prisma-statement.org
SPOILER ALERT: Very few do. The ones that do *typically* include a checklist in the supplement
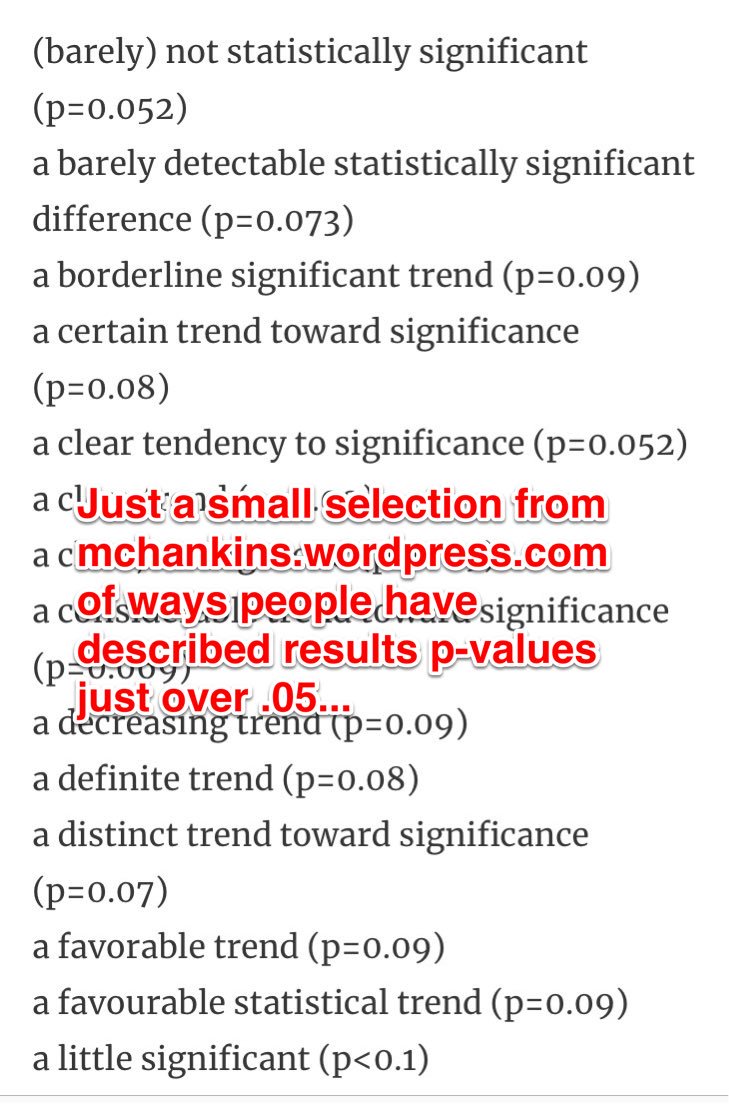
