Long story short - this article was about how people were using deep learning to increase the pixel resolution of photos. I'd already explored DL for *segmentation*, but this was much more exciting!
arxiv.org/abs/1609.04802
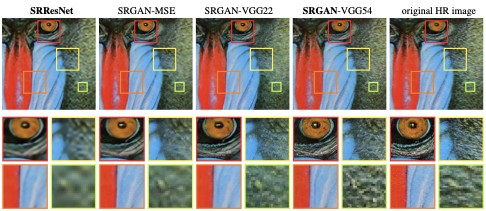
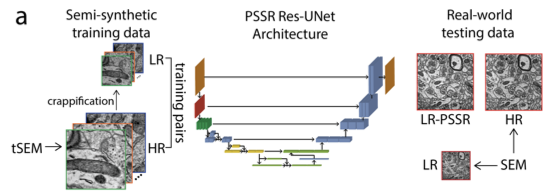
The crappifier simply adds noise while downsampling the training data. The tSEM training data was acquired by Kristin Harris's group, and we had a LOT.
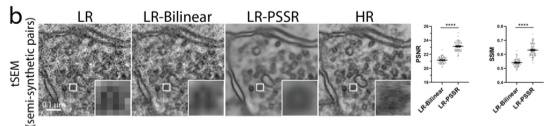
