My theory: Cambridge Analytica was matching users with political messages using the exact same technique Netflix uses to recommend you movies.
On the other hand, if my guess is correct, CA’s targeting was likely state of the art. I expect it worked exceedingly well.
sifter.org/simon/journal/…
It remains pretty much state-of-the-art for predicting what users will like.
motherboard.vice.com/en_us/article/…
If so, what would that mean?
Then use the 100 most powerful factors to predict users’ age, race, partisanship, or even personality.
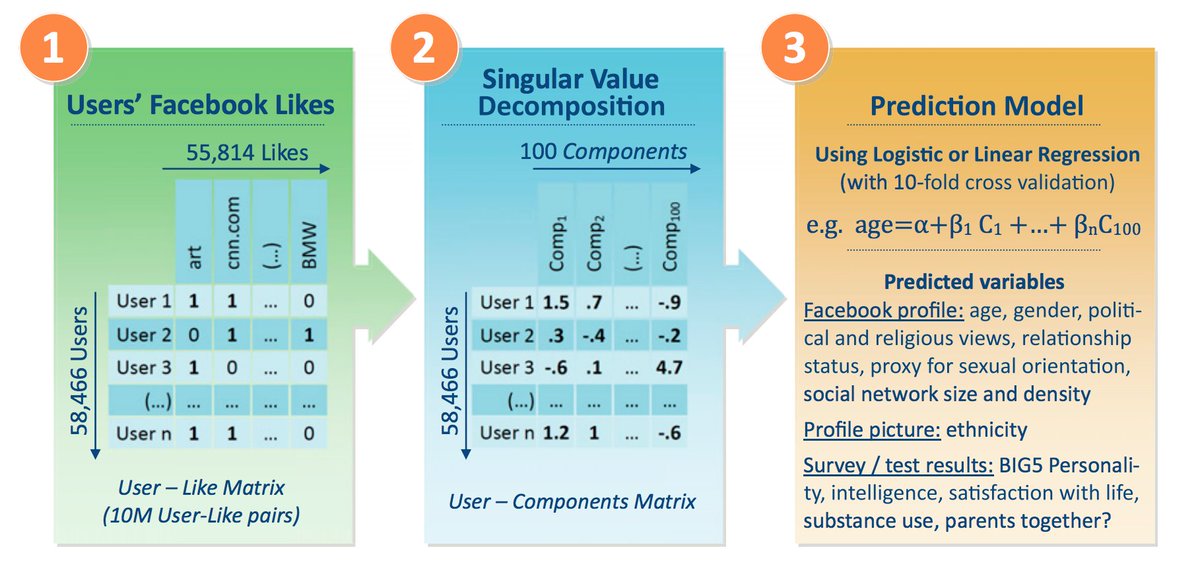
But you can also use it to predict age. Or race. Or partisanship. Or almost anything correlated with Facebook behavior.
These are very different sorts of movies, but it’s tough to give them a clear label.
But the Big Five models, which had *already* been roughly mapped to the Facebook data, provide a solution.
But the *same exact model* could, just as accurately, be sold as targeting (say) low-education older Republican men.
Even if all of the above are true, it’s really *constant*, iterative testing to map messages to these factors that makes this work.