arxiv.org/pdf/1906.04652…
(do tell me if this was done before, or somehow predicted by Aristotle).
We ain't no gas molecules, so how is this relevant human behavior?
We (typically) cannot access averages that are computed over multiple agents or parallel universes.
Win 50% of your current wealth for heads, lose 40% of your current wealth for tails.
Changes in wealth now are non-ergodic, so calculating the expectation value is not informative of the time average growth rate of wealth.
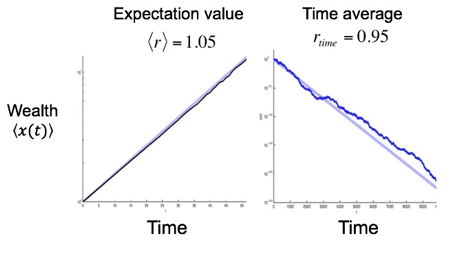
All ideas so far are from @ole_b_peters, Murray gell-mann + @alex_adamou.
...then the two classes of theory make different predictions for how people make choices.
Via a Bayesian hierarchical model of isoelastic utility we estimated risk aversion parameters.
If time optimal, they should shift from approximately linear utility to log utility, when ergodicity breaks in moving from additive to multiplicative dynamics.
Ergodicity-breaking can exert strong and systematic effects on human behavior.
Switching from add- to multi- dynamics reliably increased risk aversion, which in most subjects tracked close to the levels that maximise the time average growth of wealth.