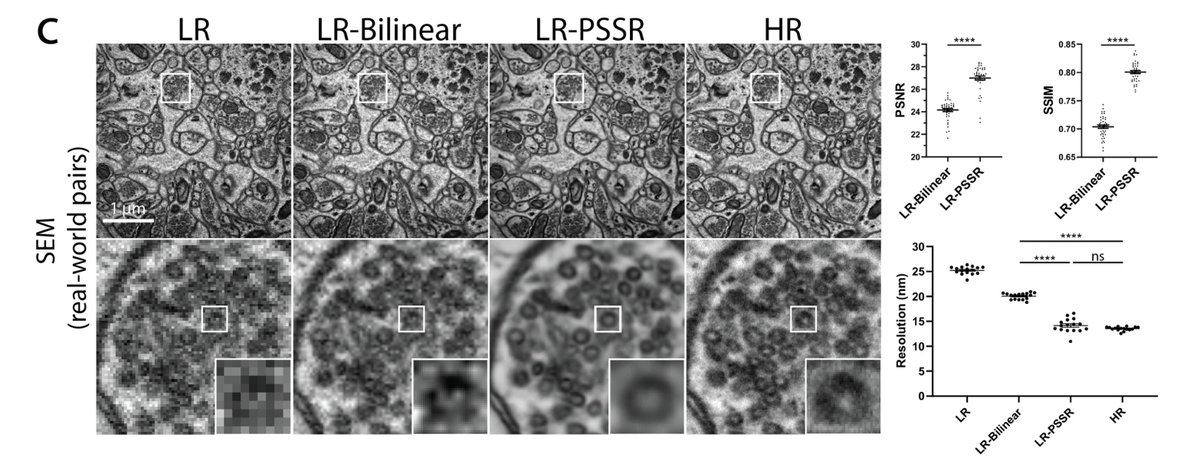
1) I'm not certain all our false positives are false. As mentioned above, our ground truth data is BSD SEM, which isn't as good as tSEM. So it is possible our model can better resolve data than our ground truth data
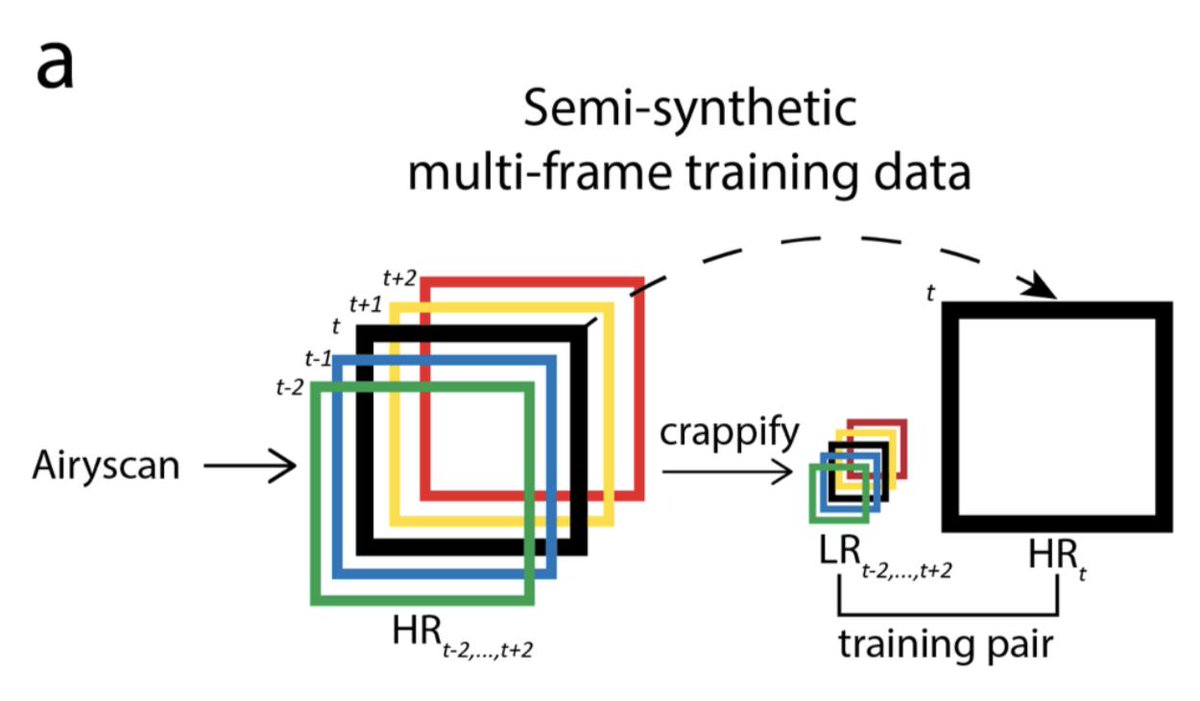
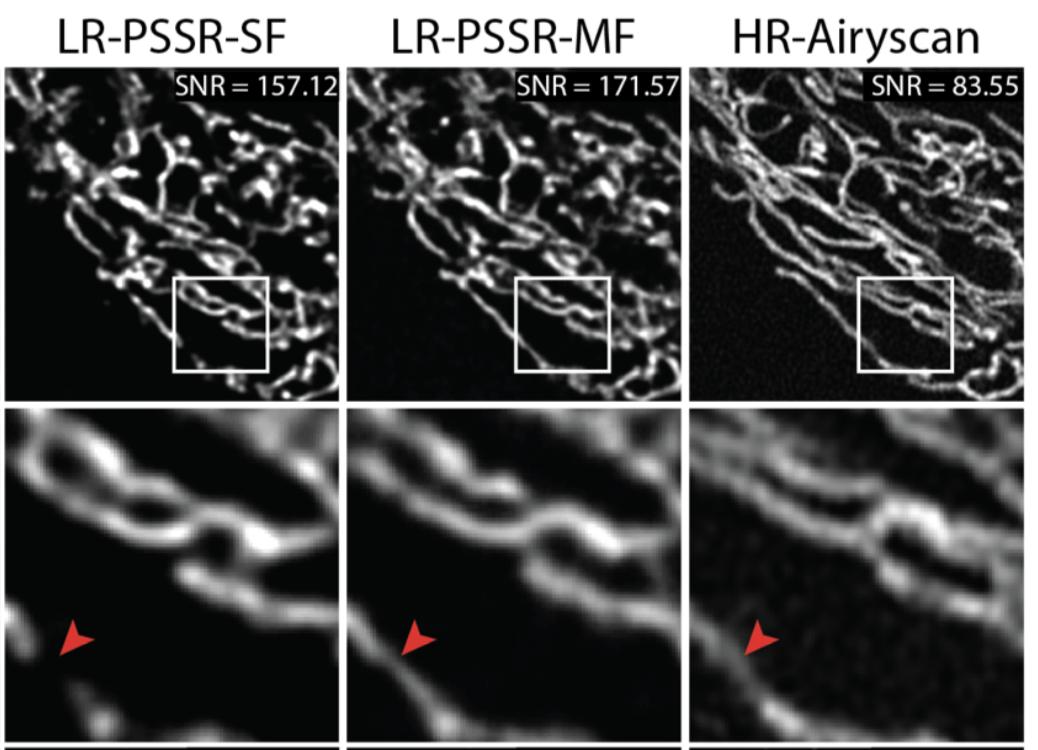
1) Laser in LR was reduced 5x (so total laser=90x lower)
2) LR is *confocal*. HR is @zeiss_micro AIRYSCAN.
See Airyscan (R) vs. confocal (L) compare with *identical* settings.
