⏳Reducing your batch size increases training time; but it also decreases the likelihood that your optimizer will settle into a local minimum instead of finding the global minimum (or something closer to it).
If you're using @TensorFlow, standardization can be accomplished with something like tf.nn.moments and tf.nn.batch_normalization.
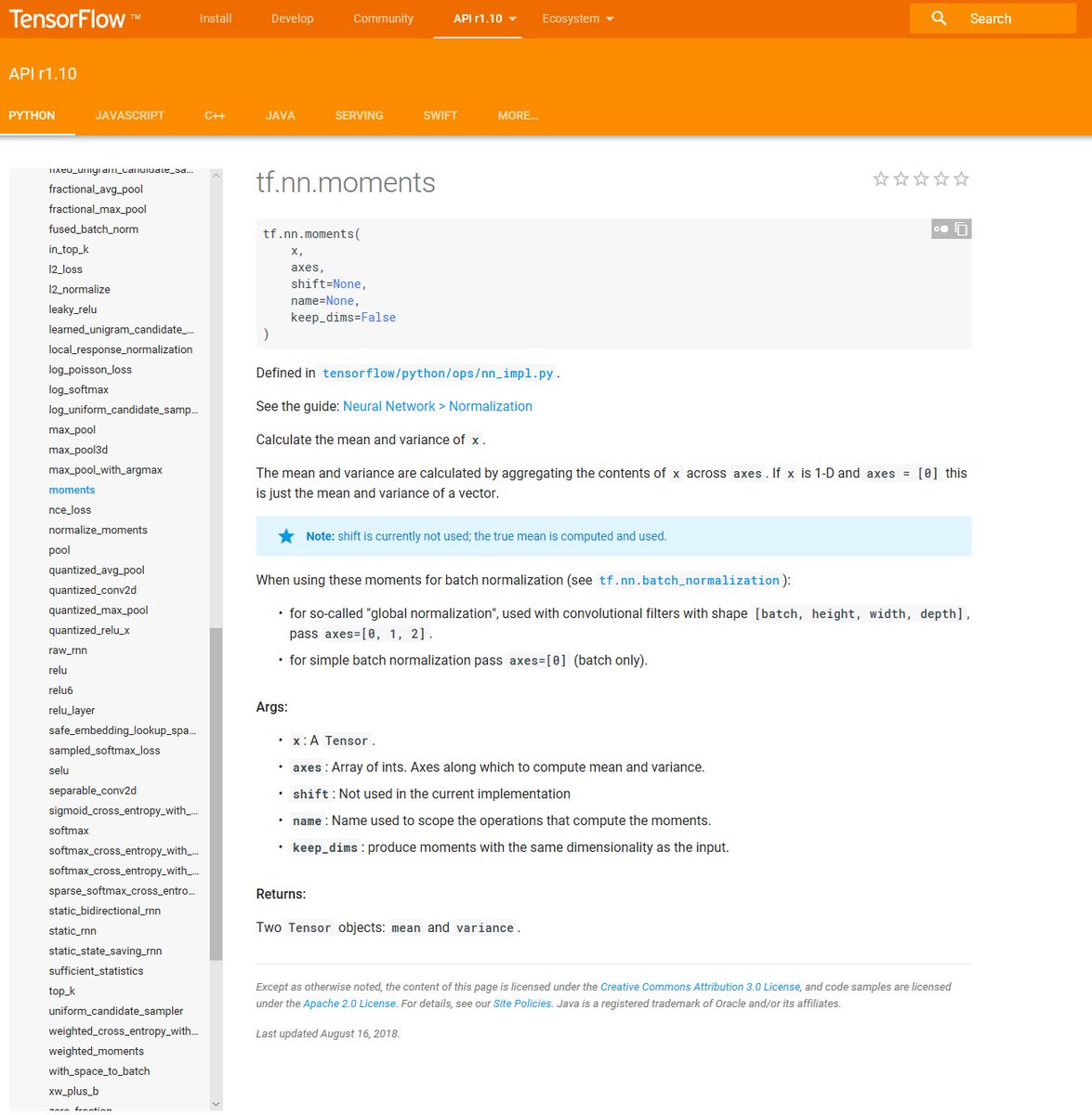
