Will try to live tweet, but if I can't keep up, the good news is that @rctatman is sitting right next to me, and I'm sure she'll be on it :)
Notes major changes in the last 6 years: cheap computing power, changes in algorithms, and vast pipeline of data.
How? We are training contemporary AI systems to see through the lens of our own histories.
But: "Science may have cured biased AI" headline... *sigh*
14c: Oblique line
16c: Unfair treatment
20c: Technical meaning in statistics ... ported into ML
But we have to work across disciplines if we're going to solve these problems.
Allocation is getting all the attention because it's immediate. Representation is longterm, affecting attitudes & beliefs.
Allocation also much easier to quantify.
Word embeddings aren't just for analogies. Also used across lots of apps, like MT.
Being able to understand that problem requires understanding history & culture. Need people in the room who can call it out and say this has to change.
Need to think more deeply about what is being optimized for in the ML set up.
Need a different theoretical tool kit.
1. Classification is always a product of its time
2. We're currently living through the biggest experiment on classification in human history
[EMB: I wish we could get away from framing Aristotle as "the" beginning.]
Most common person in it: George W. Bush
b/c it was made by scraping Yahoo! news images from 2002.
Implications of choice of using e.g. news photography...
Classificatory harm.
And there's currently start ups making money on this (?!) e.g. Faceception.
Q: Final decision v. proxy decision --- intermediate steps are what causes the harm. Maybe we can go directly from data to decision?
A: Really -- can you think of any cases where we go from data to decision without someone designing how that's done?
A: How do we do that?
Q: By getting enough data.
A: Regulation?
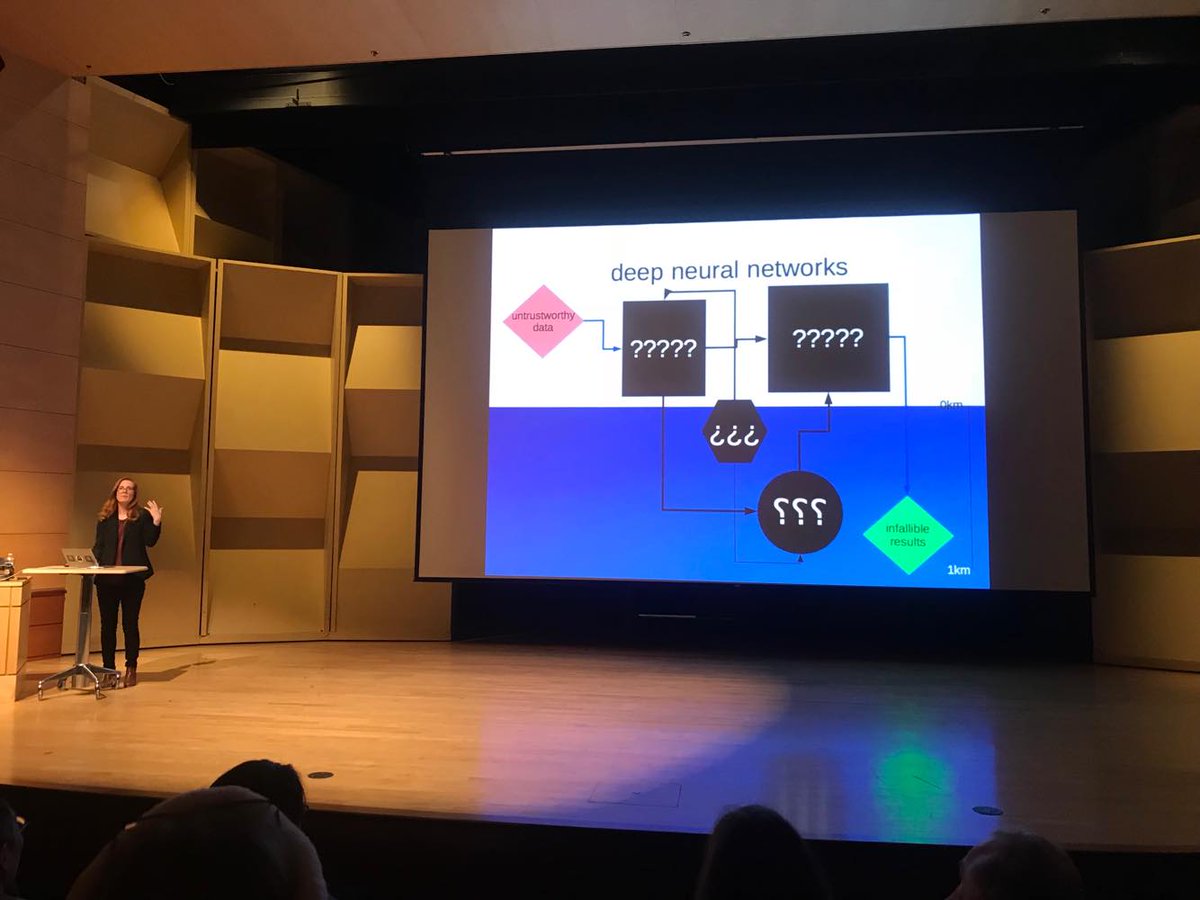