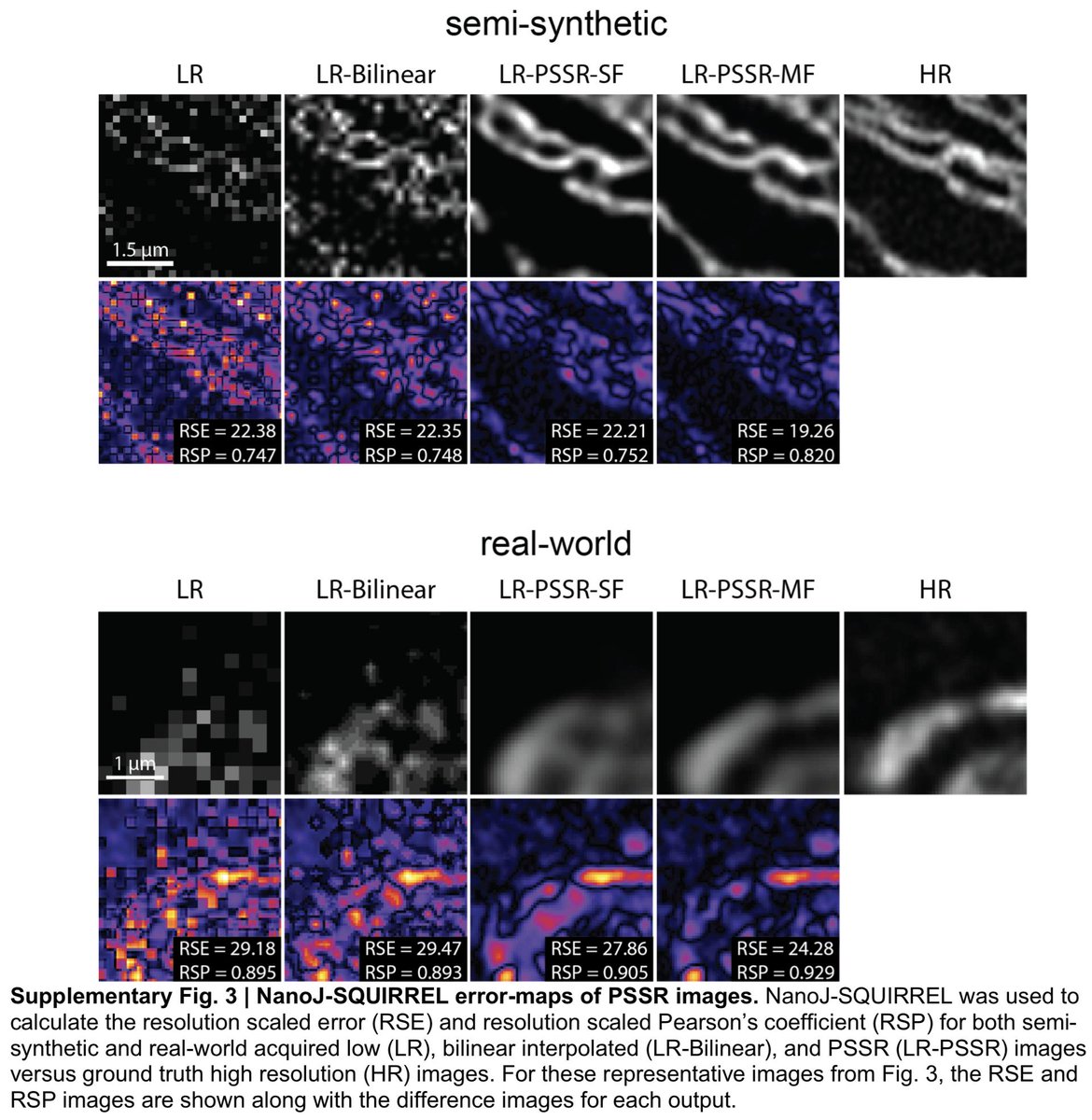
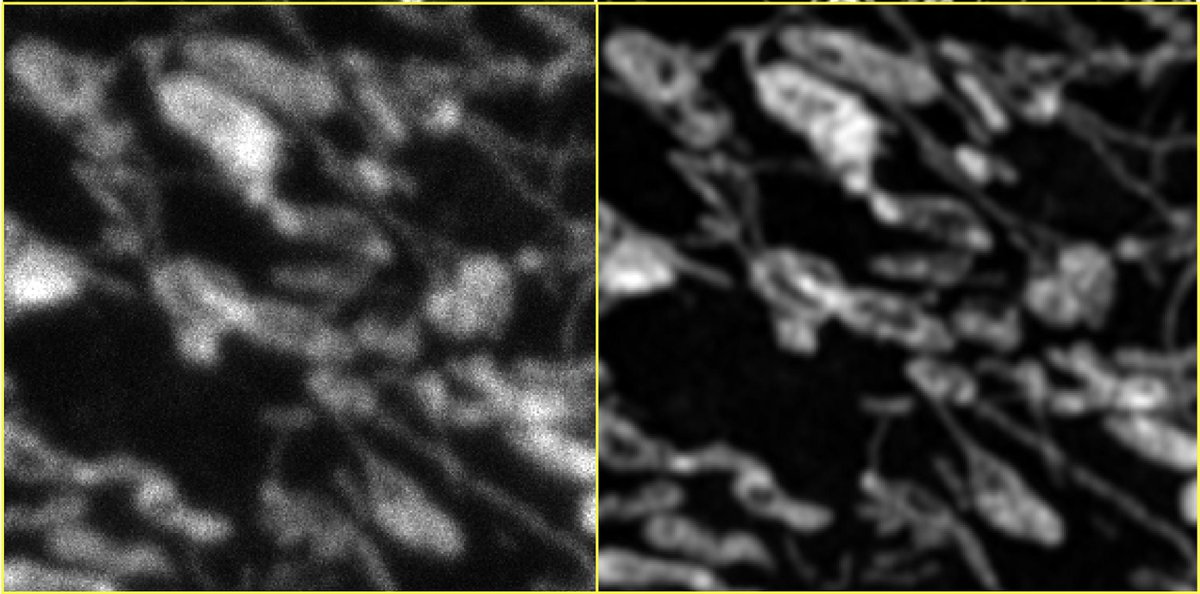
1) pixel super-resolution (aka "super-sampling")
2) Airyscan-quality deconvolution (or something close to that)
3) denoising
That's pretty remarkable considering only ~10GB of training data! FYI our EM dataset was ~80GB.
