- there's a test accuracy of 99%
- 8 million items
- resulting in 8 (ie: 8m at 1/1m) false-negatives
...solve this equation for the "badness" rate
Fortunately we can plug this into the code and run it until we see ~ 8 "inaccurate:bad" results
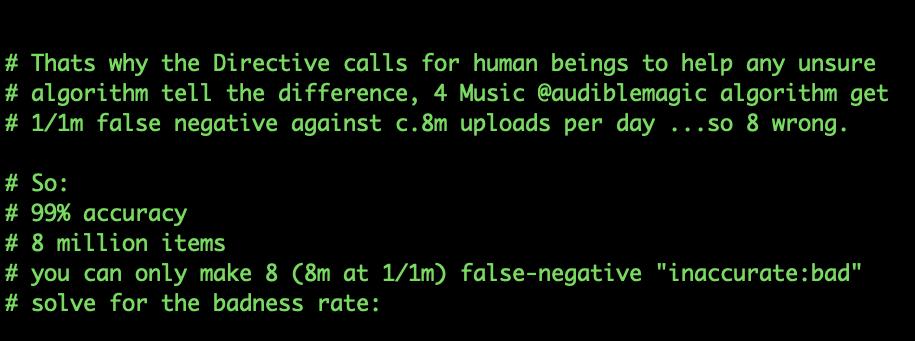
They are trying to get #Article13 imposed on the internet for 0.01% of uploads.
Those 8 false-negatives (ie: infringers you missed) are balanced by about 80,000 false-positives (ie: innocent people you squelched)
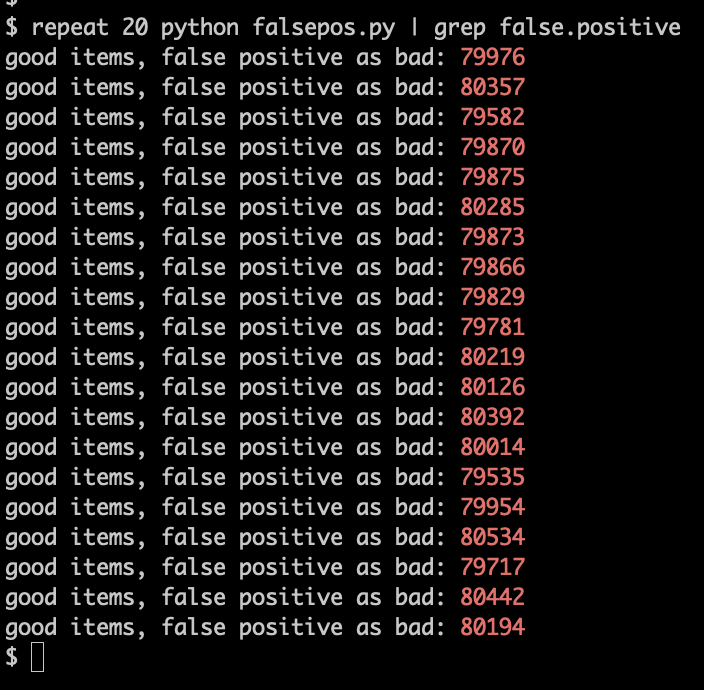
