Proud to be published by @oiioxford, with thanks to the illustrious @pnhoward @lmneudert and @polbots.
comprop.oii.ox.ac.uk/research/worki…
The sort of thing often seen in bot strikes and attempts to game the Trending algorithm.
Flows which diverge massively from that range can then be studied in detail to see why.
(h/t @marcowenjones for the lead on this)
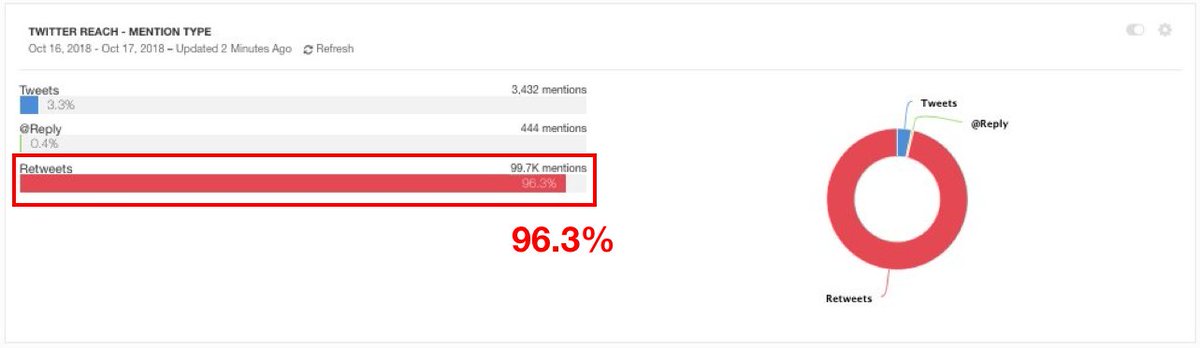
comprop.oii.ox.ac.uk/wp-content/upl…