Rather than hardcoding forward prediction, we try to get agents to *learn* that they need to predict the future.
Check out our #NeurIPS2019 paper!
learningtopredict.github.io
arxiv.org/abs/1910.13038
Rather than assume forward models are needed, in this work, we investigate to what extent world models trained with policy gradients behave like forward predictive models, by restricting the agent’s ability to observe its environment.
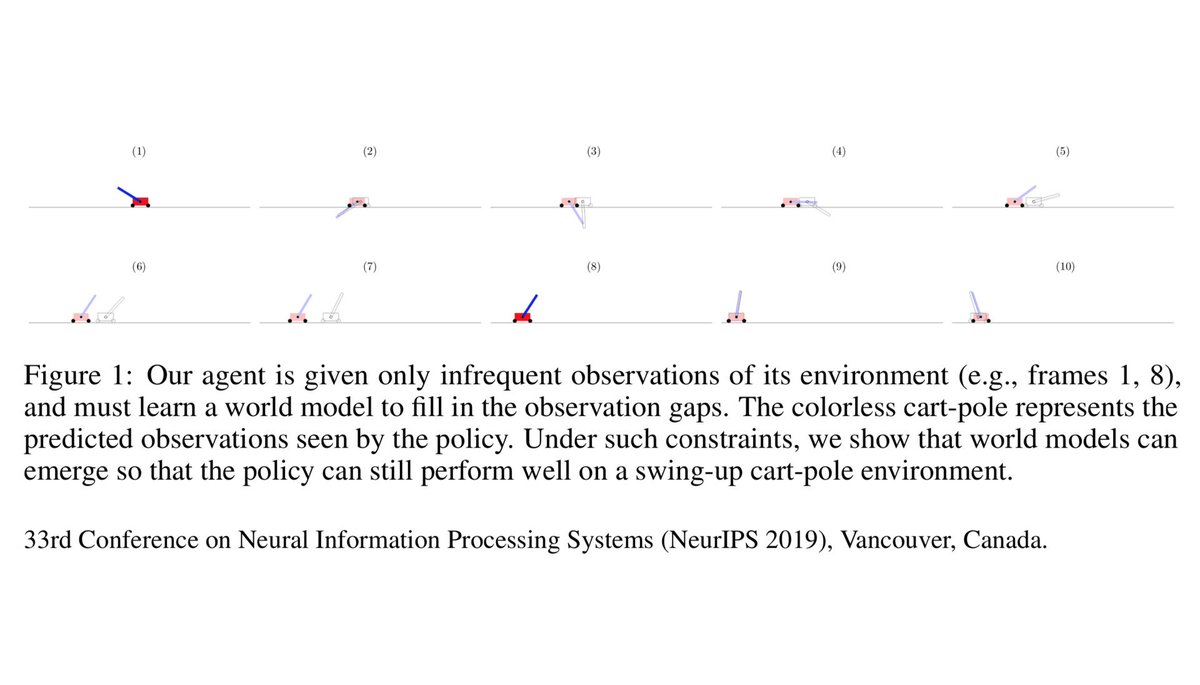
It's like training a blindfolded agent to drive. Its world model realigns with reality when we let it observe (red frames):
The true dynamics in color, full saturation denoting frames it can see. The outline shows the state of the emergent model.
Meanwhile, the model outputs a “predicted” observation based on the policy's previous observation and action.