- 7.9 million correct-and-proper non-infringing posts
- 80,000 erroneous takedowns of non-infringing content
- about 800 correct-and-proper takedowns
- 8 infringements missed
This is the true cost & impact of #Article13
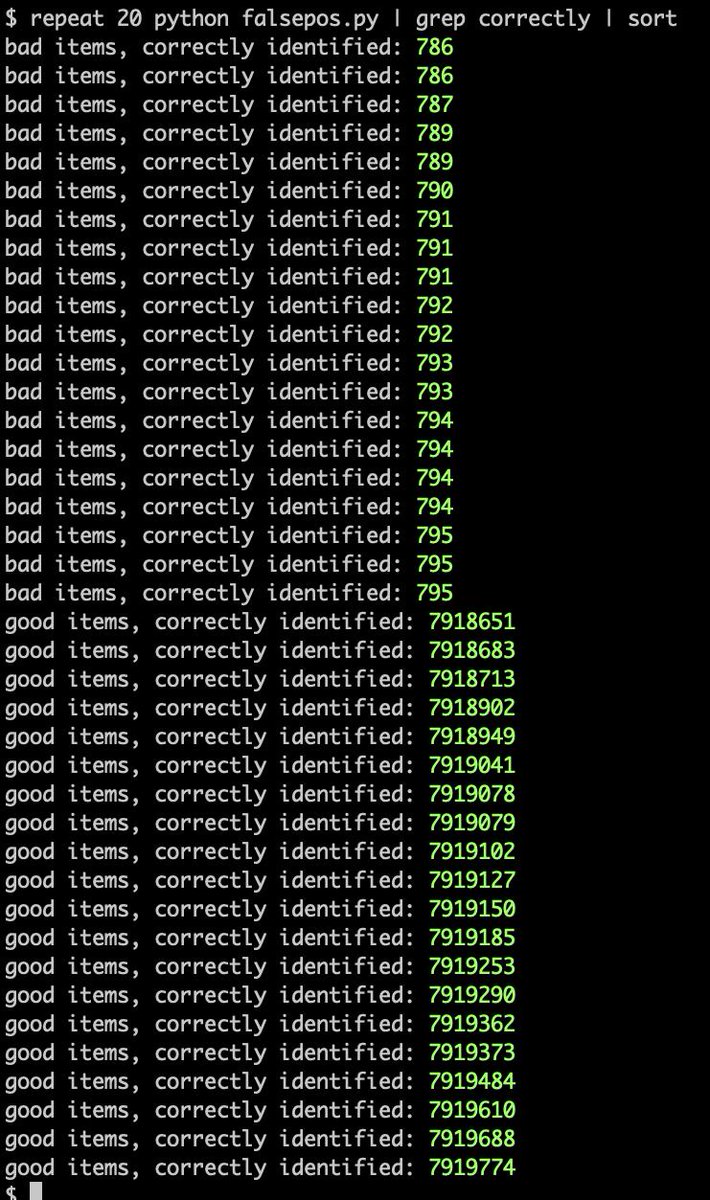
Fortunately he gave it the correct name.
@crispinhunt wasn't entirely clear on the difference between the two figures, either.
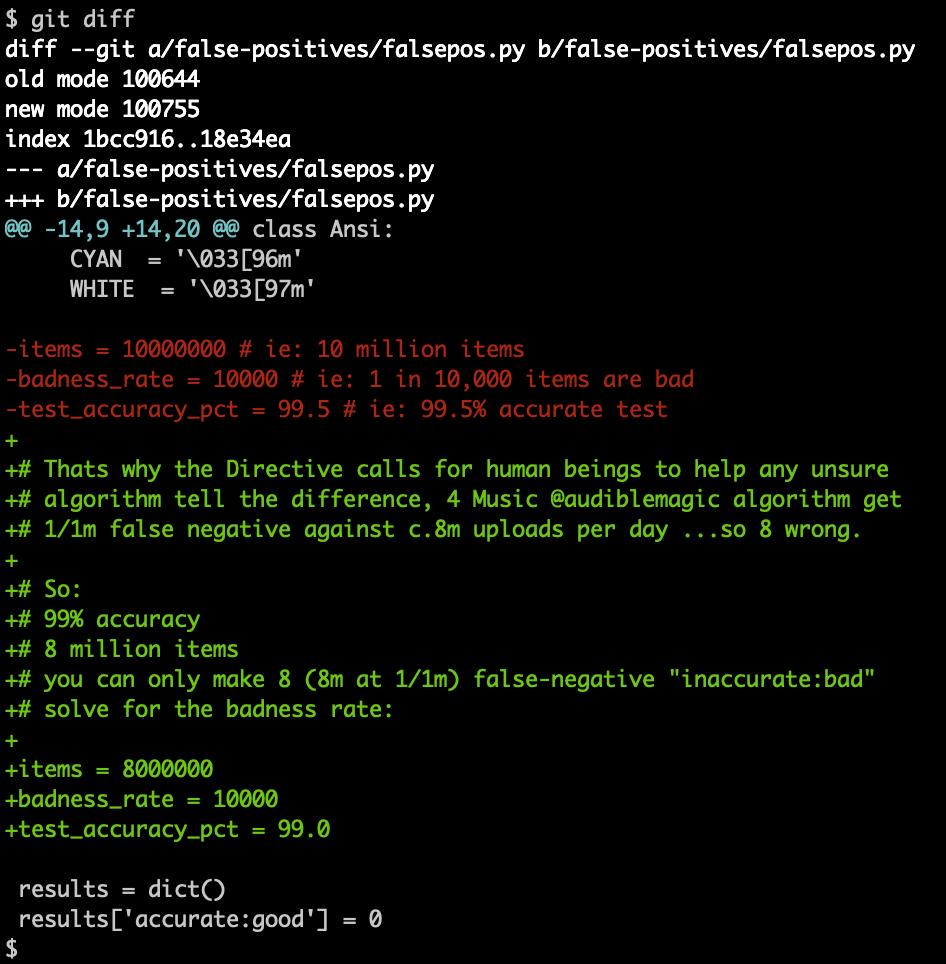
'What if @crispinhunt was not mistaken, and was actually saying there were 8 false _positives_ on 8 million uploads?'
