The research paper by @leeb & @wattenberg that introduced streamgraphs to the world is one of the best dataviz papers I've ever read
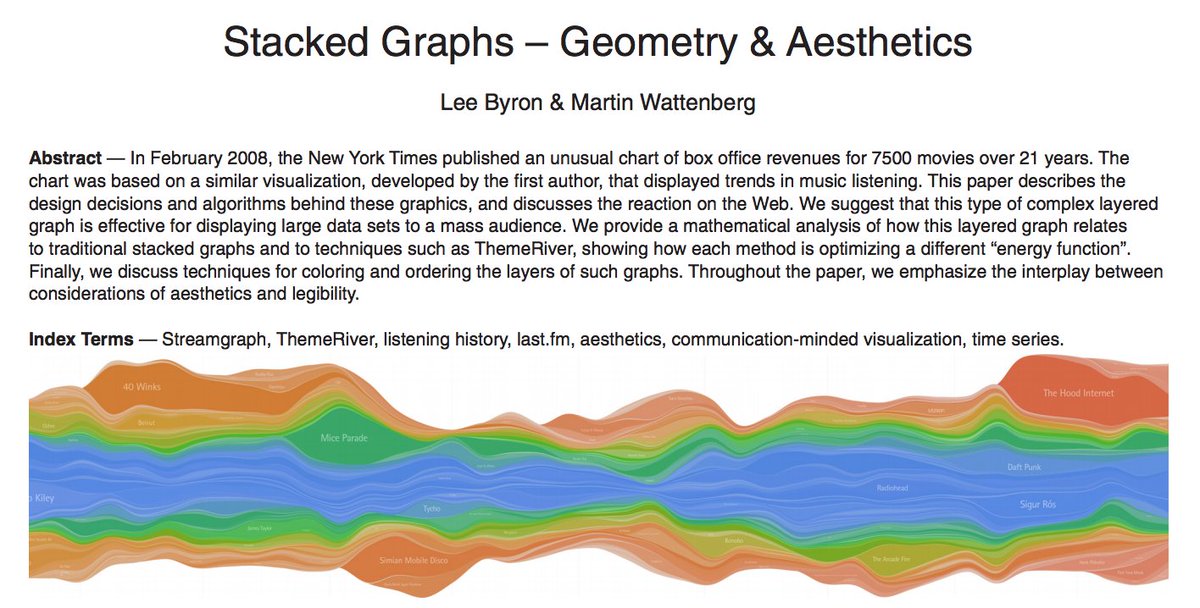
Intro: the paper followed 2 early examples of stacked, meandering graphics
- "Listening Histories": last fm listening data (pic 1)
- "The ebb and flow of movies" by @nytgraphics on box office takings (pic 2)

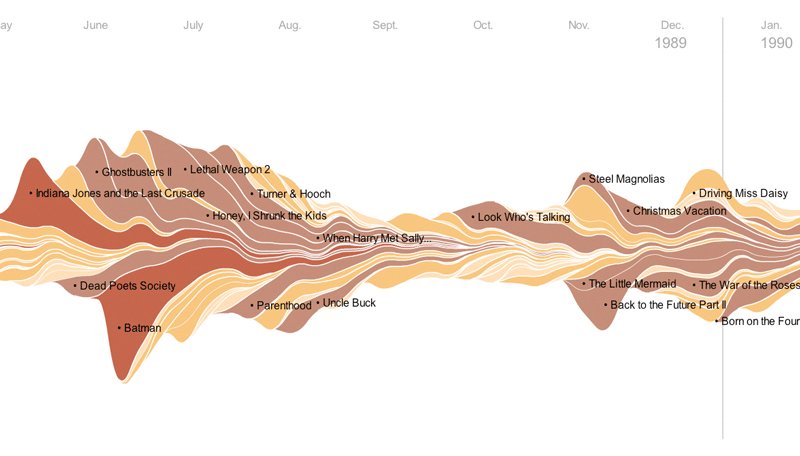
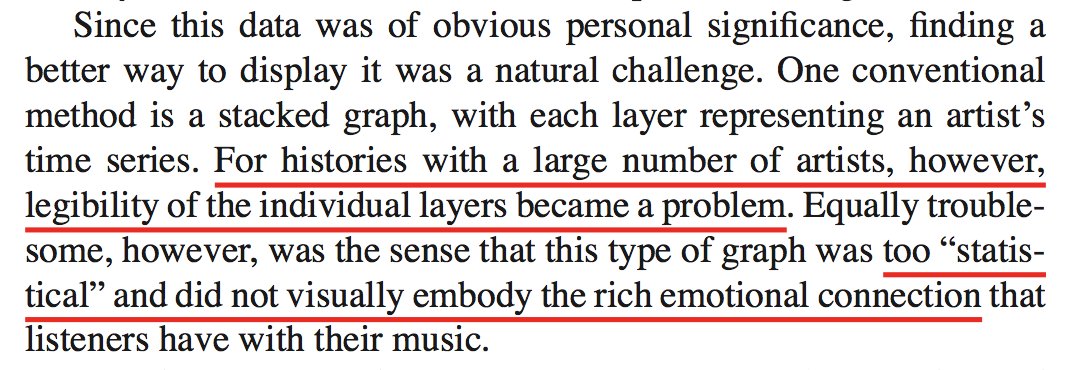
• Overall shape of stacked graph (silhouette)
• Ordering of layers
• Placement & styling of layer labels
• Colours
• Encoding some information from the data (maybe a category to which a layer belongs, maybe its overall size across the whole time series)
• Creating a boundary between layers [without requiring a stroke]