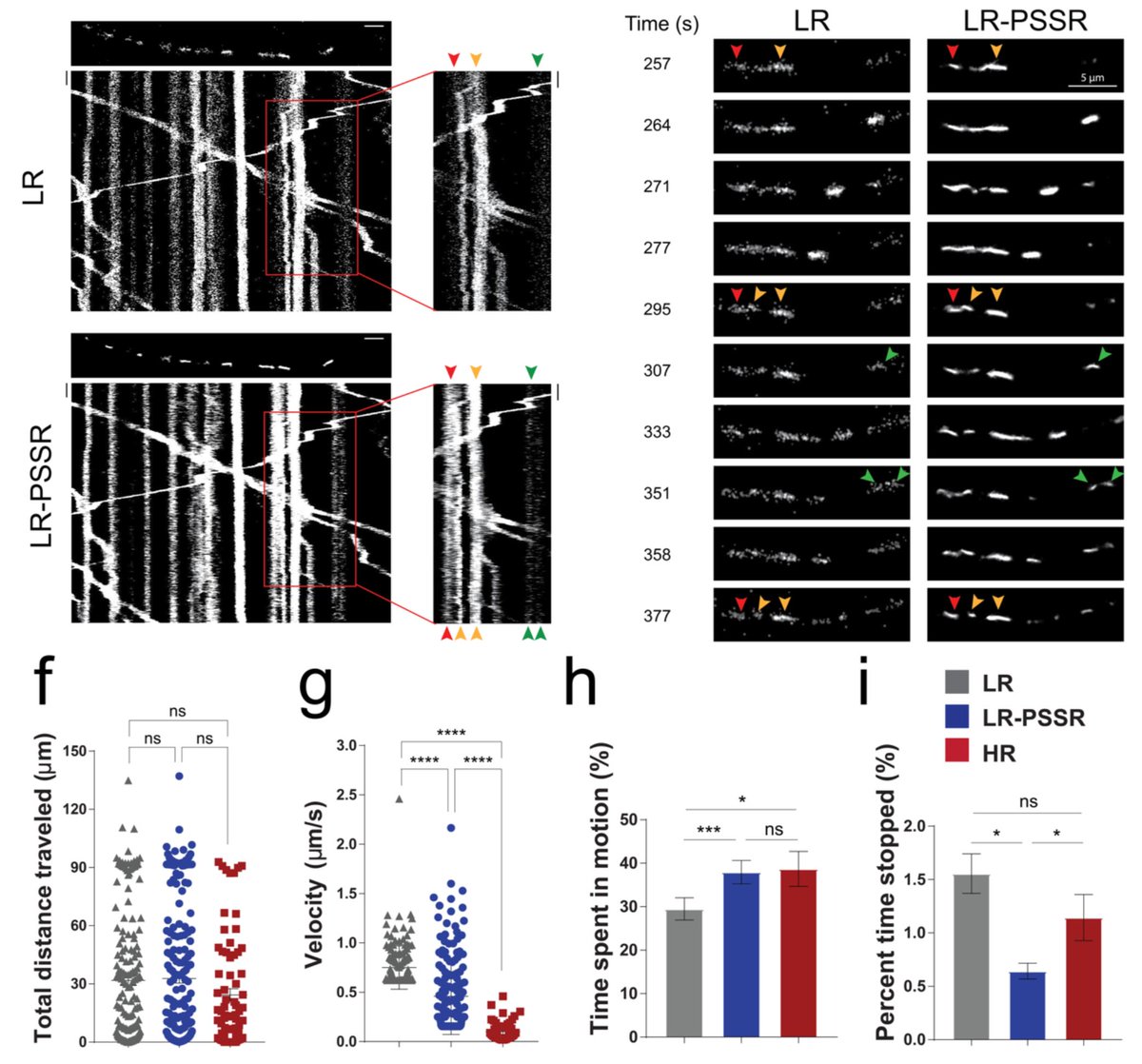
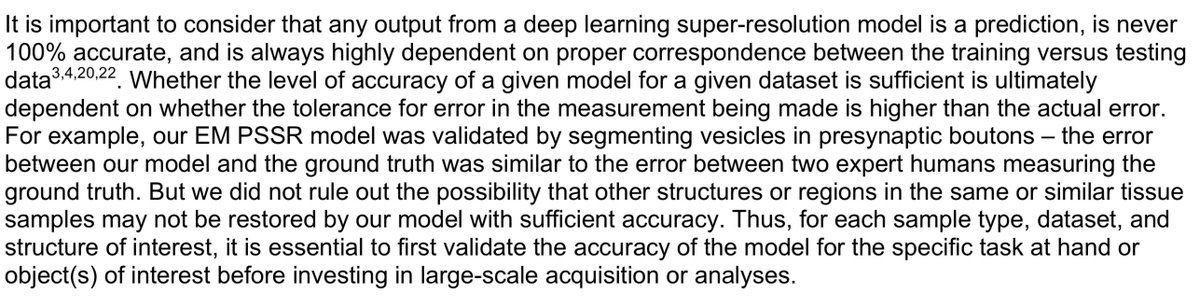
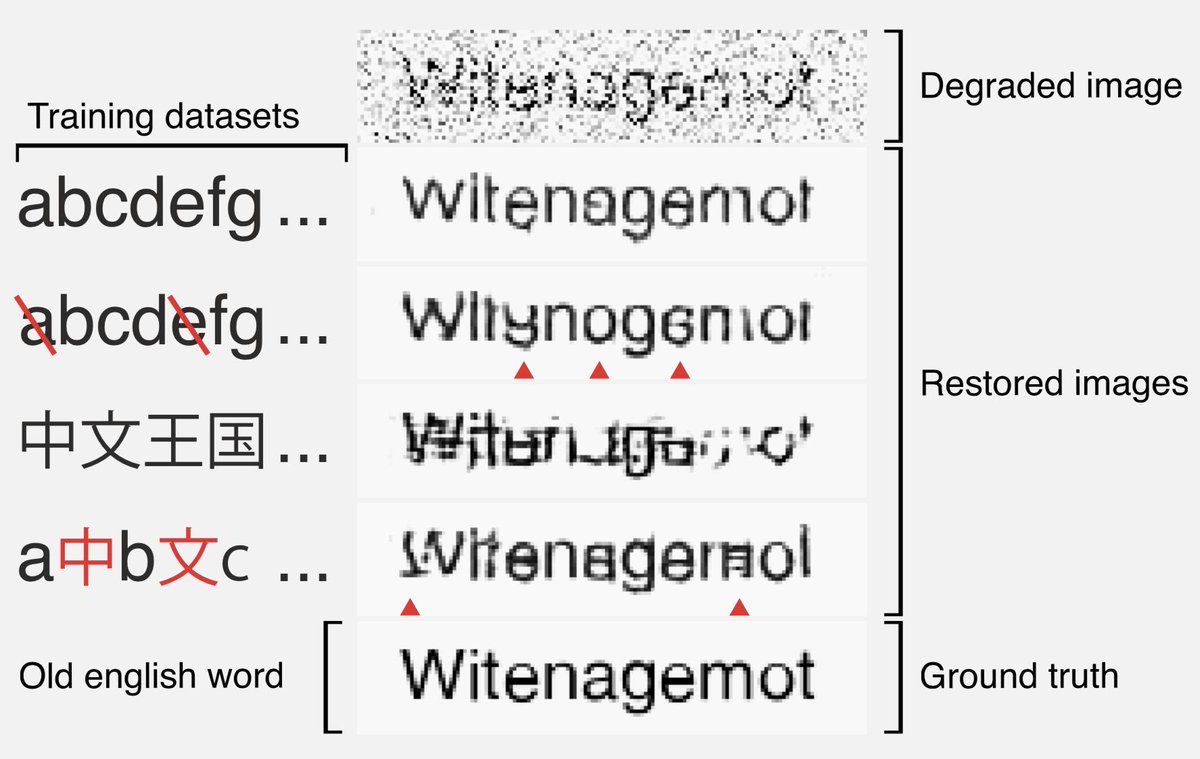
If the pixel size is wrong, it won't work so well. Same thing happens with PSSR, which for EM was specifically trained for 8nm LR data.