Well … with a 99% accuracy rate, that's not really feasible; the FP rate is a function of the test accuracy (cont…)
This is why I think @crispinhunt cited a correct number with its correct name, erroneously.
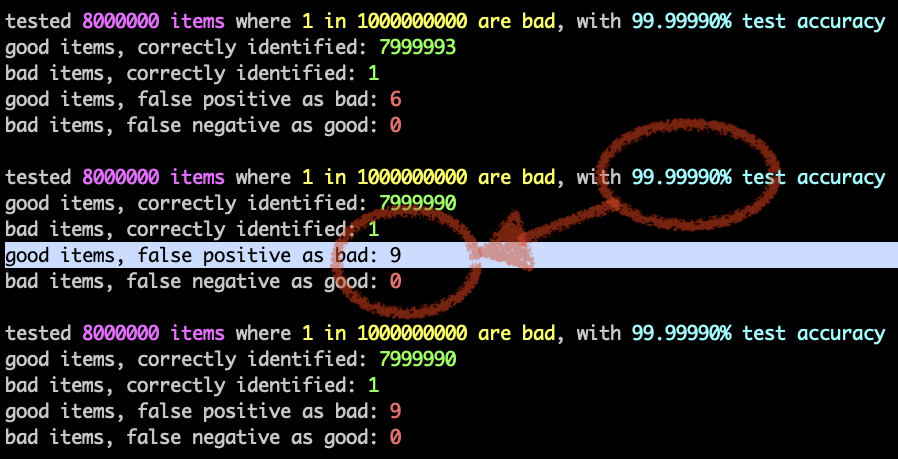
- 80,000 appeals per day
- 4,800 appeal reviews per person per day
- 80000 / 4800 = 16.67 = 17 review staff on 8h shifts
Assume wage = $10/h
17 staff * 8 hours * $10 * 365 days/year == $496,400
So: about $500k in wages for content reviewers?
Say it takes 60 seconds / 1 minute per review; then your appeal-review wage cost is $5 million per annum.
This is literally the #Article13-lobby's proposed *mitigation* to machine-learning's failures.
In terms of spin, it's quite clever.