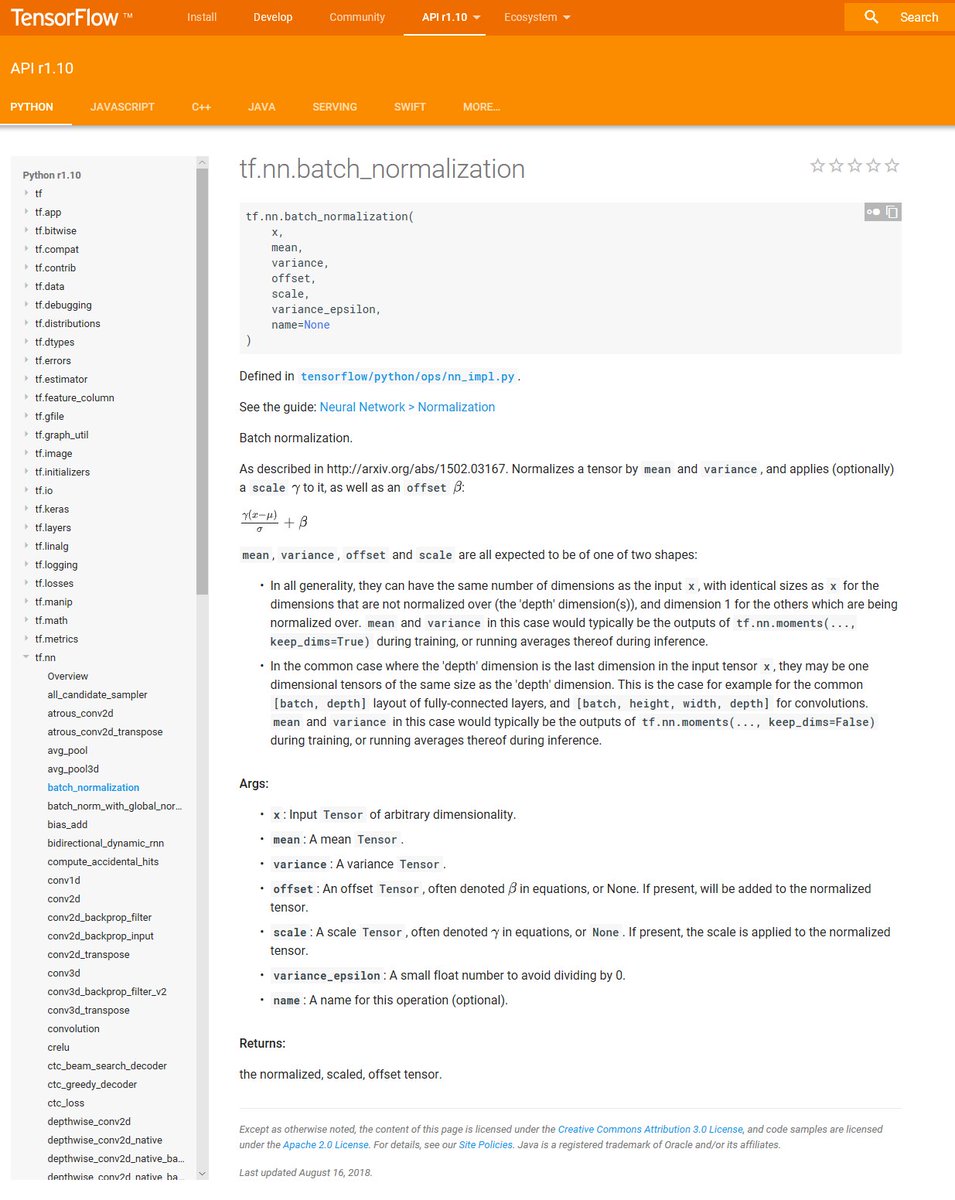
Start with a few layers; if you aren't happy with accuracy, you can always add more. Another perk? The fewer layers you start out with, the faster the execution.
Your weight initialization randomization function needs to be carefully chosen and specified; otherwise, there is a high risk that your training progress will be slow to the point of impractical.