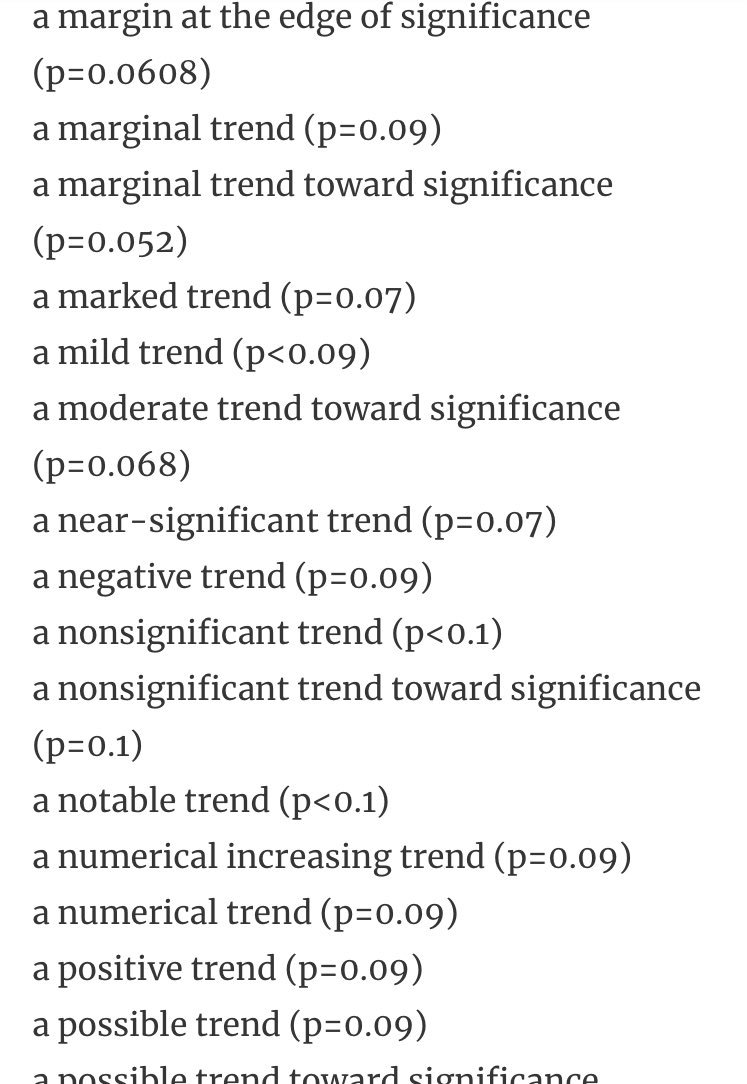
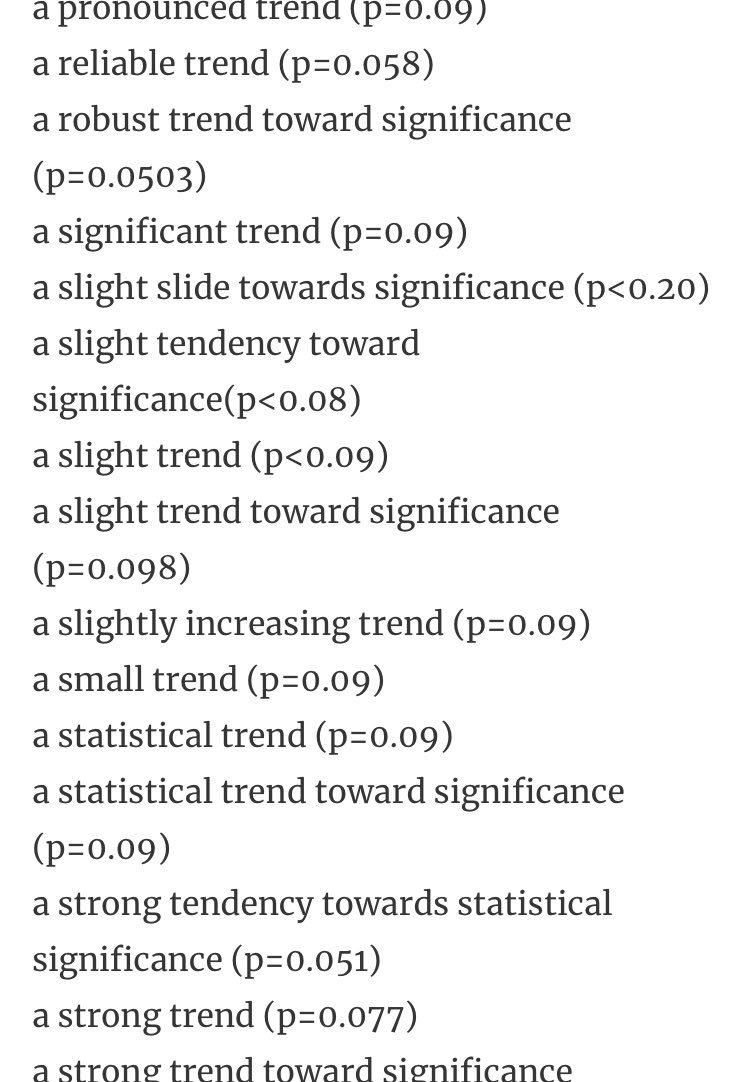
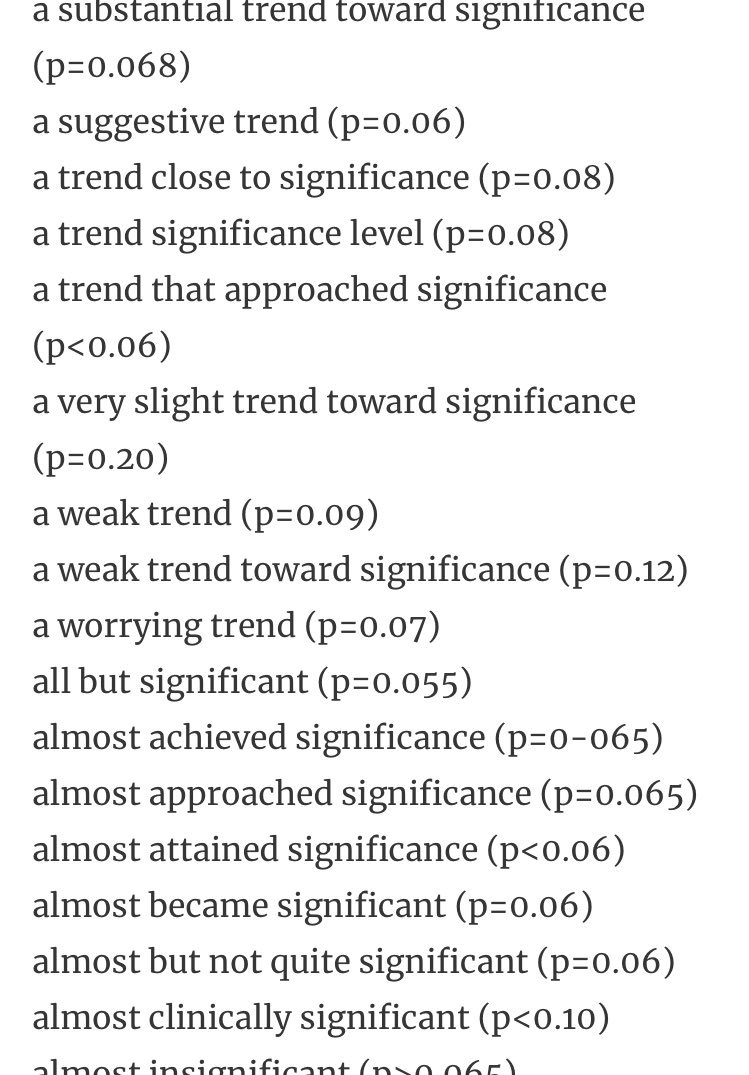
Better: Peer-reviewed protocol (more detail required but few journals offer this)