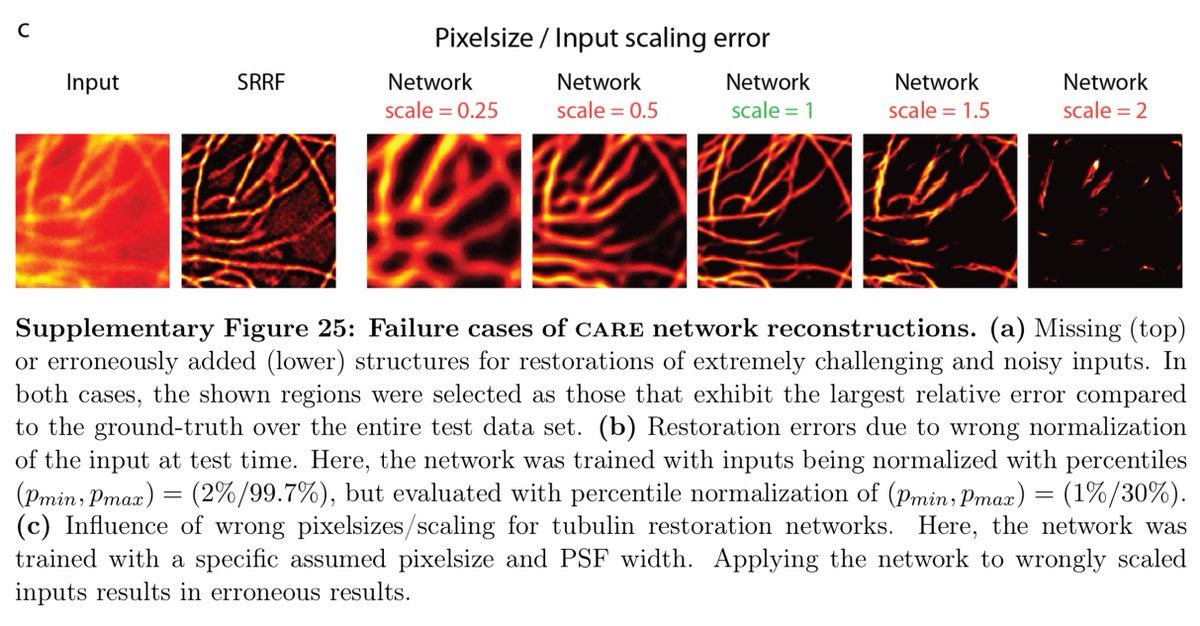
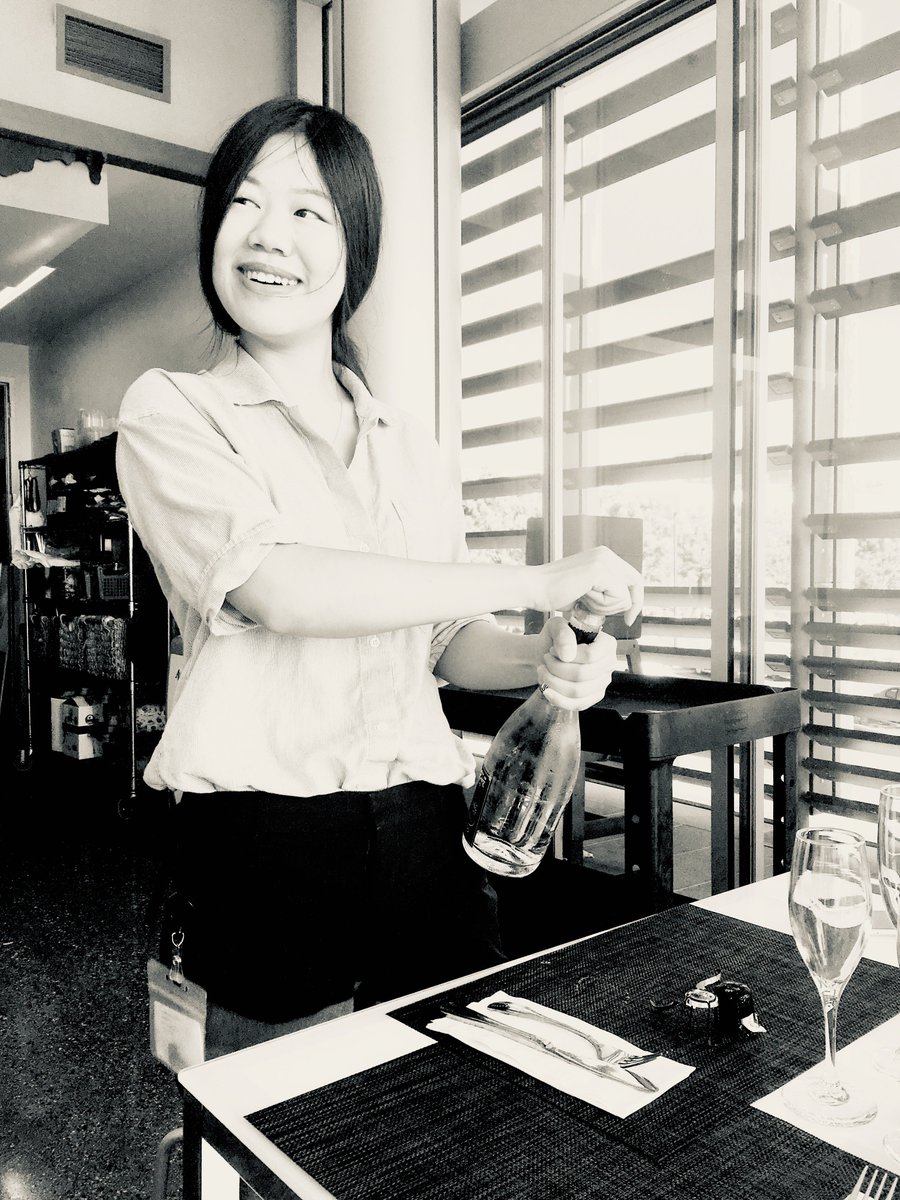
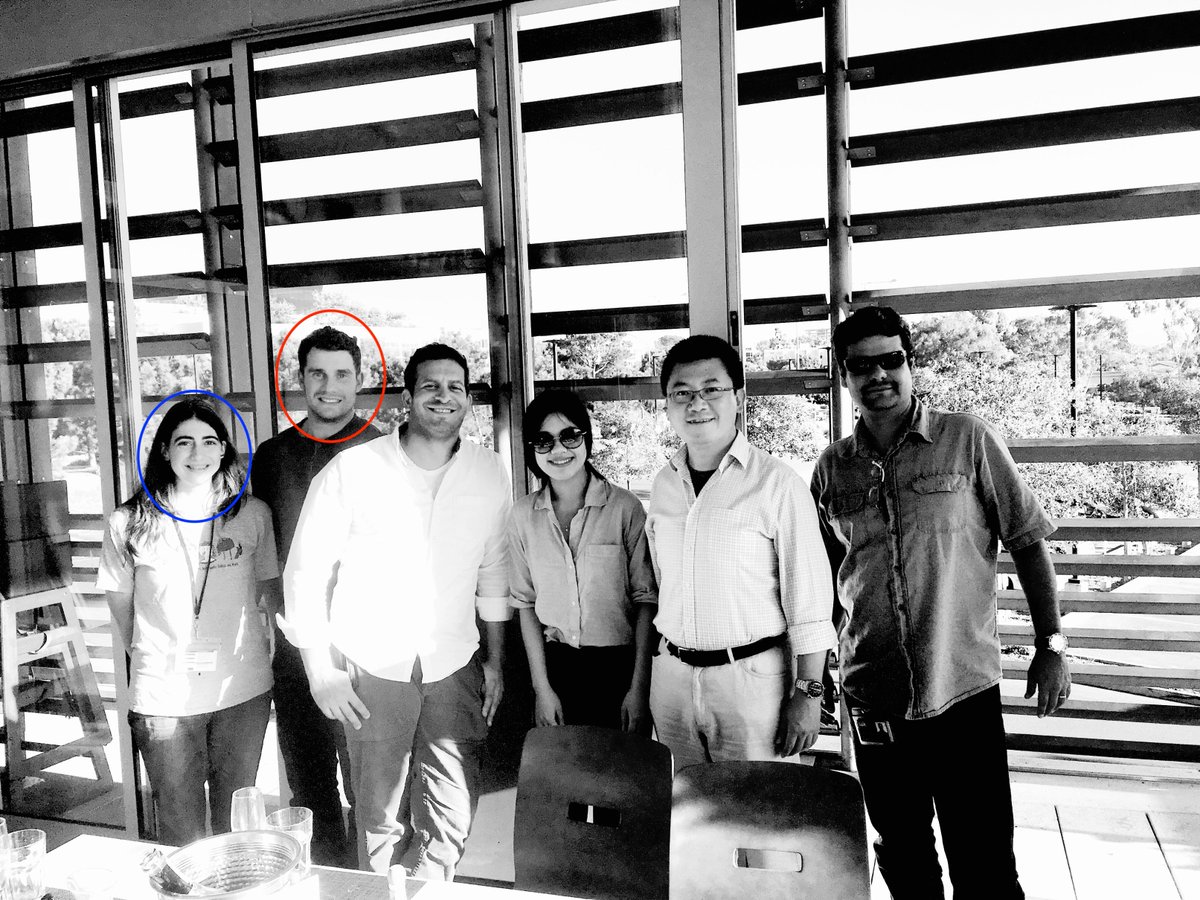
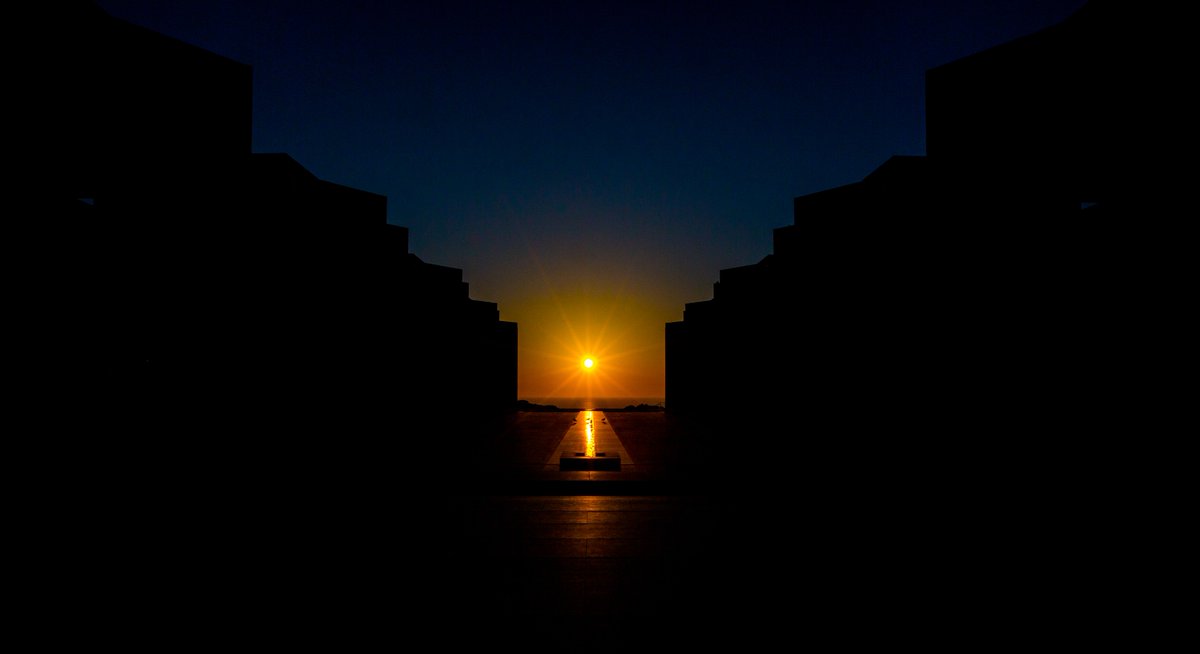
news.utexas.edu/2019/09/03/tex…
1) ALL THE DATA is now downloadable at doi.org/10.18738/T8/YL… thanks to the amazing team at @TACC
2) We updated our preprint! Specifically, we added references to @docmilanfar's video superres work and fission event data in Fig 3.
