Today, let’s talk about e-values and how to interpret them!
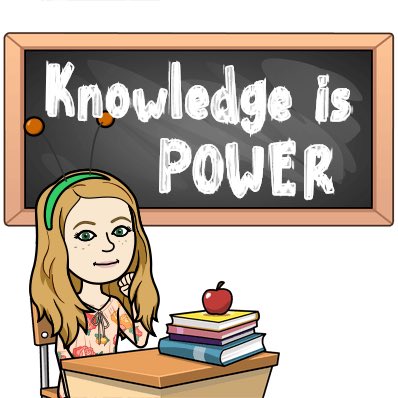
The original paper introducing the e-value is here: annals.org/aim/article-ab…
academic.oup.com/aje/article/18…
Say we are interested in the effect of diet on health or on the effect of air pollution on health (the 2 types of studies assessed in the paper by @l_trinquart & colleagues).
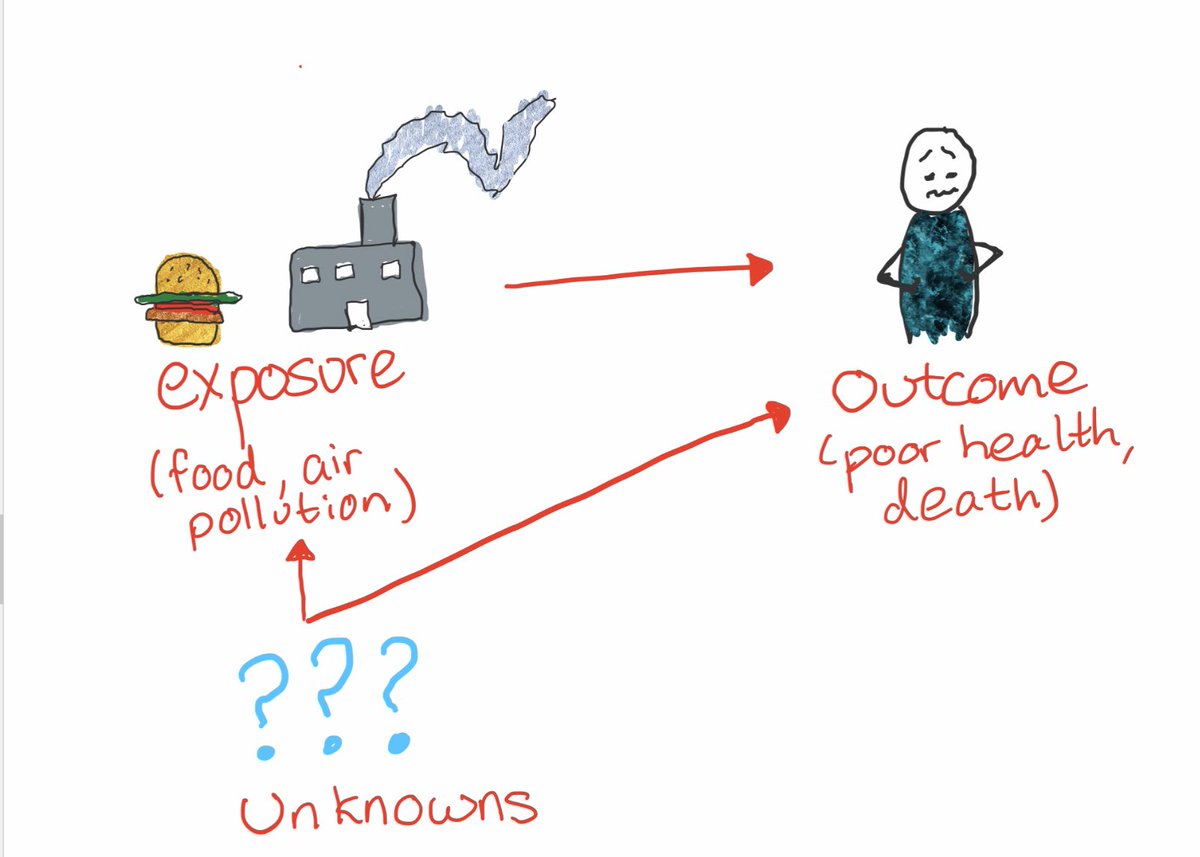
But the paper by @l_trinquart & colleagues is a good example of why that’s not always true!