An option, in @TensorFlow: tensorflow.org/api_docs/pytho…
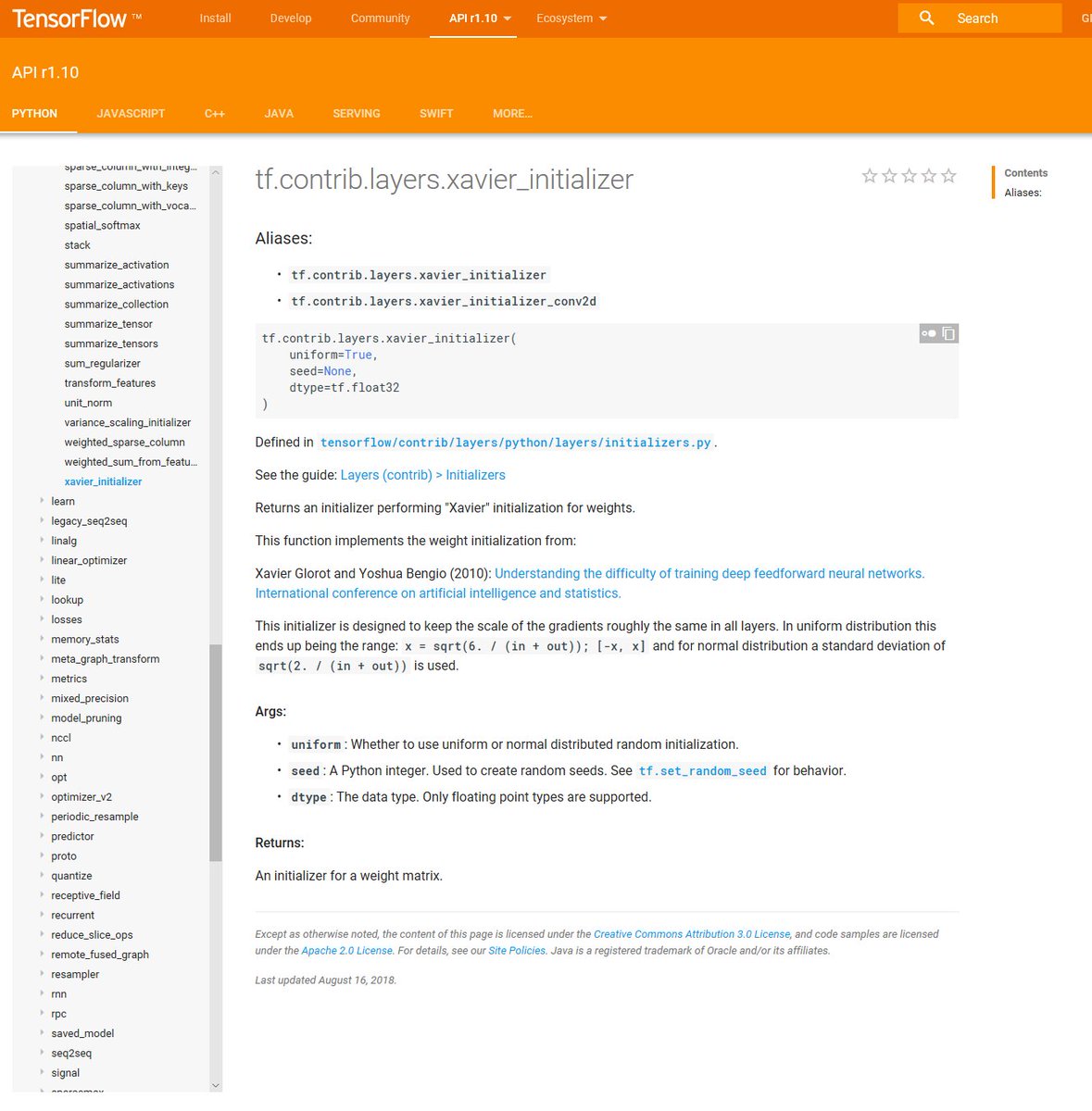
Dropout layers set a certain percentage of inputs to zero before passing the signals as output, which reduces codependency of the inputs.
In @TensorFlow, you can use something like tf.nn.dropout.