• Placement: at this point I'd actually point to more recent work by @veltman bl.ocks.org/veltman/3195a6…
• Colour: by using white labels on layers whose saturation grows with salience, you automatically make labels jump out more on important layers
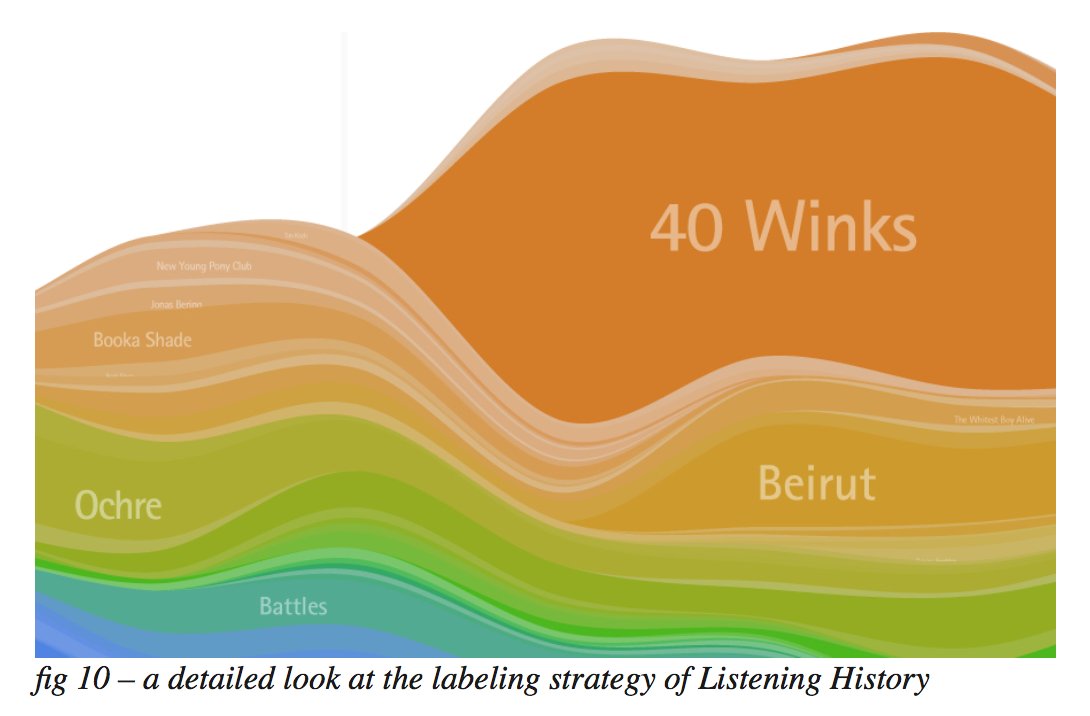
• If ur data is different — e.g u dnt have churn of new layers present in the movies dataset — u might want to order ur layers inside out by volatility: put least volatile layers in the middle, so outer baselines are distorted as little as possible
For me, #dataviz = problem solving & compromise in aesthetics & maths/geometry, in order to effectively communicate a message/story.
This paper embraces all of that, giving each part equal care & thought.