doi.org/10.1101/740548
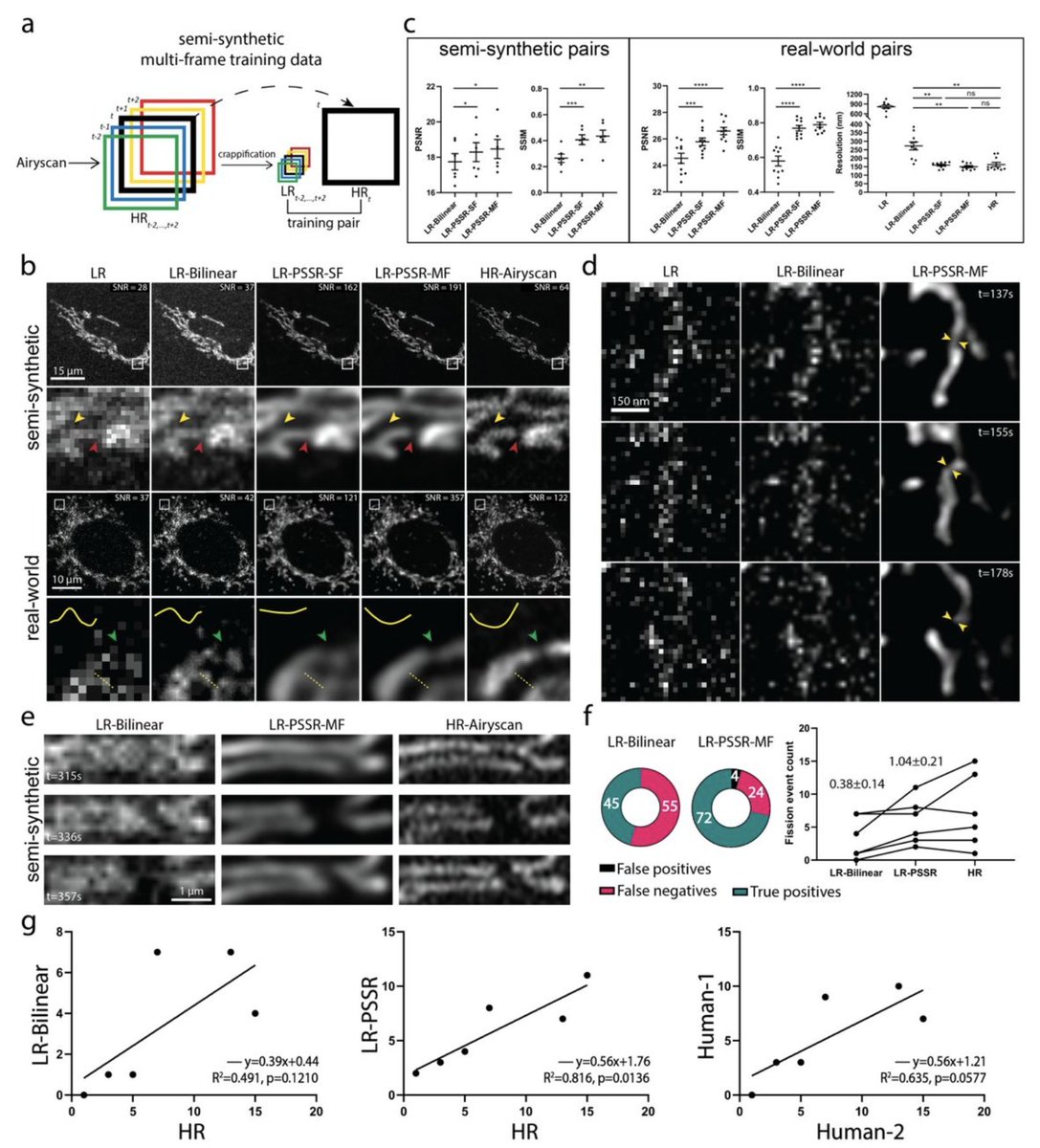