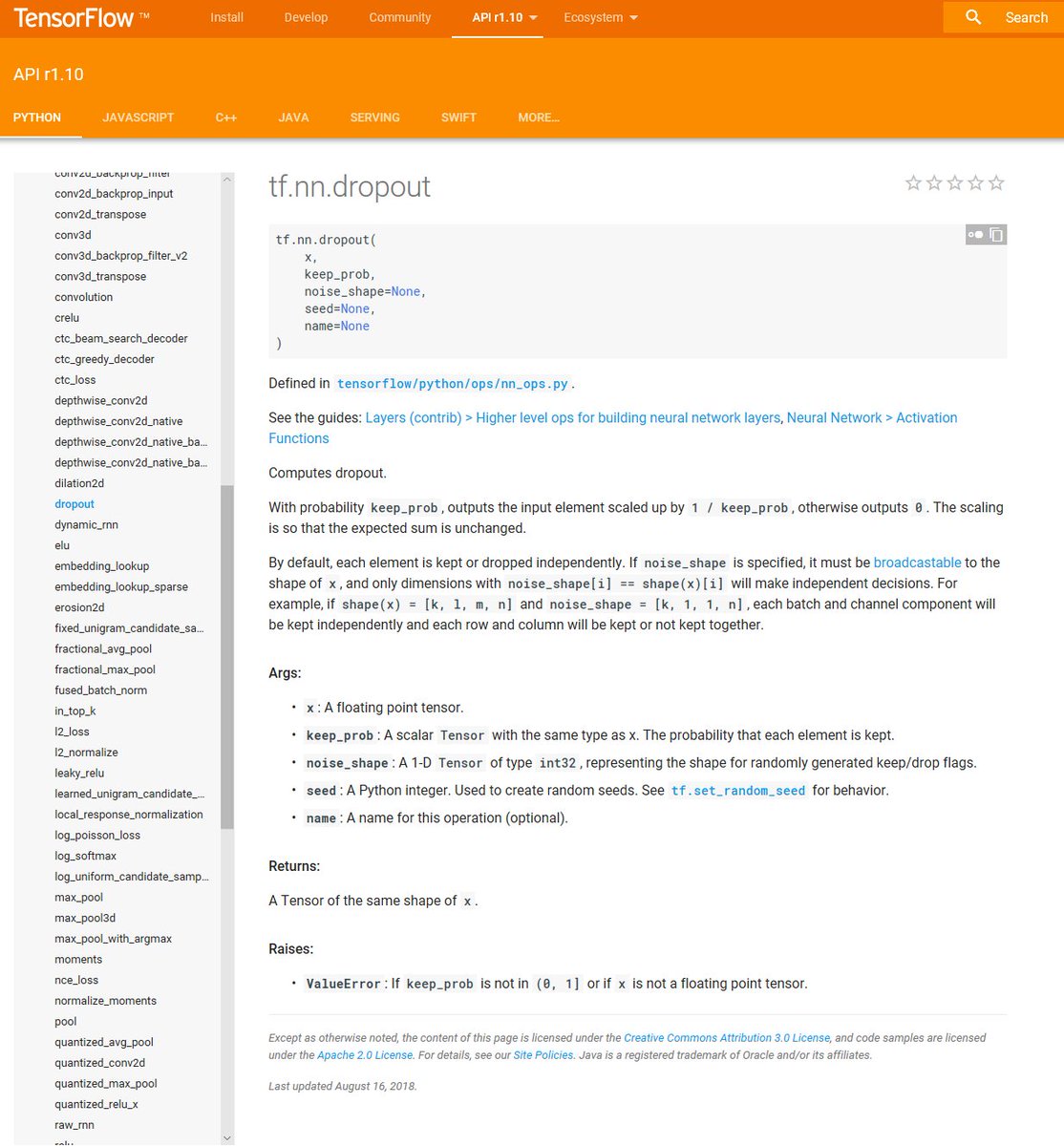
The most common aren't necessarily the best for your particular task; and researchers haven't reached consensus, either.
👍A few of the most common: GradientDescentOptimizer, AdamOptimizer, AdagradOptimizer. tensorflow.org/api_guides/pyt…
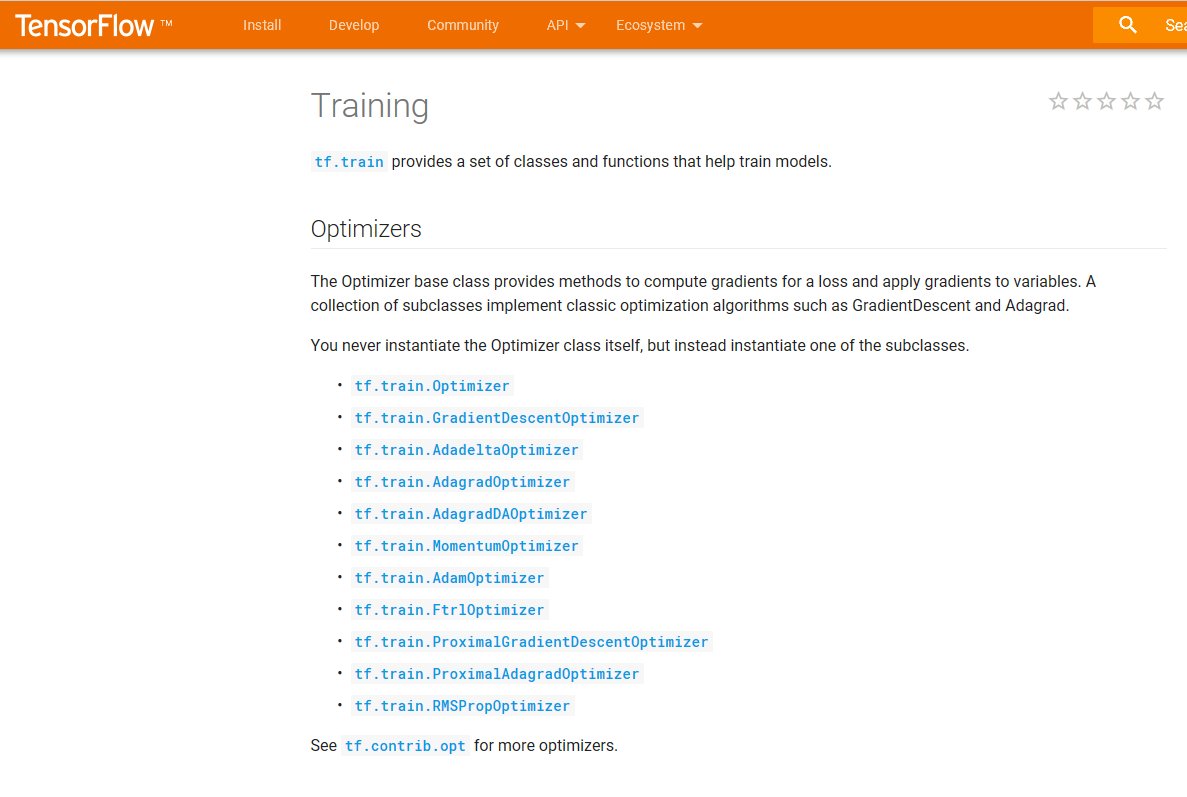
Most of the time, learning rates vary from 0.0001 to 0.5 - and most examples you see in tutorials start at the higher end. 📈