Best: Registered report MA (even greater detail needed but even fewer journal options, for now)
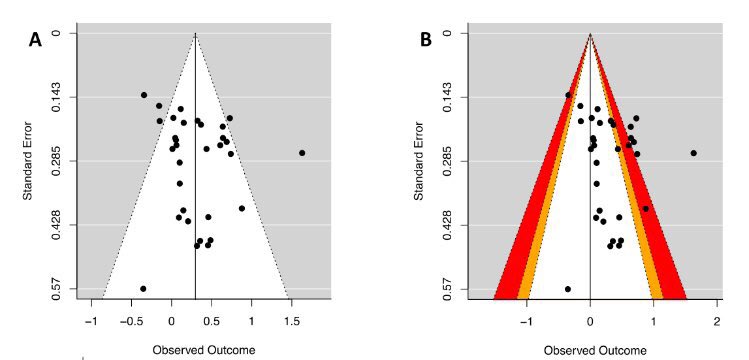
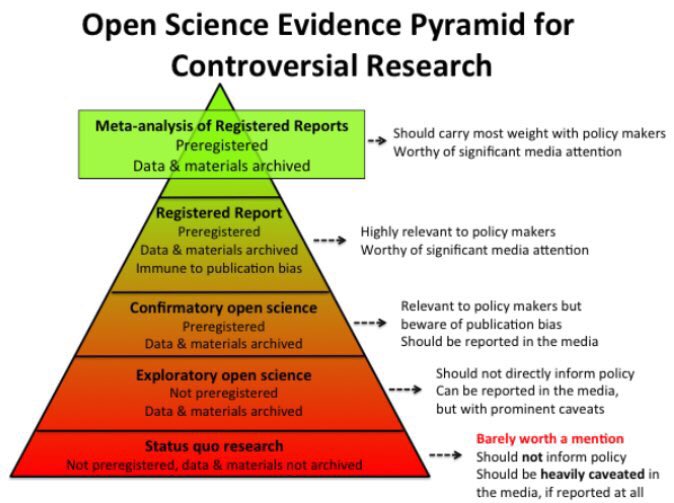
All things equal, trust the meta-analysis with open data & scripts
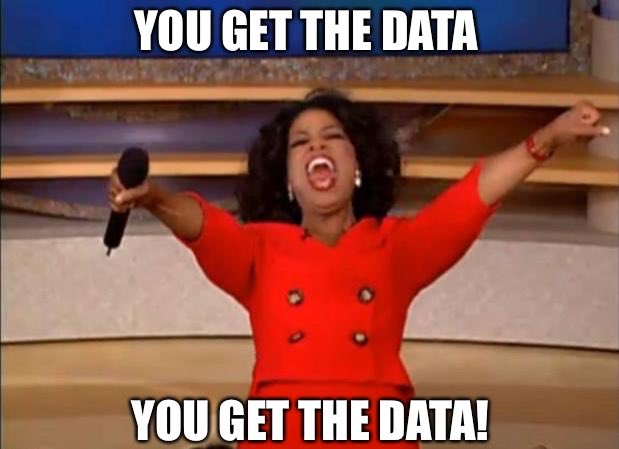
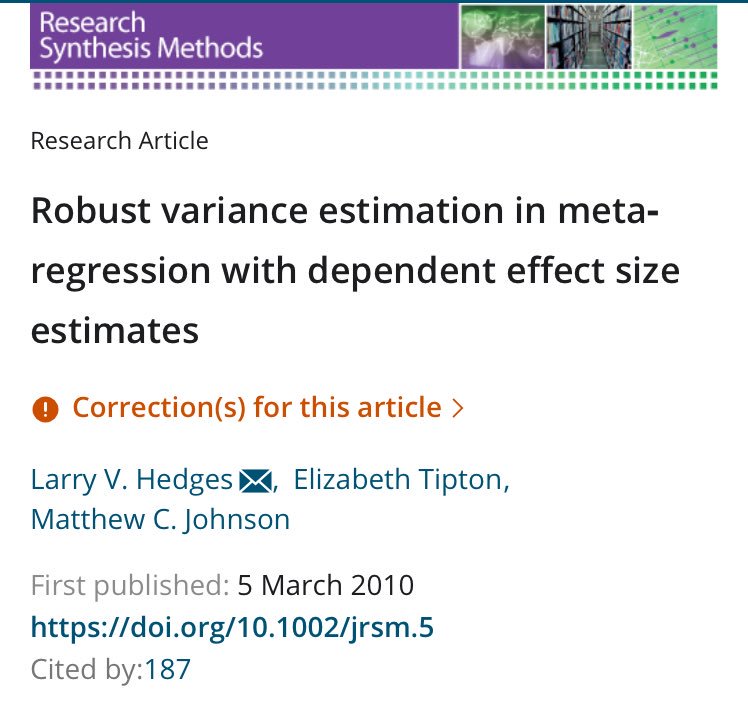