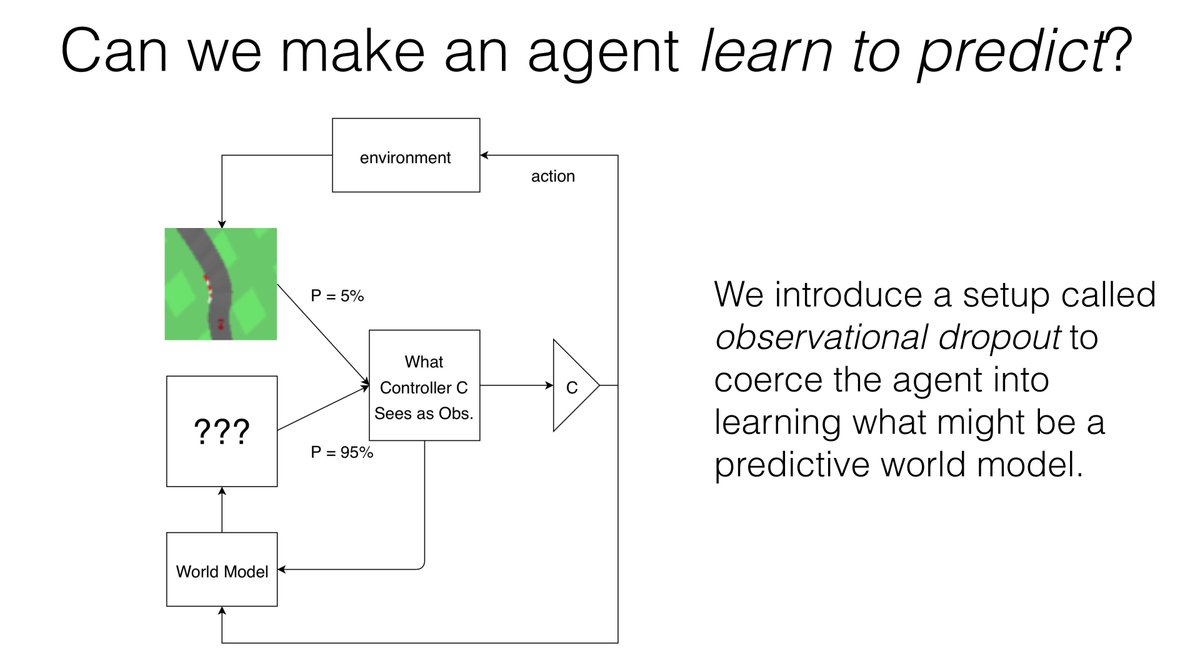
The “physics” the model learns is also imperfect. We can see this by training from scratch a policy on this model's “hallucination”
Our paper will be presented at #NeurIPS2019:
article learningtopredict.github.io
arxiv arxiv.org/abs/1910.13038
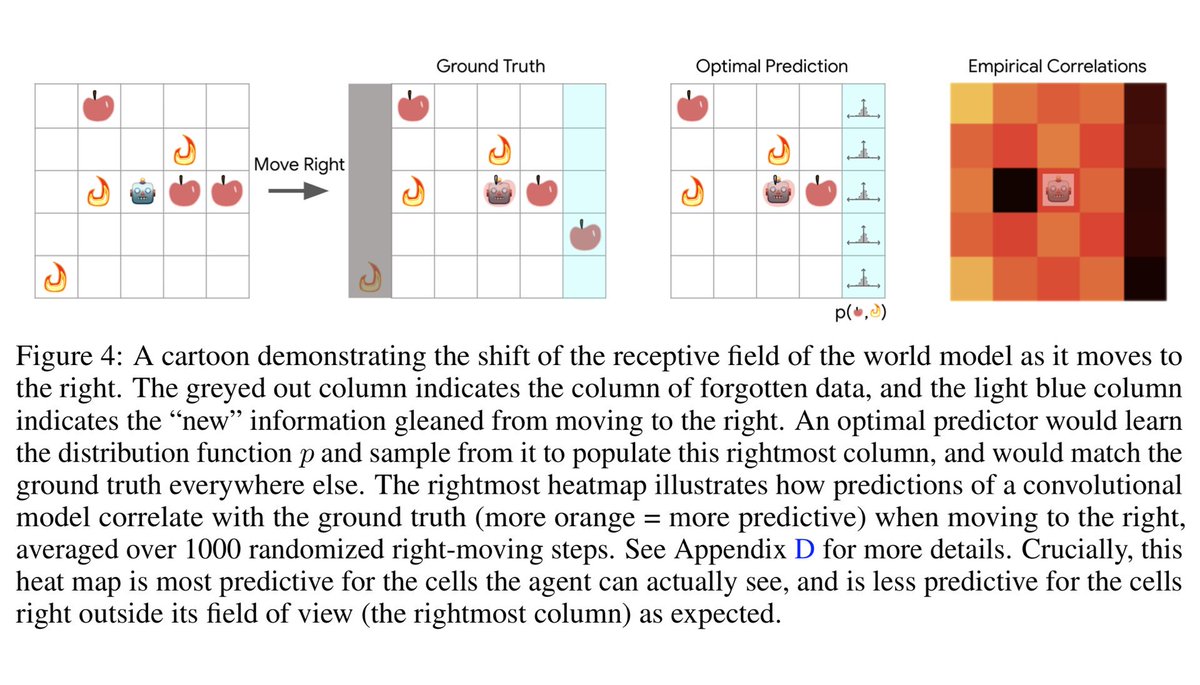
github.com/google/brain-t…