This stuff changes, every day. It's okay to feel overwhelmed. 💕
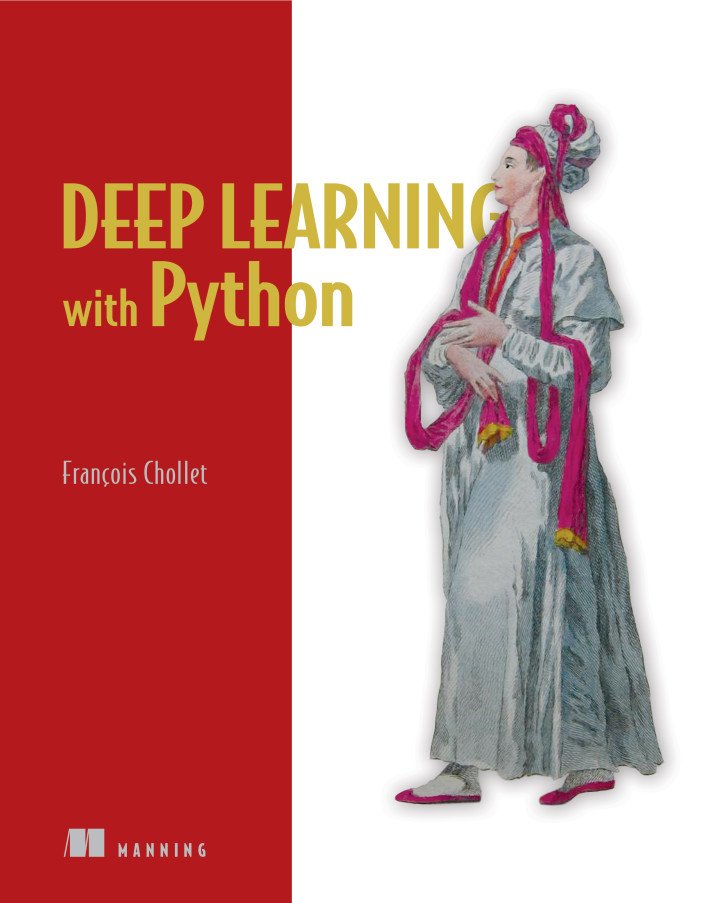
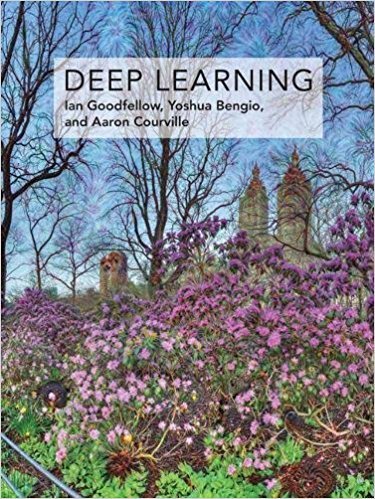
Deep learning is progressing rapidly, and new research is being published *every day*. It's 100% okay to feel overwhelmed. ❤️
(My goal is just to have you feel energized and excited about it, too.)