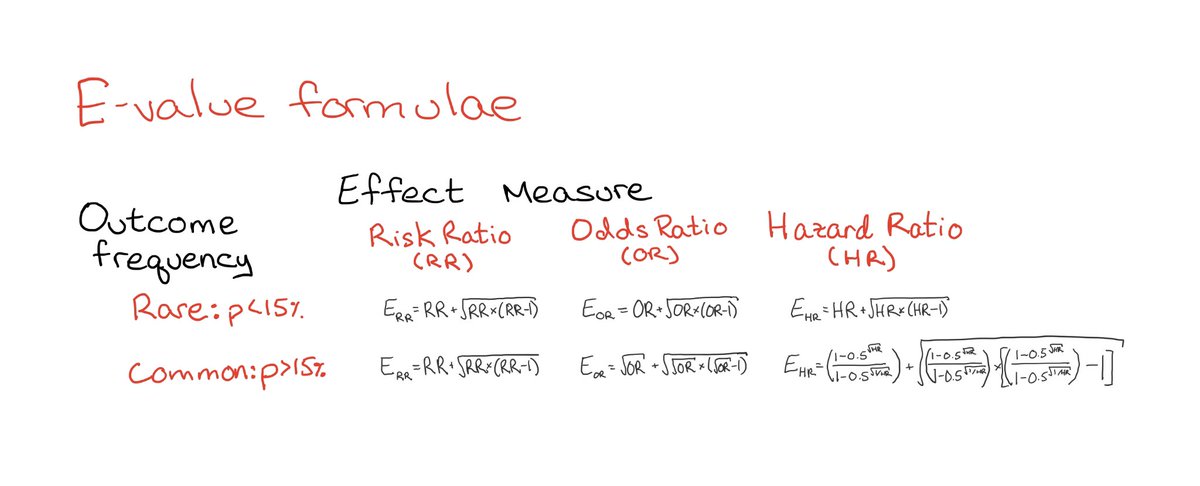
This makes sense—a bigger estimate *does seem* harder to explain by unknowns.
(ps do you like my custom gif?👇🏼-@epiellie)
(Also FYI, descendants of a collider operate with the same DAG rules as colliders!👇🏼p-value is opened!)
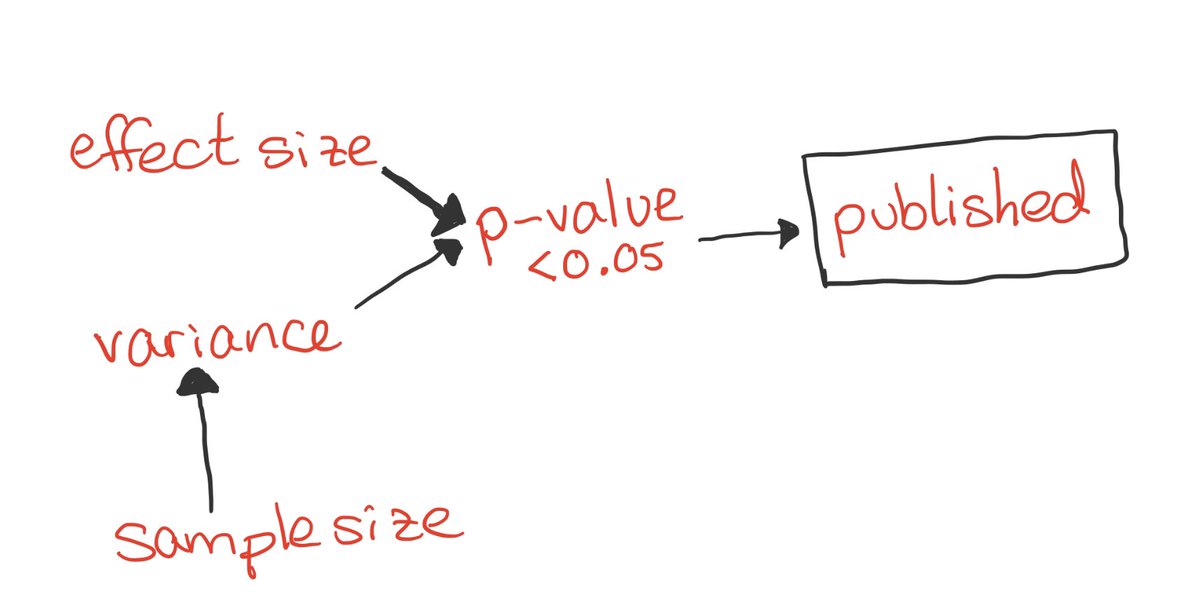
Studies of air pollution had bigger sample sizes than studies of nutrition—over 20,000 more participants on average!!
