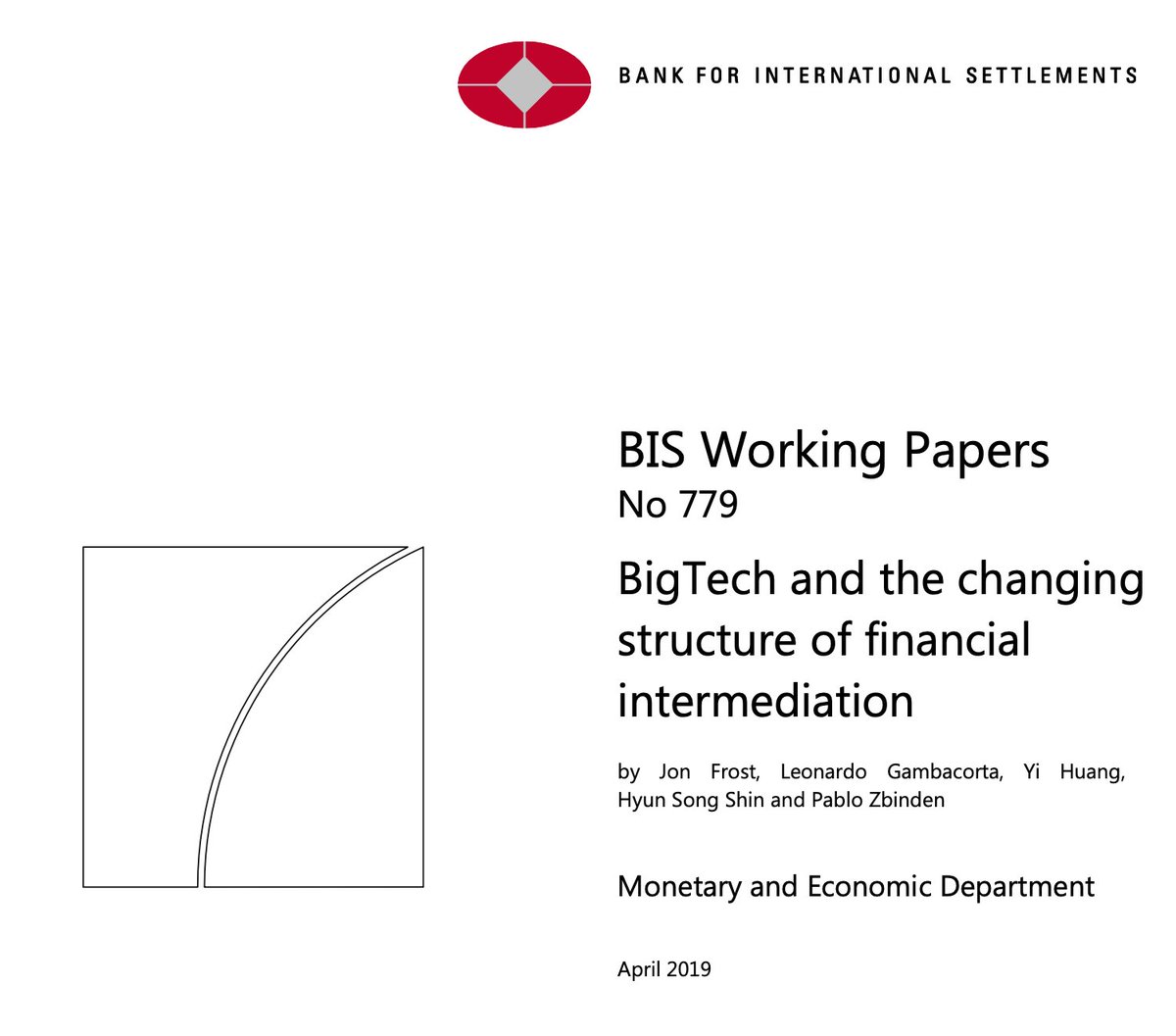
Check out this list of requirements by the US Small Business Administration to obtain a loan. Plan a few weeks to work that off
sba.gov/sites/default/…
(2/15
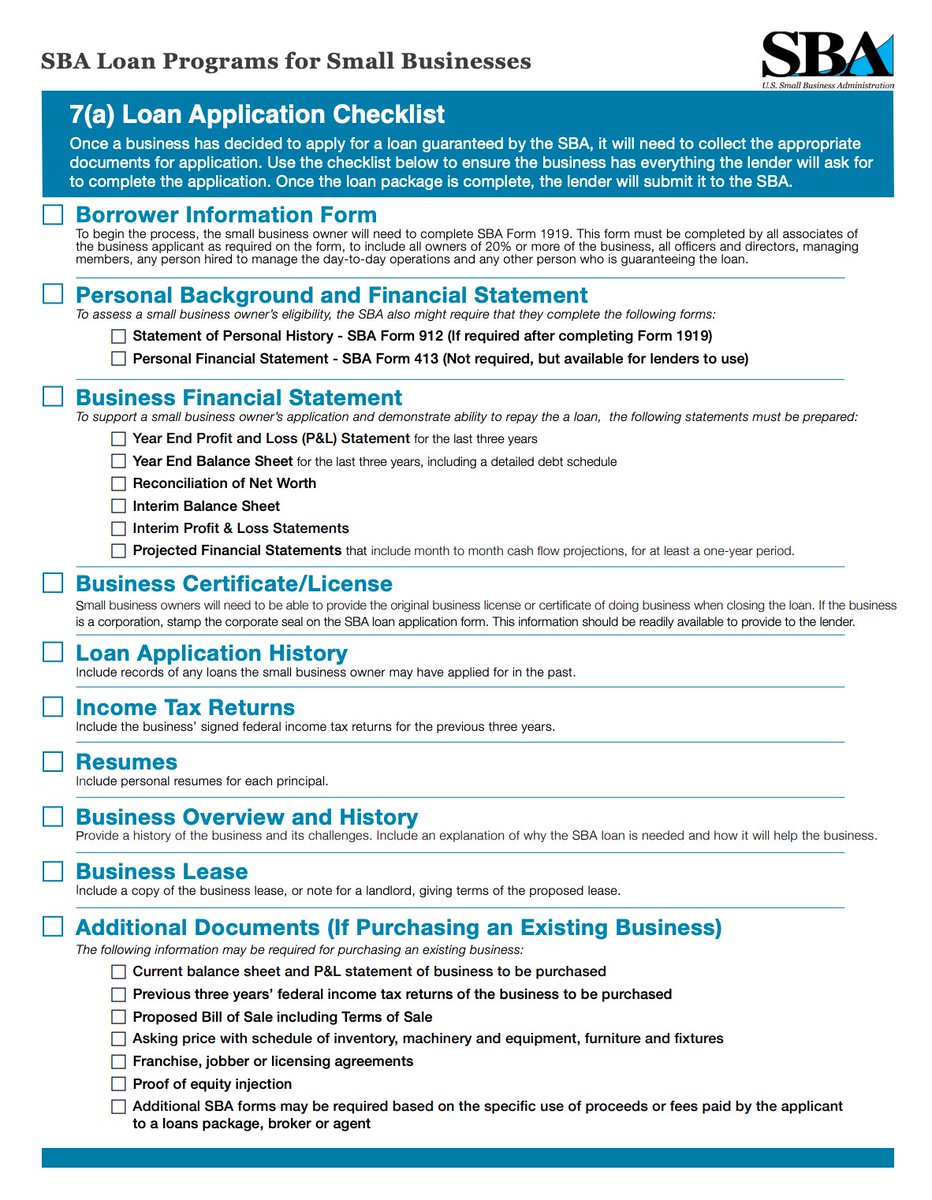
(3/15)
(4/15)
(5/15)
(7/15)
(8/15)
(9/15)
(10/15)
Check out this introduction if you're interested. Also being not a data scientist, it's an interesting concept.
(11/15)
(13/15)
(14/15)
Check out the full paper here:
bis.org/publ/work779.p…
(fin)