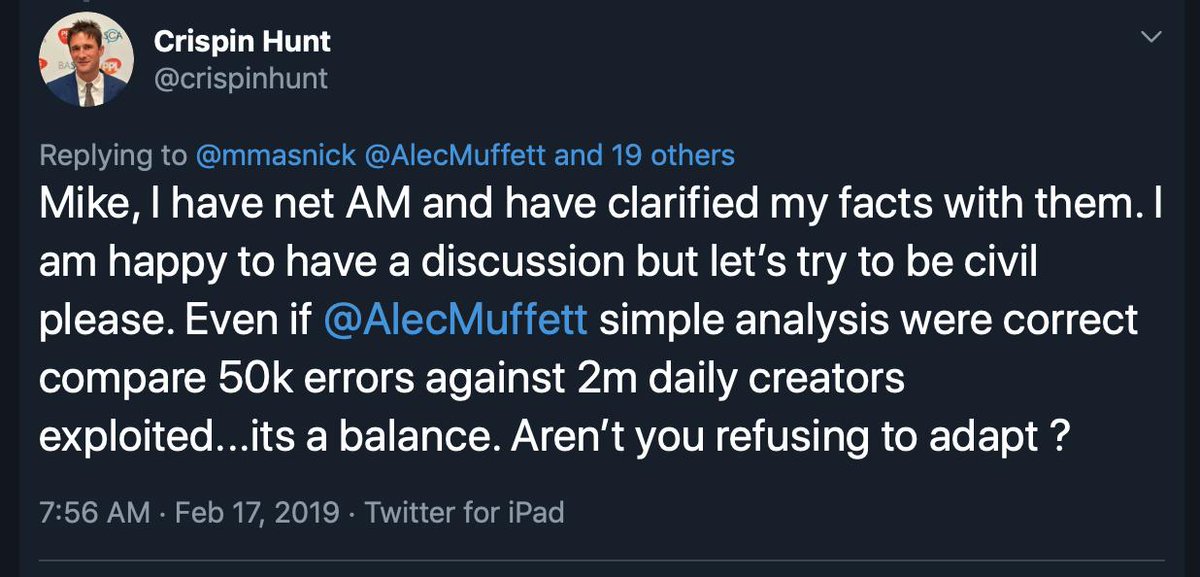
Secondly: the math suggests 80k errors PER DAY, and so we get annually: 80,000 * 365 = 29,200,000 erroneous takedowns.
- at what point
- in pursuit of prohibiting 0.01% of uploads
- in defence of protecting the copyright of less than 2 million artists
- does pissing-off up to* 29 million people per annum
…become acceptable?
* because statistical repeat-victims
1. degraded / sucky user experience