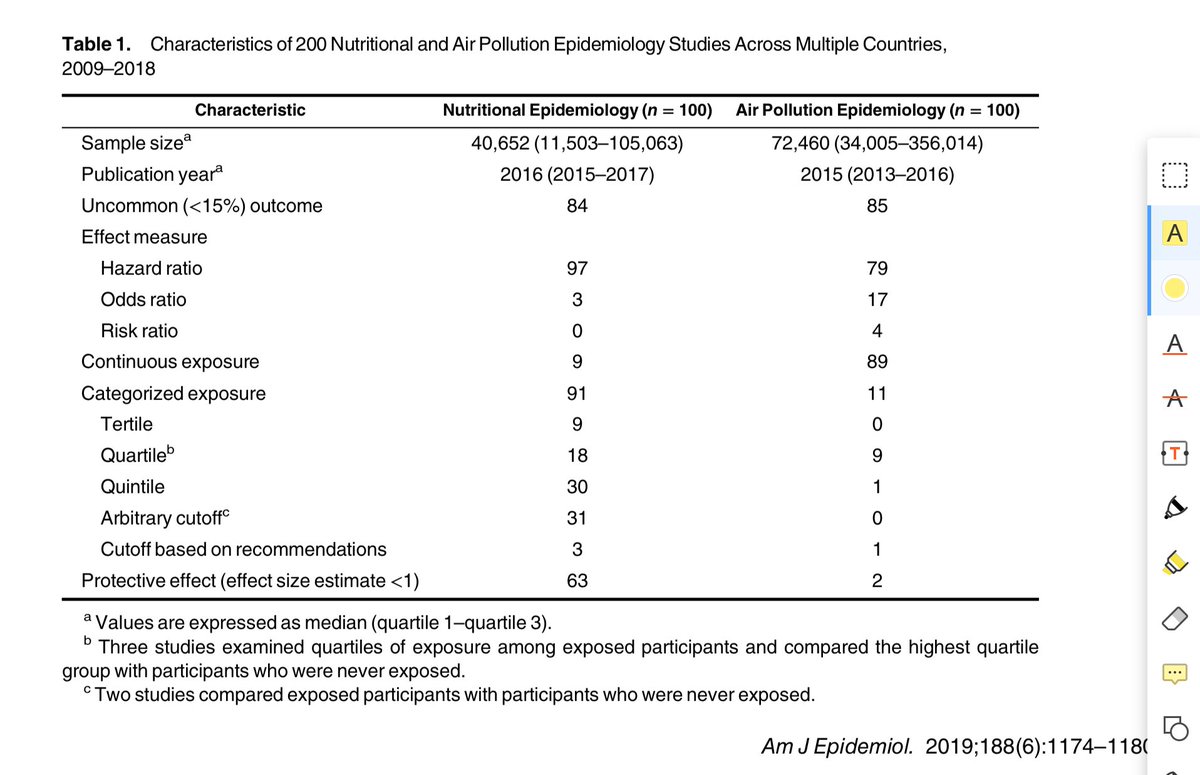
(Expand to vote in poll!)
I’ll be in the comments to help you think through it!
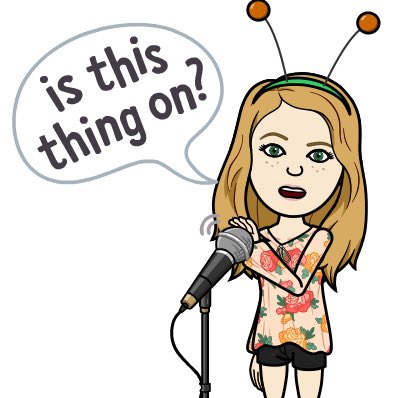