"18 [#deeplearning] algorithms ... presented at top-level research conferences ... Only 7 of them could be reproduced w/ reasonable effort ... 6 of them can often be outperformed w/ comparably simple heuristic methods."
Paper:
lnkd.in/dTaGCTv
#AI
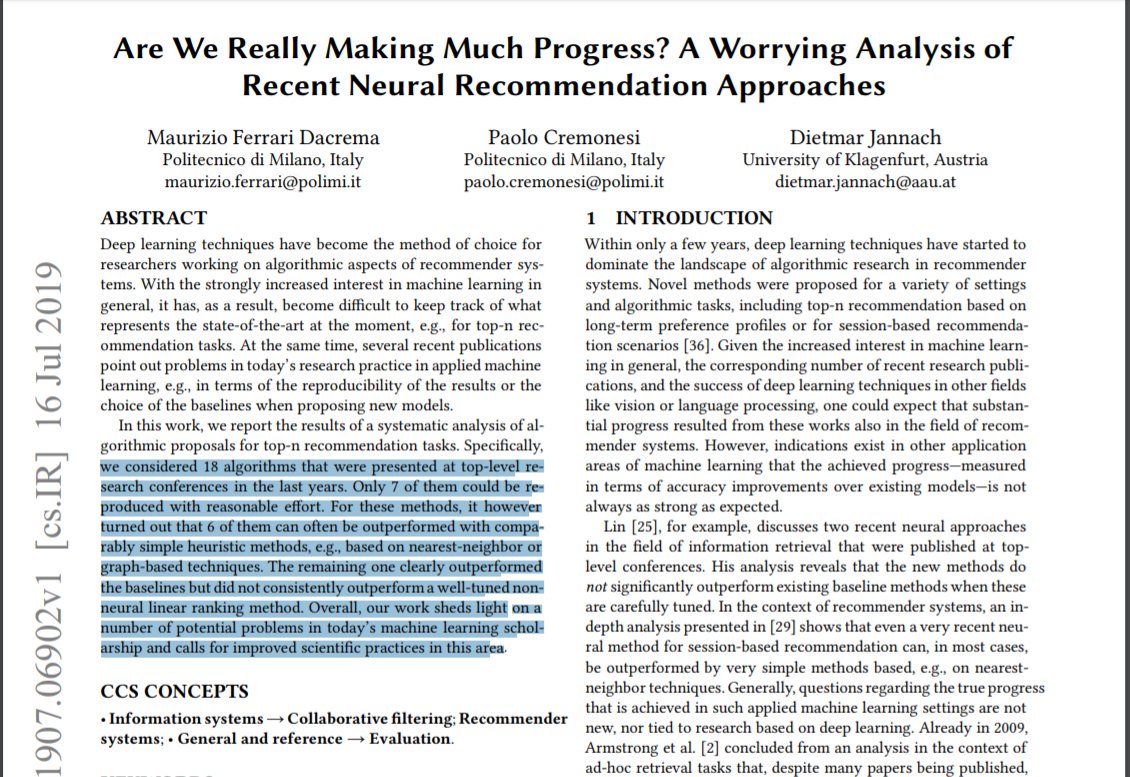
2/
There is much concern about #reproducibility issues and flawed scientific practices in the #ML community in particular & #academia in general.
Both the issues and the concerns are not new.
Isn't it time to put an end to them?
There are several works that have exposed these and similar problems along the years.
👏👏 again to @Maurizio_fd et al. for sharing their paper and addressing #DL algorithms for recommended systems (1st tweet from this thread).
But there is more, unfortunately:
Don't miss the chapter and the accompanying video of Craig Tovey's tutORial @ INFORMS2018:
"Nature-Inspired Heuristics: Overview and Critique"
Pure gold!
H/T @TedPavlic
Tovey: "Some of the more recently invented methods have exotic names such as roach infestation, shuffled frog-leaping, invasive weed optimization, and cuckoo search. This tutorial is a guide to the bewildering, burgeoning menagerie of such heuristics"
Some #DL authors: 🙈🙉🙊
Craig Tovey's tutORial addresses population-based metaheuristics.
Needless to say, #neuralnets (and thus #DL as a special case) are not only another kind of metaheuristics but also suffer from the same sicknesses.
Fun intermezzo: A guy here in Twitter explained to me what neural nets are!
🤦♀️
That made my day today 🤣🤣🤣🤣
This is a paper that puts one concrete example of #metaheuristic algorithms under fire:
"A critical analysis of the harmony search algorithm—How not to solve sudoku" by Dennis Weyland.
H/T @ChrKroer
😳
"[M]ore than a decade of research about harmony search has not revealed any other sort of novelty or has led to any new insights or significant contributions in the field of heuristics. In short, there is no reason for [it] to exist as a separate metaheuristic framework."
I don't get tired of pointing to @zacharylipton and Steinhardt's paper on "Troubling Trends in Machine Learning Scholarship":
arxiv.org/abs/1807.03341
It should be of mandatory reading/discussion for every #ML #AI practitioner, researcher, student, etc.!
@MFischetti (thanks!) adds another worth reading paper to the collection:
"Metaheuristics—the metaphor exposed" by Kenneth Sörensen
Sörensen on 'new' algorithms: "[I]t seems that no idea is too far-fetched to serve as inspiration to launch yet another #metaheuristic. In this paper, we will argue that this line of research is threatening to lead the area of metaheuristics away from scientific rigor."
@ChristophSchll3 et al. question the use of #neuralnetworks for pedestrian motion prediction in "The Simpler the Better: Constant Velocity for Pedestrian Motion Prediction."
In other words: why the urge to kill a mosquito with an artillery cannon?
arxiv.org/abs/1903.07933
#reproducibility of scientific research goes beyond #ML #AI.
Goodman et al. "review an array of explicit & implicit definitions of reproducibility & related terminology, & discuss how to avoid potential misunderstandings when these terms are used as a surrogate for 'truth.'"
(Goodman et al., 2016)
"What does research reproducibility mean?"
Science Translational Medicine 01 Jun 2016: Vol. 8, Issue 341, pp. 341ps12
#ML #AI - there is a lot to learn from other fields!
stm.sciencemag.org/content/8/341/…
*Spoiler alert!*
Short answer: "Not without checking."
eurekalert.org/pub_releases/2…
What do you need to consider in order to make your #ML #AI findings "reproducible"?
Olorisade et al. (2017) evaluate 30 studies and give some hints in "Reproducibility in Machine Learning-Based Studies: An Example of Text Mining"
semanticscholar.org/paper/Reproduc…