2. chilling effects on small business
3. reinforcement of the monopolies of big business which can afford this (because 2.)
4. opening the door to government censorship
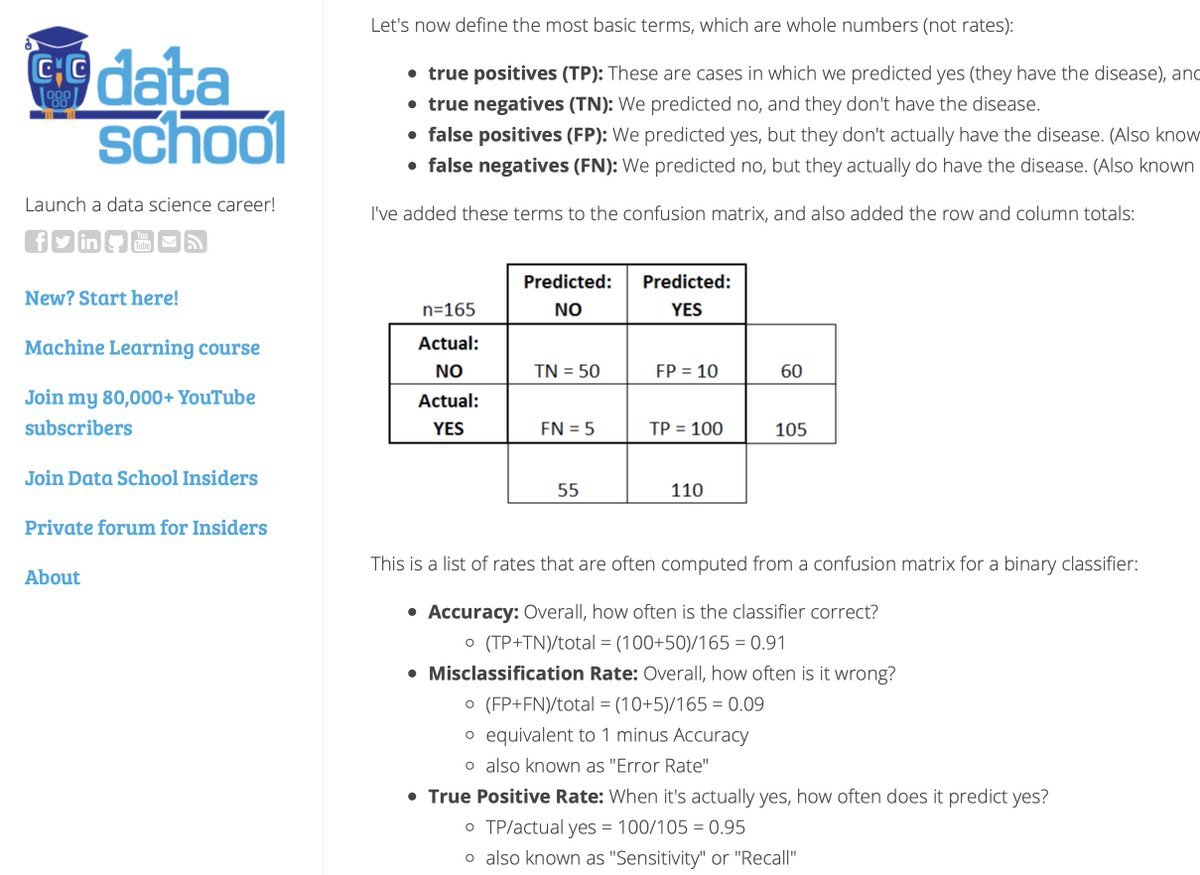
audiblemagic.com/why-audible-ma…
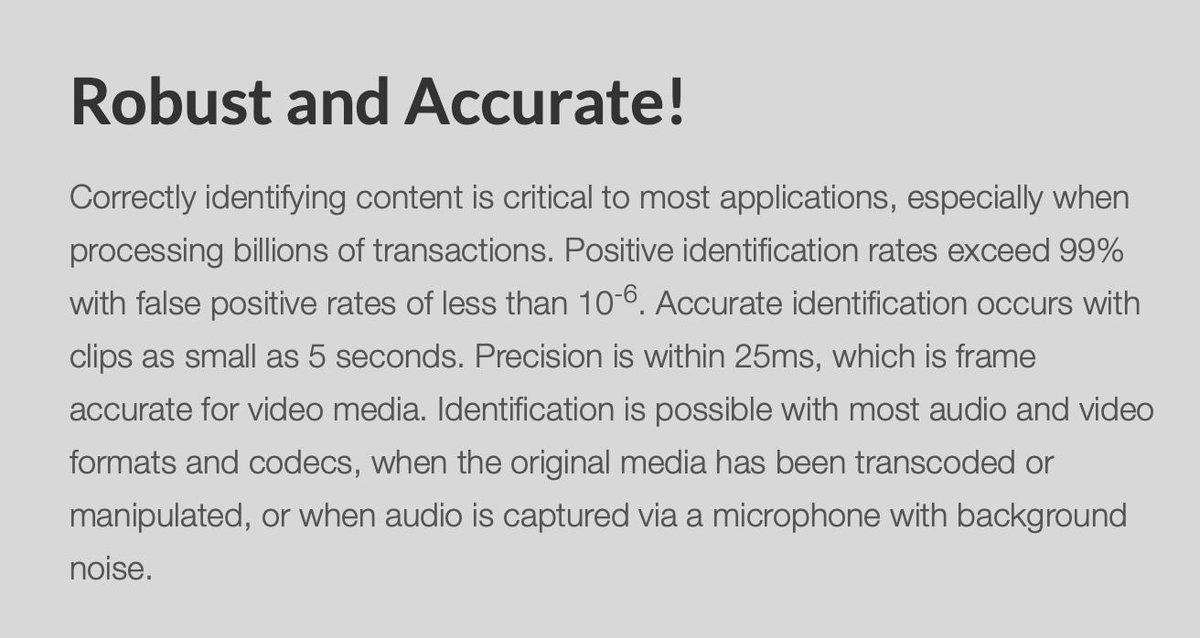
docs.google.com/spreadsheets/d…
en.wikipedia.org/wiki/Precision…
and dataschool.io/simple-guide-t…
But is it plausible, realistic, good?
In the meantime, what's going on behind the scenes?